VGGNET 소개
- VGGNET은 19 Layer, GoogleNet은 22 Layer으로써 Layer 개수가 늘었다.
- CNN 망이 깊고 넓을 수록 성능이 더 좋아지지만, 문제는 학습이 어려워진다.
- 또한 Parameter 개수가 늘어나는 문제, Vanishing/Exploding Gradient Problem 등이 등장하게 된다.
- CNN 기본 구조가 Convolutional 다음에 해상도를 줄이는 Pooling Layer가 오는데. VGGNet은 그러지 않고 Convolutional Layer을 추가적으로 더 쌓았다. 이에 따른 효과는 Parameter 수가 줄어들게 되고, 학습 속도가 빨라짐. 또한 Non-linearity가 증가하게 됨.
- 224 x 224 Color image를 받고 -> [여러 개의 Convolutional Layer -> Maxpooling] 의 반복 -> 맨 마지막에 Fully Connect Layer 3개가 옴.
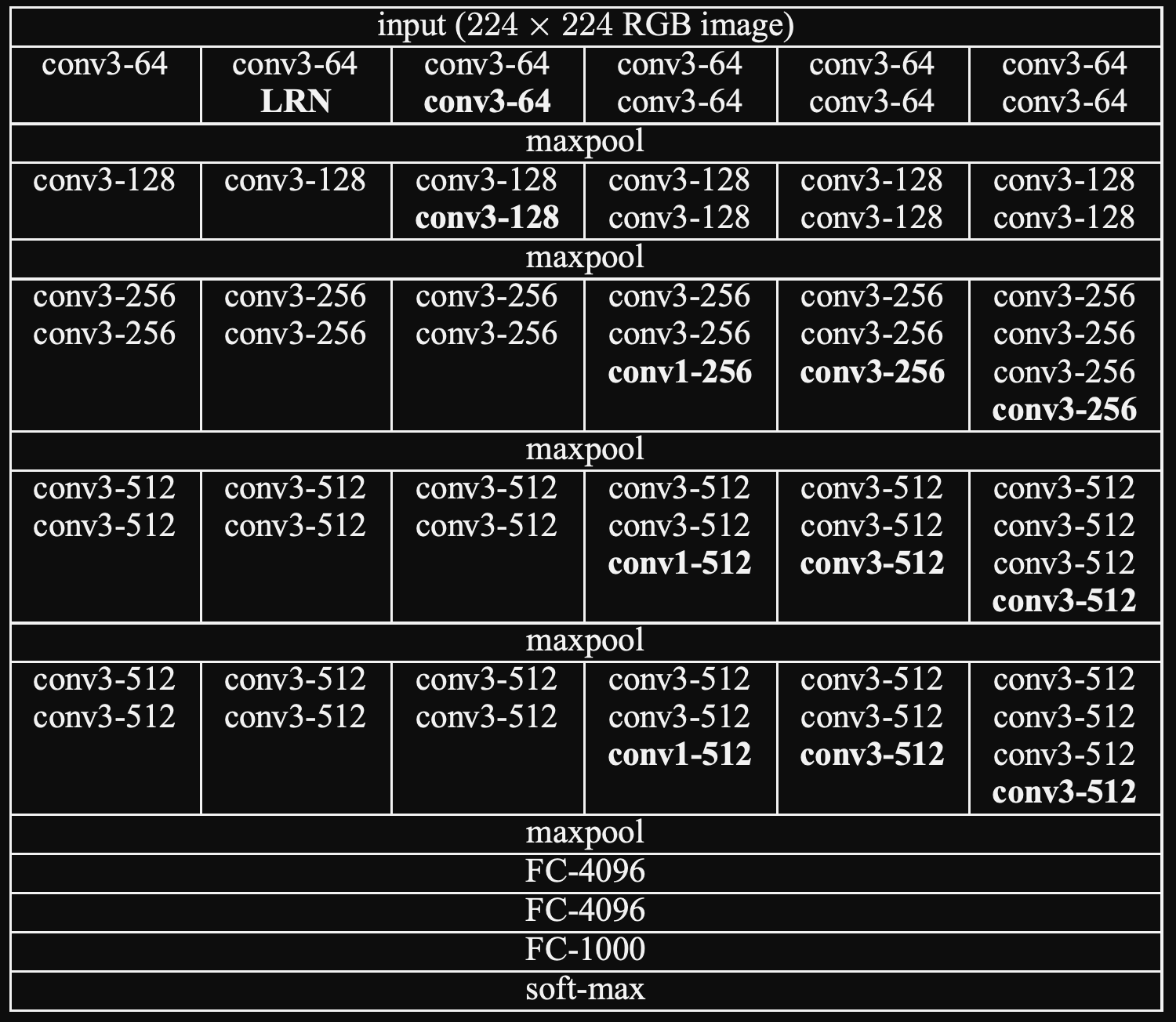
- 각 Hidden Layer에는 ReLU가 쓰임.
- Tensor Factorization: VGGNet에서 Convolutional Layer을 중첩해서 쌓을 때 일어나는 현상. Parameter가 절감되는 것을 보여줌. 5 x 5 Layer 가 아니라 이를 3 x 3 9개로 쪼개고, 그 다음에 3 x 3 하나, 마지막에 1 x 1 을 거치게 된다면. 25 parameter이 아니라 9 + 9 = 18 개의 Parameter가 된다는 것.
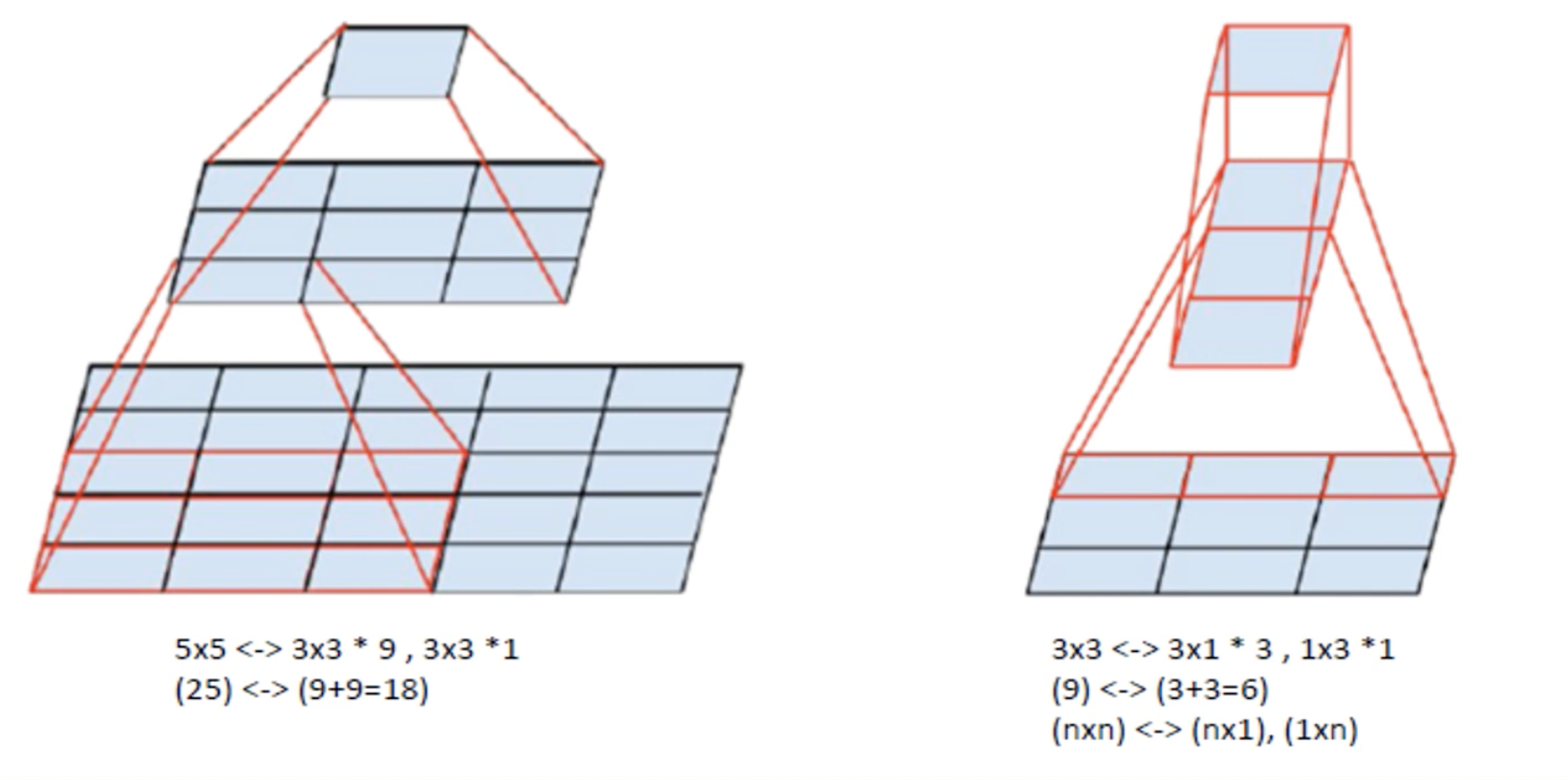
- Mulri-crop, dense를 동시한 수행이 기능이 제일 좋았다.
torchvision VGGNet Code
class VGG(nn.Module):
def __init__(
self,
features: nn.Module,
num_classes: int = 1000,
init_weights: bool = True
) -> None:
super(VGG, self).__init__()
self.features = features
self.avgpool = nn.AdaptiveAvgPool2d((7, 7))
self.classifier = nn.Sequential(
nn.Linear(512 * 7 * 7, 4096),
nn.ReLU(True),
nn.Dropout(),
nn.Linear(4096, 4096),
nn.ReLU(True),
nn.Dropout(),
nn.Linear(4096, num_classes),
)
if init_weights:
self._initialize_weights()
def forward(self, x: torch.Tensor) -> torch.Tensor:
x = self.features(x)
x = self.avgpool(x)
x = torch.flatten(x, 1)
x = self.classifier(x)
return x
def _initialize_weights(self) -> None:
for m in self.modules():
if isinstance(m, nn.Conv2d):
nn.init.kaiming_normal_(m.weight, mode='fan_out', nonlinearity='relu')
if m.bias is not None:
nn.init.constant_(m.bias, 0)
elif isinstance(m, nn.BatchNorm2d):
nn.init.constant_(m.weight, 1)
nn.init.constant_(m.bias, 0)
elif isinstance(m, nn.Linear):
nn.init.normal_(m.weight, 0, 0.01)
nn.init.constant_(m.bias, 0)
def make_layers(cfg: List[Union[str, int]], batch_norm: bool = False) -> nn.Sequential:
""" Making Multiple Convolutional Layers before pooling, according to configurations """
layers: List[nn.Module] = []
in_channels = 3
for v in cfg:
if v == 'M':
layers += [nn.MaxPool2d(kernel_size=2, stride=2)]
else:
v = cast(int, v)
conv2d = nn.Conv2d(in_channels, v, kernel_size=3, padding=1)
if batch_norm:
layers += [conv2d, nn.BatchNorm2d(v), nn.ReLU(inplace=True)]
else:
layers += [conv2d, nn.ReLU(inplace=True)]
in_channels = v
return nn.Sequential(*layers)
cfgs: Dict[str, List[Union[str, int]]] = {
'A': [64, 'M', 128, 'M', 256, 256, 'M', 512, 512, 'M', 512, 512, 'M'],
'B': [64, 64, 'M', 128, 128, 'M', 256, 256, 'M', 512, 512, 'M', 512, 512, 'M'],
'D': [64, 64, 'M', 128, 128, 'M', 256, 256, 256, 'M', 512, 512, 512, 'M', 512, 512, 512, 'M'],
'E': [64, 64, 'M', 128, 128, 'M', 256, 256, 256, 256, 'M', 512, 512, 512, 512, 'M', 512, 512, 512, 512, 'M'],
}
def _vgg(arch: str, cfg: str, batch_norm: bool, pretrained: bool, progress: bool, **kwargs: Any) -> VGG:
if pretrained:
kwargs['init_weights'] = False
model = VGG(make_layers(cfgs[cfg], batch_norm=batch_norm), **kwargs)
if pretrained:
state_dict = load_state_dict_from_url(model_urls[arch],
progress=progress)
model.load_state_dict(state_dict)
return model
내용 출처