1. Feature Scaling
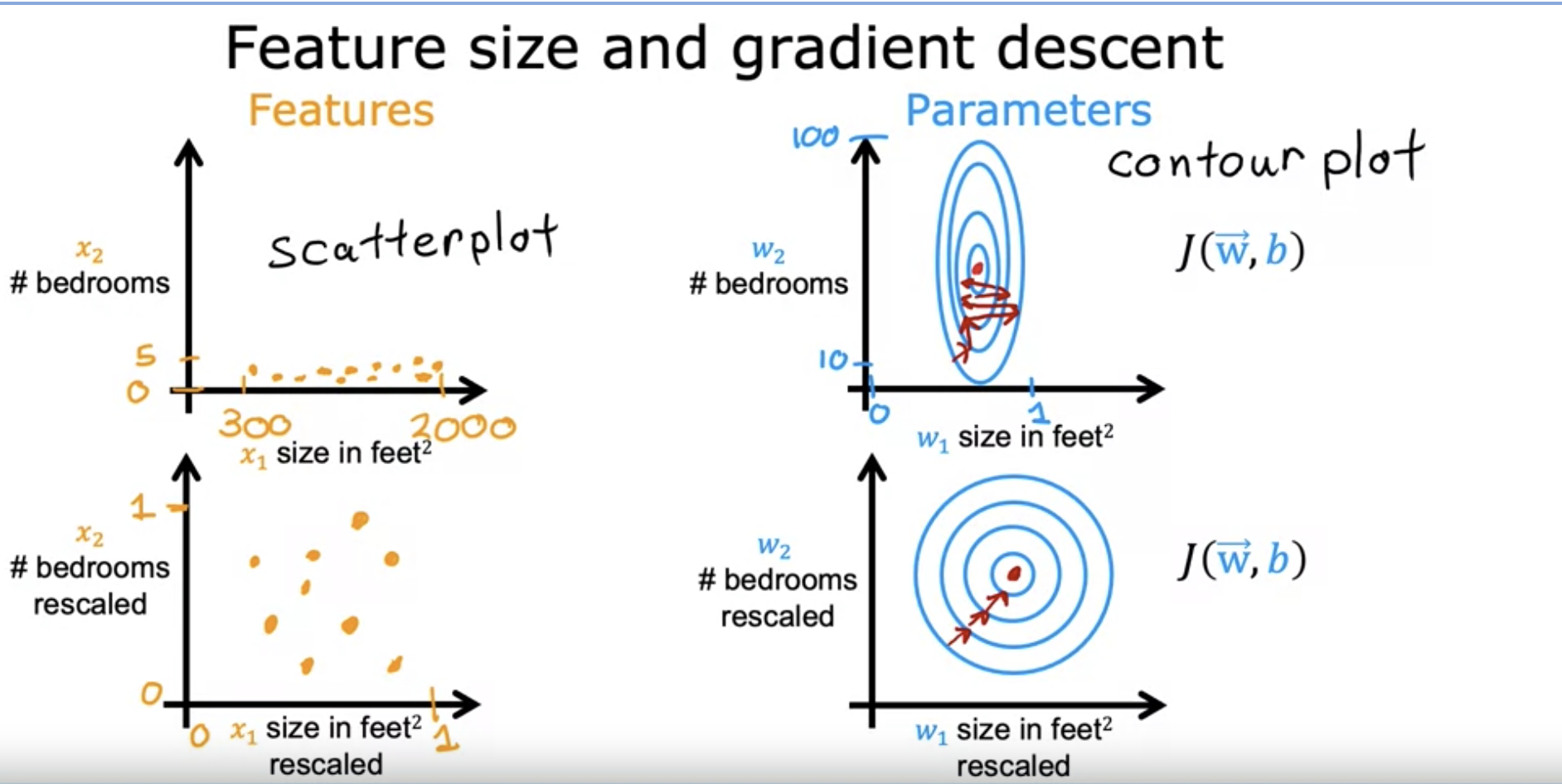
- feature scaling is about rescaling the training set values to make gradient descent easier.
2. Ways to do Feature Scaling
- Deviding the values by maximum possible value.
- Mean Normalization:
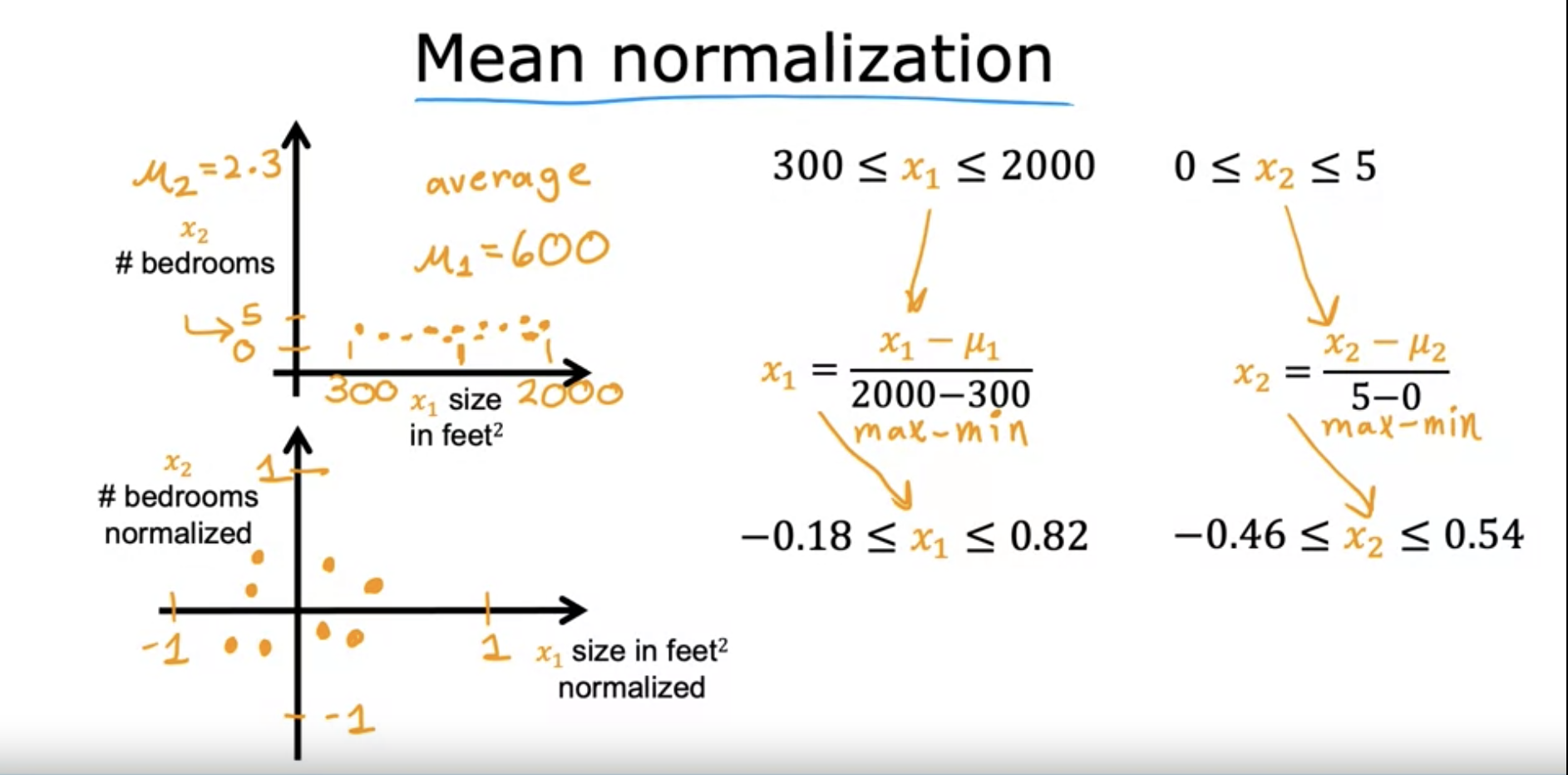
- Z-score normalization: how many standard deviation away is the value from the mean?
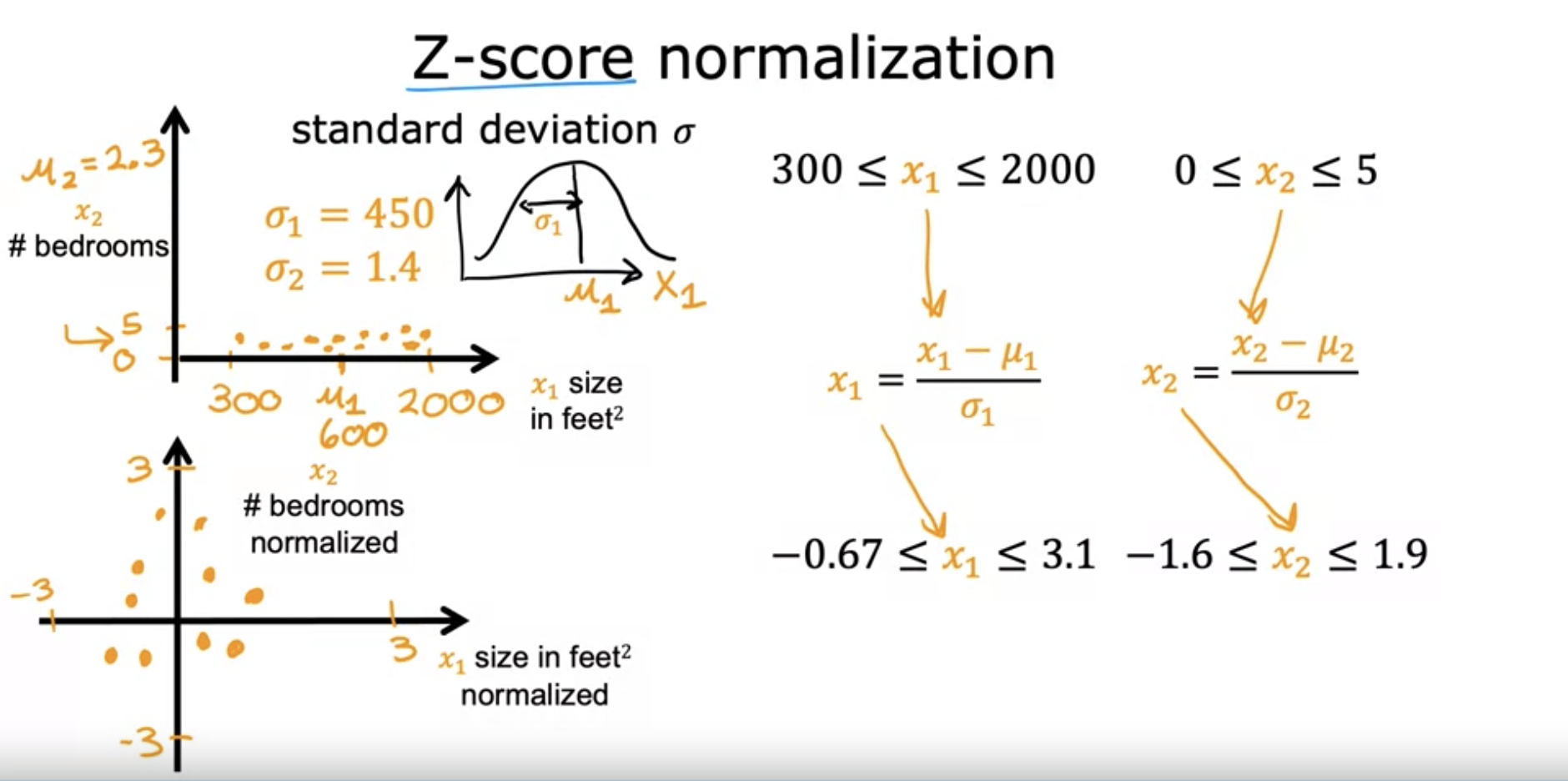
Aim for -1 <= x <= 1.
3. Choosing the Learning Rate
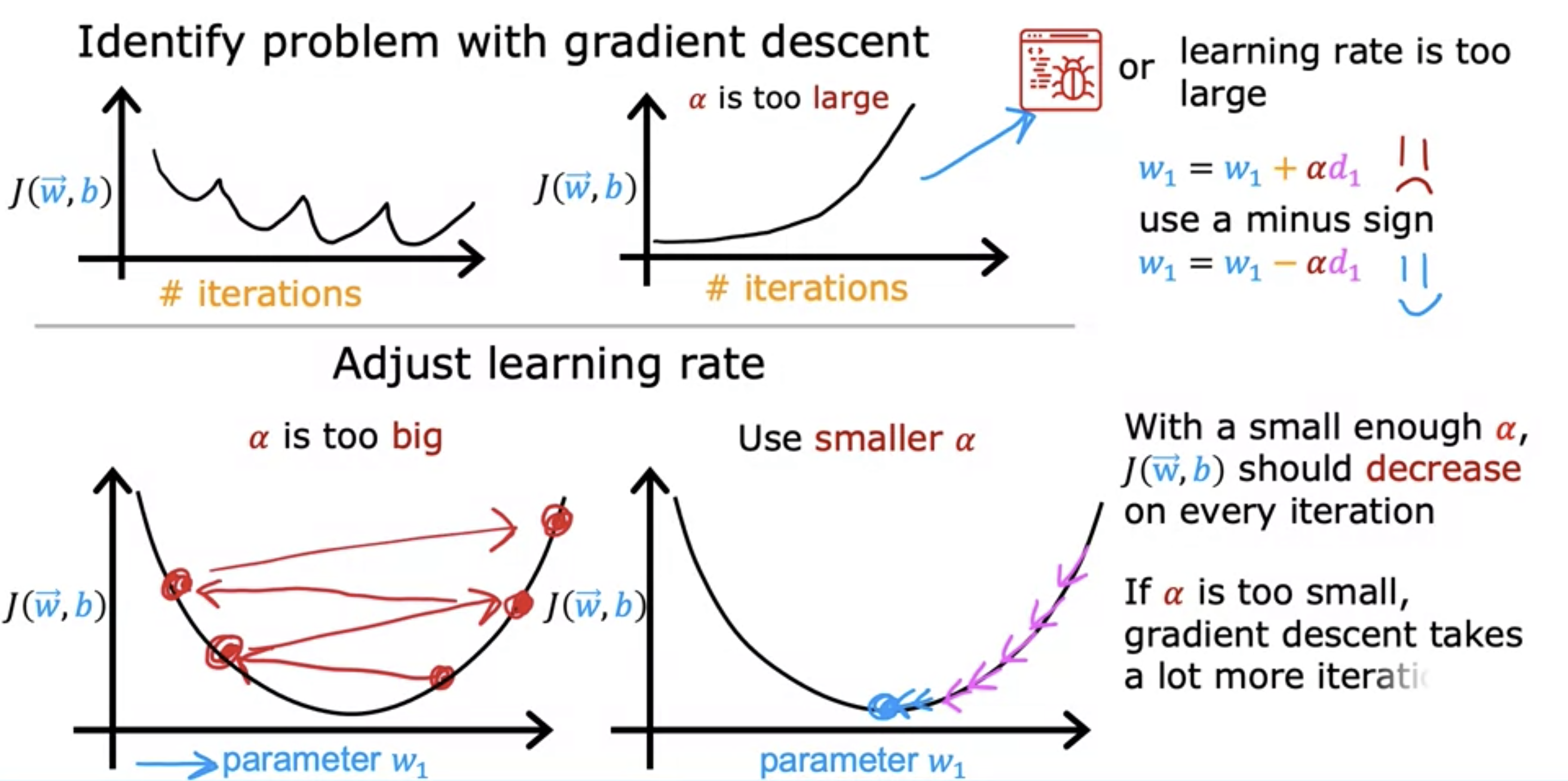
- Learning Graph is a graph of J(w,b) value by iterations.
- As iterations get larger, the cost value should decrease and converge.
- Learning rate should not be too large or small. If too large, cost may increase instead of decrease.
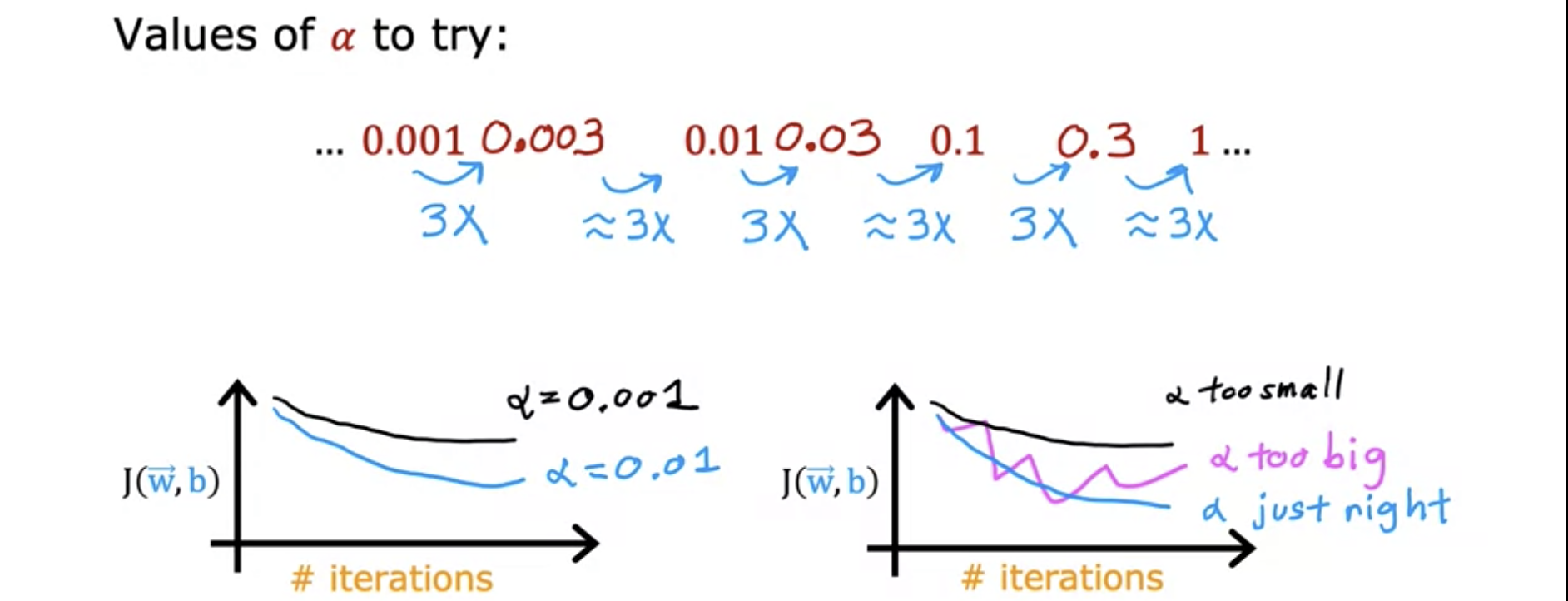
- Choose a small value and try multiplying it by 3x to look for the steepest learning curve that constantly decreases.
4. Feature Engineering
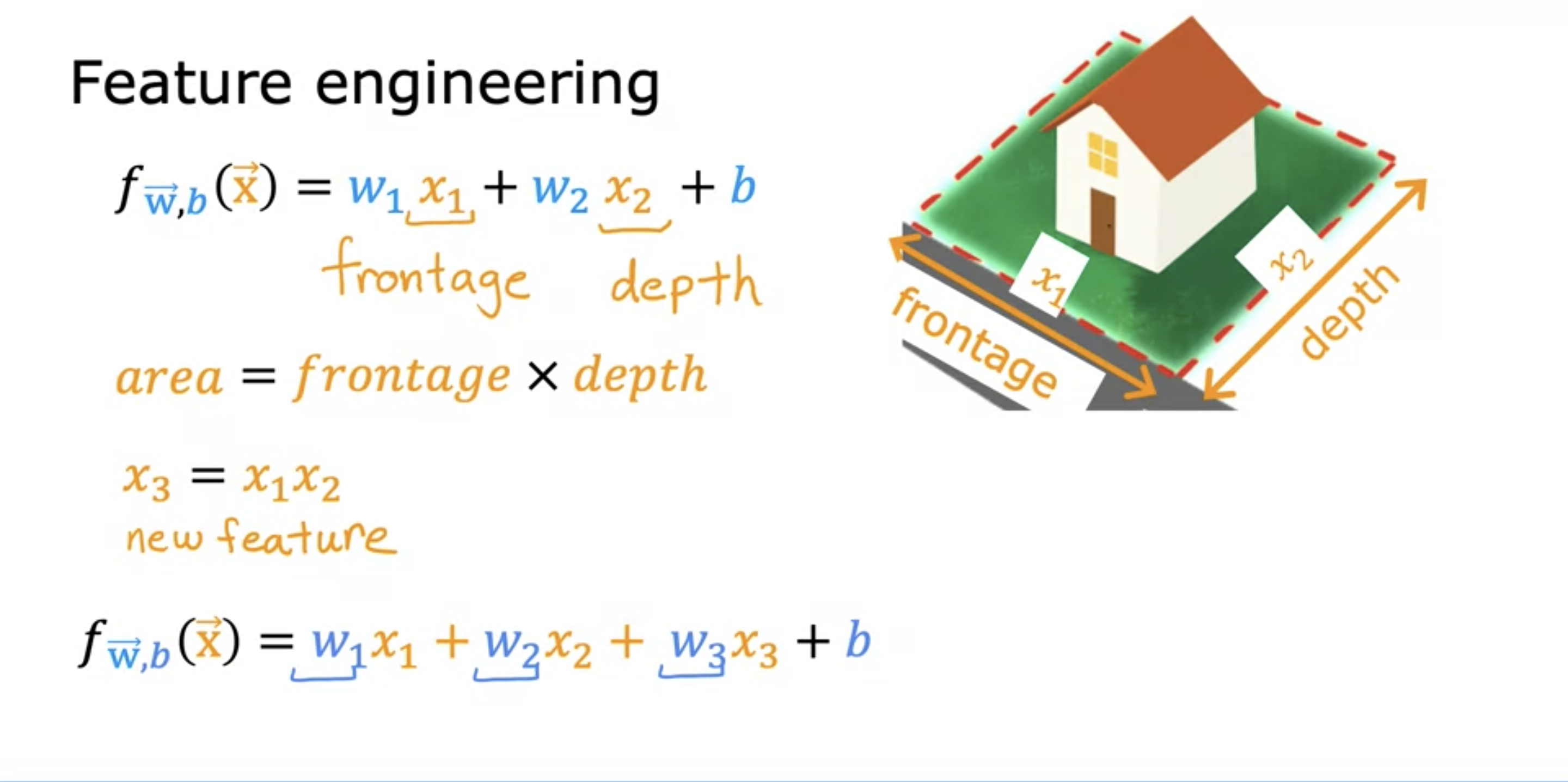
- Feature engineering involves combining or transforming the original features in the problem to get a much better model.
5. Polynomial Regression
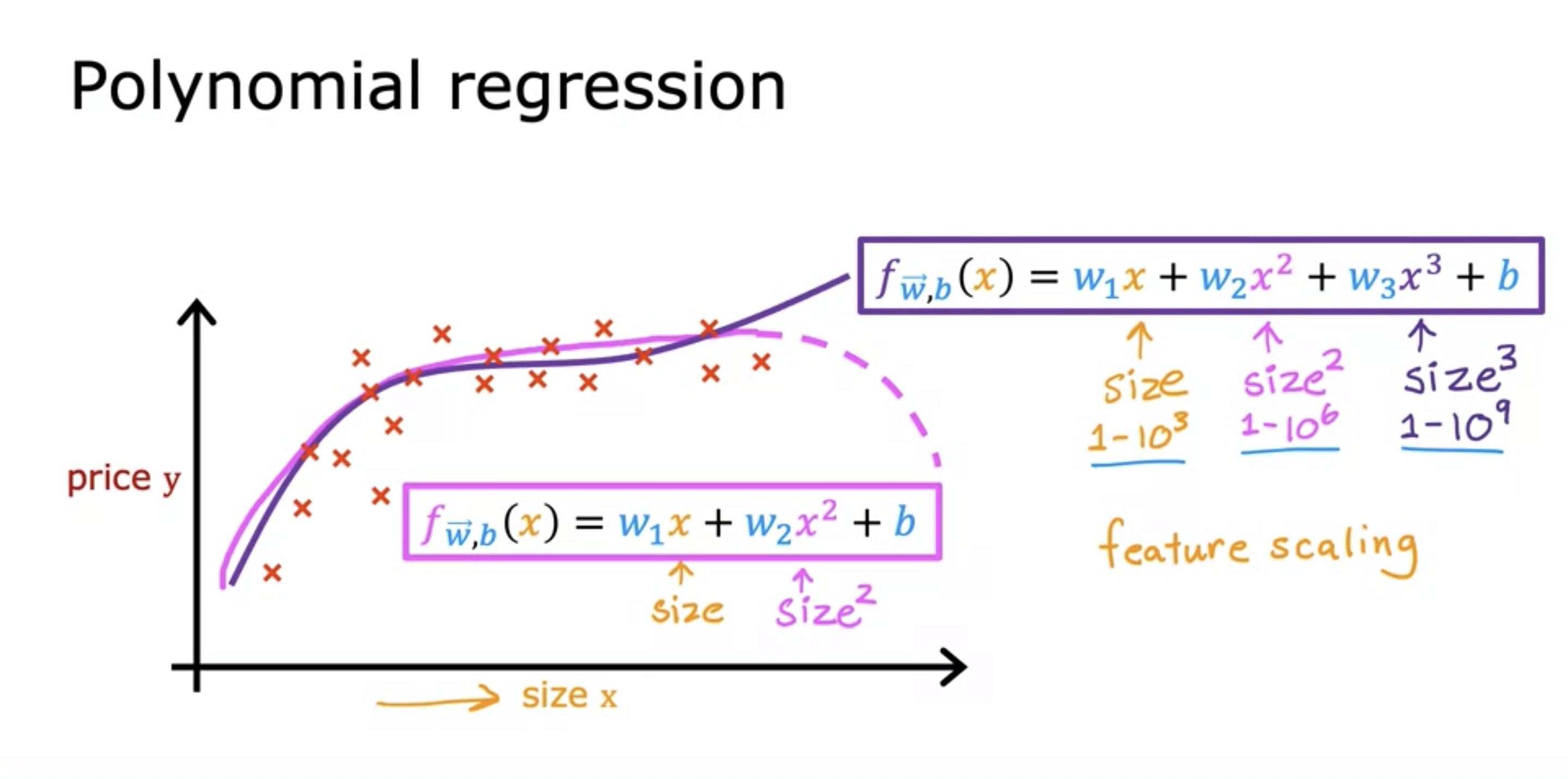
- Feature scaling is important for cubic model, because the number range would get very different for each features.
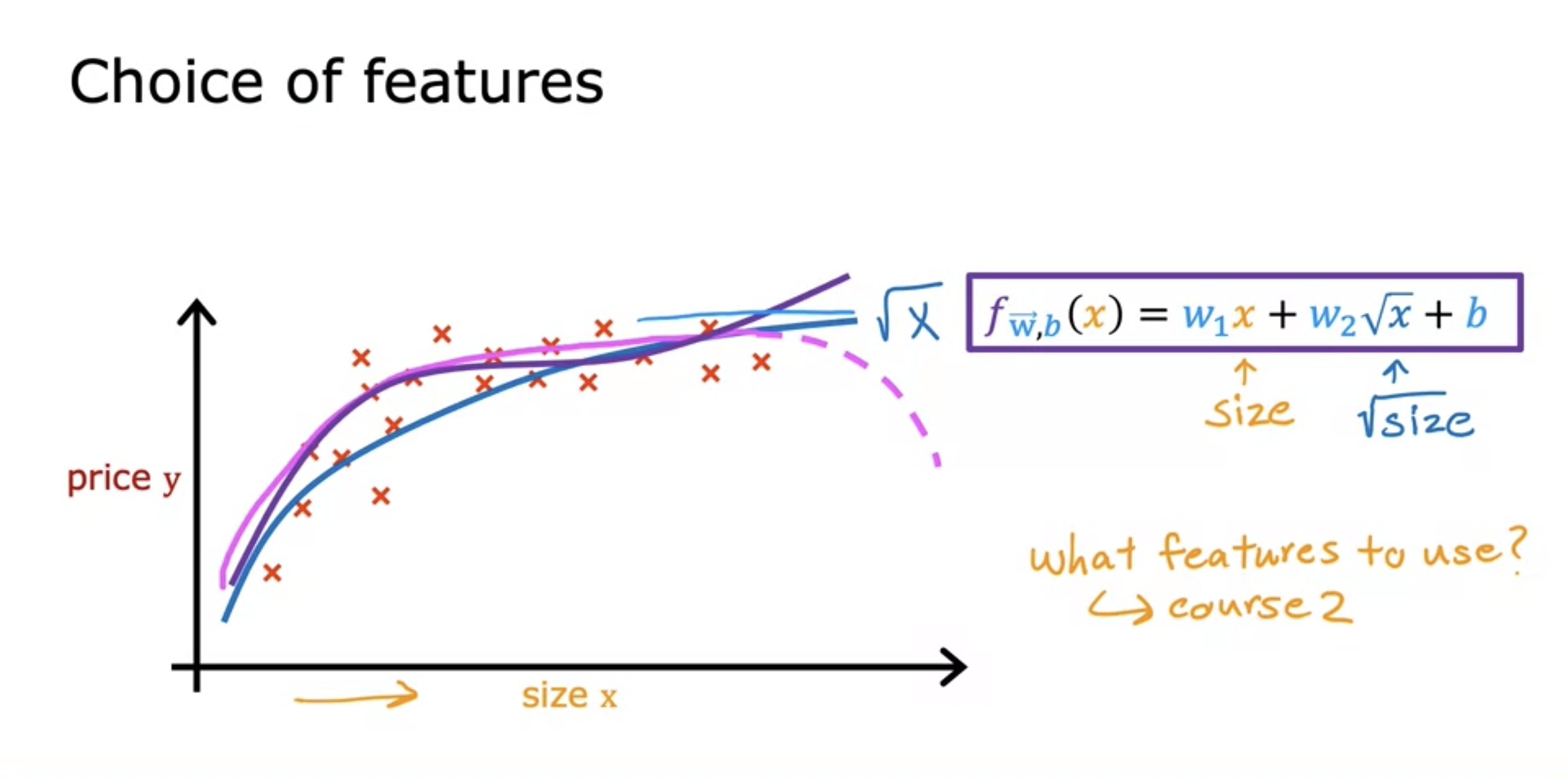
- Square rooting the size and adding it as a new feature can make the model more fitting.
6. Practice Lab
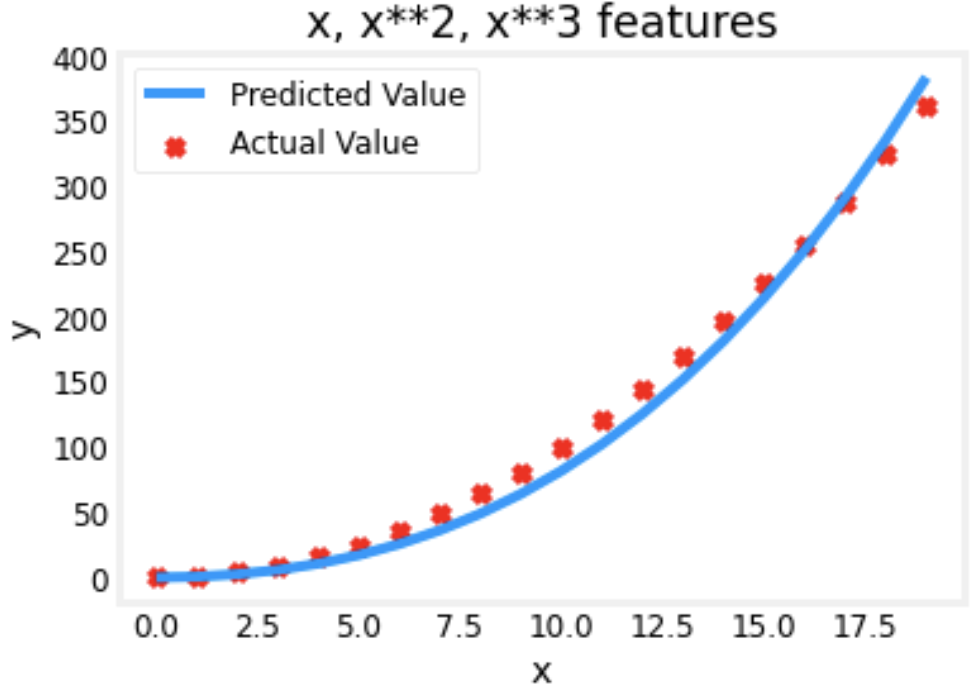
0.08𝑥+0.54𝑥^2+0.03𝑥^3+0.0106
- for y = x**2, the gradient descent shows a greater weight for x^2 alpha value, because it is the feature closest with the y value.
- Using feature engineering, even non-linear functions can be plotted with linear regression.
- Feature scaling is important for feature engineering, especially with larger exponents.