Long Sequence
Longseq introduction
- hihello/charseq과 다른점 : input문장이 길어 특정 size로 잘라서 사용함. 조각조각 데이터를 만드는 것이다.
Ex) 한칸씩(character씩) 움직이면서 10칸씩 잘라냄
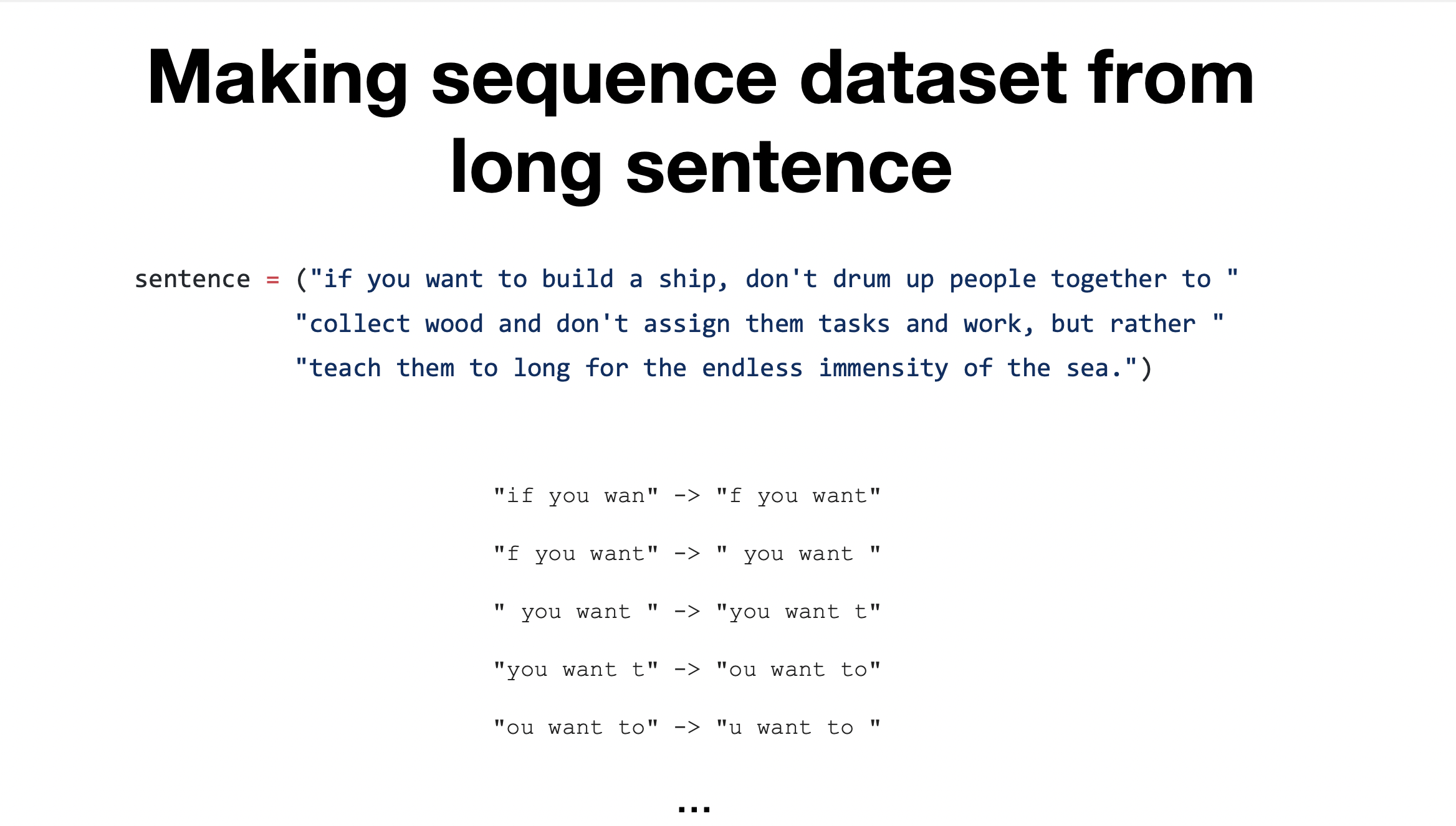
Adding FC layer and stacking RNN
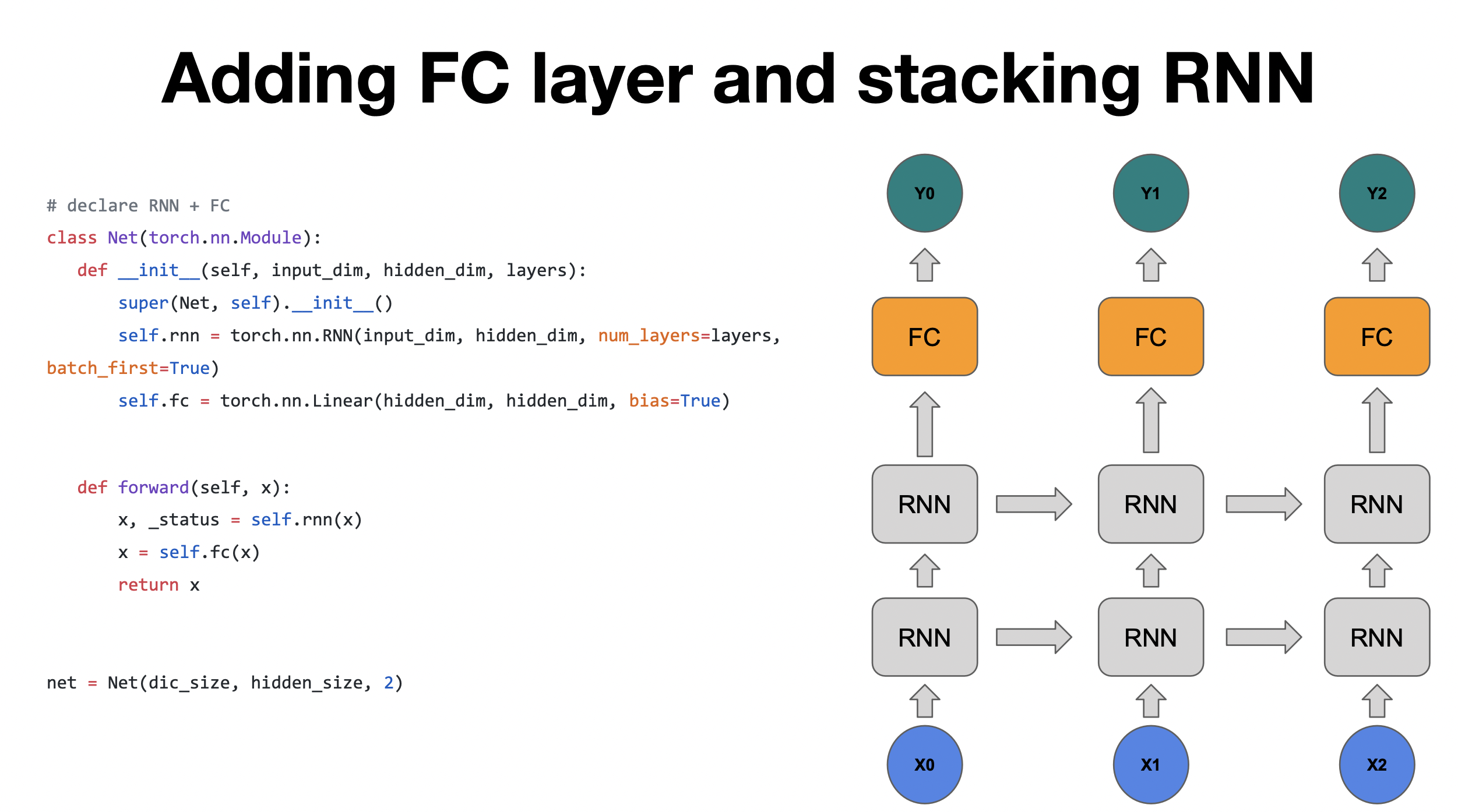
Code run through
import torch
import torch.optim as optim
import numpy as np
torch.manual_seed(0)
sentence = ("if you want to build a ship, don't drum up people together to "
"collect wood and don't assign them tasks and work, but rather "
"teach them to long for the endless immensity of the sea.")
char_set = list(set(sentence))
char_dic = {c: i for i, c in enumerate(char_set)}
dic_size = len(char_dic)
hidden_size = len(char_dic)
sequence_length = 10
learning_rate = 0.1
x_data = []
y_data = []
for i in range(0, len(sentence) - sequence_length):
x_str = sentence[i:i + sequence_length]
y_str = sentence[i + 1: i + sequence_length + 1]
print(i, x_str, '->', y_str)
x_data.append([char_dic[c] for c in x_str])
y_data.append([char_dic[c] for c in y_str])
x_one_hot = [np.eye(dic_size)[x] for x in x_data]
X = torch.FloatTensor(x_one_hot)
Y = torch.LongTensor(y_data)
class Net(torch.nn.Module):
def __init__(self, input_dim, hidden_dim, layers):
super(Net, self).__init__()
self.rnn = torch.nn.RNN(input_dim, hidden_dim, num_layers=layers, batch_first=True)
self.fc = torch.nn.Linear(hidden_dim, hidden_dim, bias=True)
def forward(self, x):
x, _status = self.rnn(x)
x = self.fc(x)
return x
class Net(torch.nn.Module):
def __init__(self, input_dim, hidden_dim, layers):
super(Net, self).__init__()
self.rnn = torch.nn.RNN(input_dim, hidden_dim, num_layers=layers, batch_first=True)
self.fc = torch.nn.Linear(hidden_dim, hidden_dim, bias=True)
def forward(self, x):
x, _status = self.rnn(x)
x = self.fc(x)
return x
net = Net(dic_size, hidden_size, 2)
criterion = torch.nn.CrossEntropyLoss()
optimizer = optim.Adam(net.parameters(), learning_rate)
for i in range(100):
optimizer.zero_grad()
outputs = net(X)
loss = criterion(outputs.view(-1, dic_size), Y.view(-1))
loss.backward()
optimizer.step()
results = outputs.argmax(dim=2)
predict_str = ""
for j, result in enumerate(results):
if j == 0:
predict_str += ''.join([char_set[t] for t in result])
else:
predict_str += char_set[result[-1]]
print(predict_str)