Least square method
우리는 error를 최소화 해야 할 필요가 있다! 그래서 가장 첫번째로 등장하는 방법이 LSE, Least Square Method이다.
Q=Q(β0,β1)=i∑(Yi−β0−β1Xi)2
Least square는 위와 같이 나타낼 수 있다. 즉 Yi에서 E(Y)를 제해준 값으로, random error term을 의미하기도 한다.
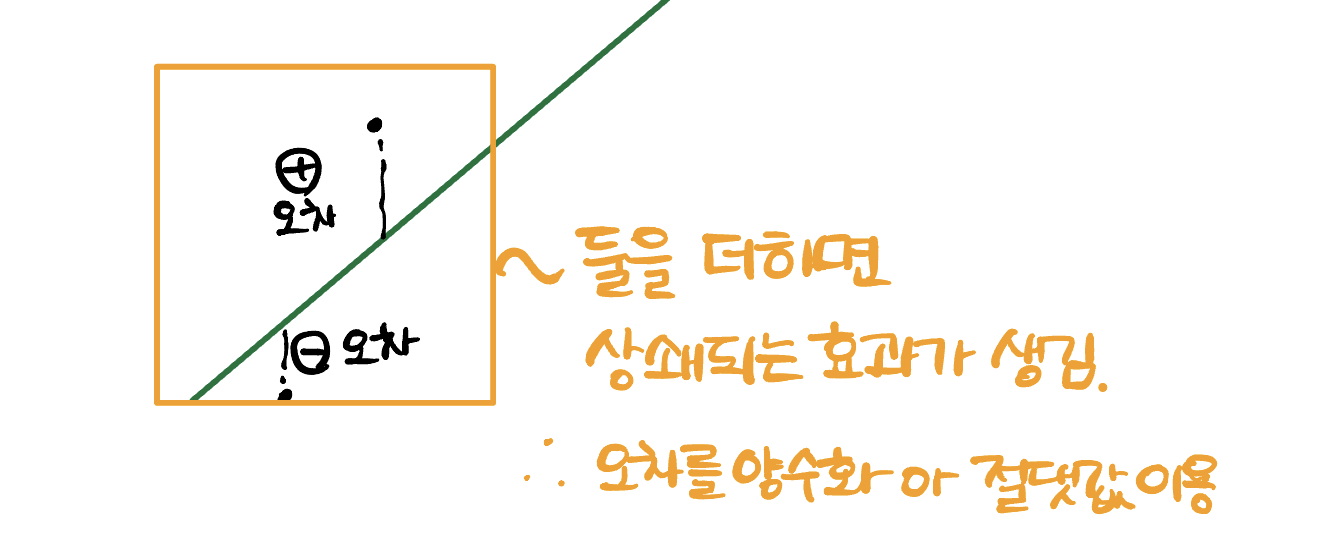
그리고 그냥 값이 아닌 제곱을 하는 이유는 음수의 오차와 양수의 오차를 더했을 때 값이 상쇄되는 효과가 일어난다. 또한 절댓값을 사용하지 않는 이유는 최솟값을 구할 때 미분을 사용하지 못하기 때문이다.
Q. 그렇다면 최적의 β0,β1 값은?
A. 편미분하면 된다!
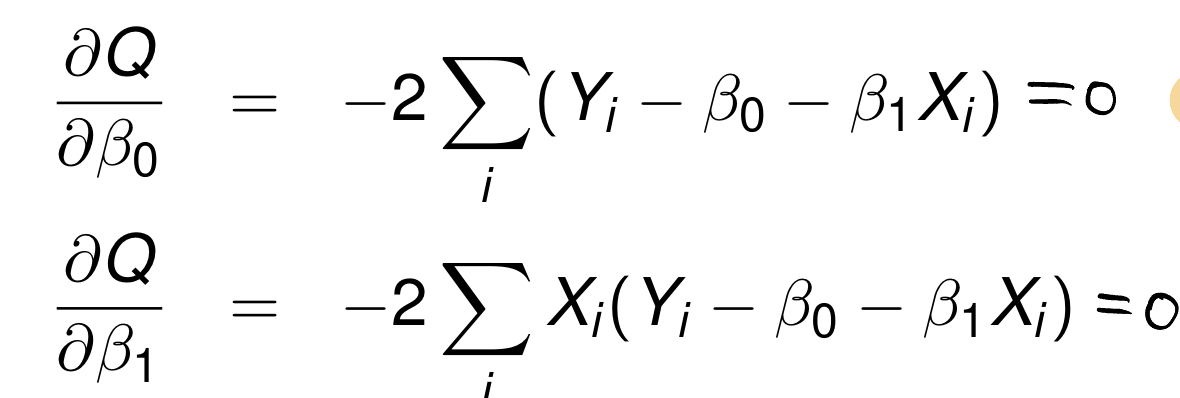
위와 같은 식을 우리는 정규방정식, normal equation 이라고 한다.
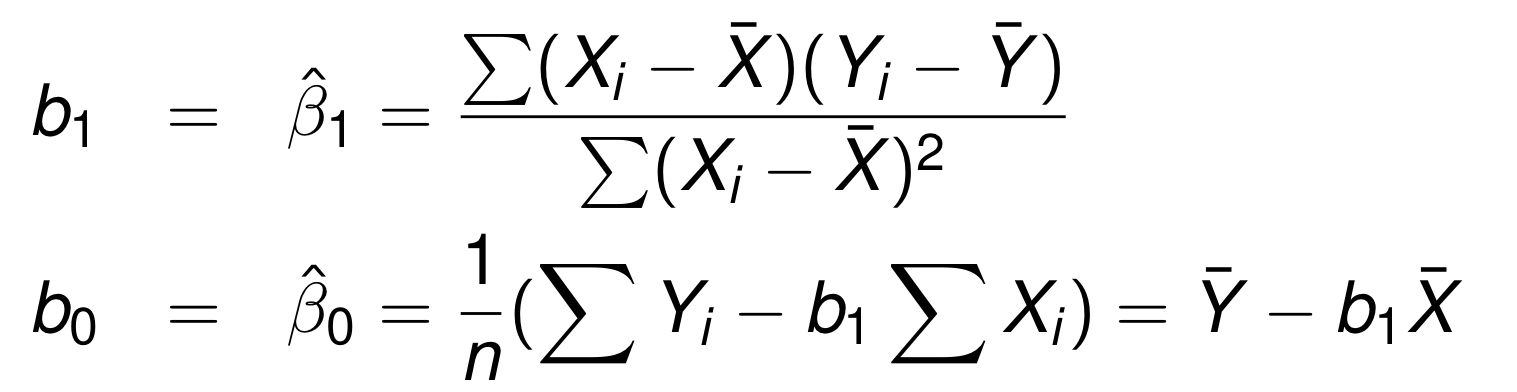
정규방정식을 풀면 다음과 같이 계수를 추정할 수 있다.
Gauss Markov Theorem
그렇다면 least square method로 얻은 것이 합리적인지, good approach인지 알아야 한다. 이 부분은 수업시간에 증명하지 않았지만 나중에 한번 읽어보기 위해 위키 링크를 미리 첨부해둔다.
https://en.wikipedia.org/wiki/Gauss%E2%80%93Markov_theorem
그리고 이 theorem에 따르면 우리가 LSE로 추정한 계수가 BLUE임을 알 수 있다. (best linear unbiased estimatiors)
Residual
그렇다면 이제 구분이 필요하다.
E(Y)=β0+β1X
Y^=b0+b1X
Y^는 regression을 통해 추정된 회귀계수를 이용해 나타낸 식이다. 이제 이것을 fitted 라고 칭할 것이다.
Residual VS Random error
residual 은 실제 값에서 추정 값을 제외한 것이고, error term과는 엄연히 다름을 확실히 해야한다. Random errorsms 실제 값에서 평균값을 제외해준 값이다. 식으로 표현하면 다음과 같다.
Residual : ei=Yi.−Yi^ (실제값 - 추정값)
Random error : ϵi=Yi−E(Yi) (실제값 - 평균값)
properties of fitted regression line
- residual의 합은 0이다.
- squared residual의 합은 최솟값이다.
- Yi의 합과 Yi^의 합은 동일하다.
- 추정된 regression line은 항상 (Xˉ,Yˉ)를 지나간다.
용어 정리
ei=Yi−Yi^ 는 residual
SSE = ∑i(Yi−Y^)2=∑i(ϵi)2
MSE : mean squared error, SSE를 degree free (자유도)로 나누어준 값이다. linear regression에서는 자유도가 n-2이다. 2는 추정해야하는 회귀계수를 제외해준 것이다.
s2=MSE=SSE/(n−2)
E(MSE)=σ2
(여기서 s2은 unbiased estimator)
Normal Error Regression model
Yi=β0+β1Xi+ϵi
위에서 같이 error가 정규분포를 따른다고 가정하자.
즉
E(ϵi)=0
Var(ϵi)=σ2
Cov(ϵi,ϵj)=0
이다.
to be continued..