Reference
๐ A Simple Introduction to Sequence to Sequence Models
Seq to Seq๋ฅผ ๊ณต๋ถํ๋ค๊ฐ Architecture์ ๋ํ ์ดํด๊ฐ ์ ๋์ง ์์, ํด๋น ํฌ์คํ
์ ์ฐพ์๊ณ ์ด๋ฅผ ๋ฐํ์ผ๋ก ๊ณต๋ถํ๊ณ ์ ๋ฆฌํฉ๋๋ค.
๋ด์ฉ์ ์ค๋ฅ๊ฐ ์๋ ๊ฒฝ์ฐ ๋๊ธ๋ก ์๋ ค์ฃผ์๋ฉด ๊ฐ์ฌํ๊ฒ ์ต๋๋ค.
Overview
sequence to sequence models์ machine translation, video captioning, image captioning, question answering ๋ฑ์ ๋ถ์ผ์์ ์ฌ์ฉ๋ฉ๋๋ค.
ํด๋น ๊ฐ๋
์ ์ดํดํ๊ธฐ ์ํด neural network์ ๋ํ ๊ฐ๋
์ ์ ์๊ณ ์์ด์ผ ํ๋ฉฐ, ํนํ RNN(recurrent neural network), LSTM(long-short-term-memory), GRU model์ ๋ํด ์๊ณ ๊ณ์๋๊ฒ ์ข์ต๋๋ค!
์ ๋ ์ด์ํ๊ฒ RNN, LSTM, GRU ์ด์ seq to seq, attention, transformer ... ๋ฑ ๋ท๋ถ๋ถ ์ง๋๊ฐ ์ ์ ๋๊ฐ๋๋ฐ, ํฌ๊ธฐํ์ง ์๊ณ ๊ณ์ ๊ฐ์์ ๋ํ๊ตฌ๋ฅผ ์ฐพ์์ผ๋ฉด ์ข๊ฒ ์ต๋๋ค.
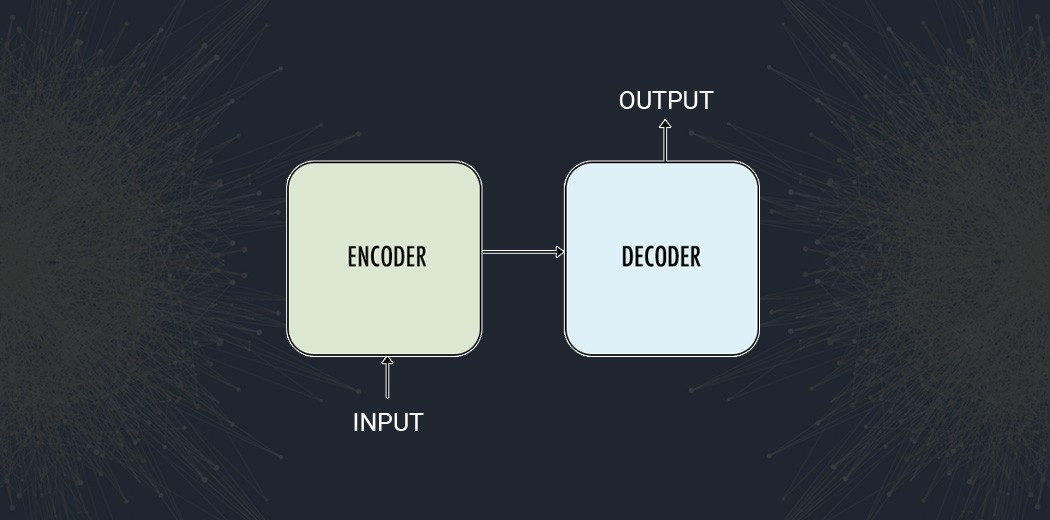
Use Cases
Sequence to sequence ๋ชจ๋ธ์ ์์์ ์ธ๊ธํ๋ฏ ์ผ์์ ์ผ๋ก ์ ํ๋ ์๋ง์ ์์คํ
์
๋๋ค.
์๋ฅผ ๋ค์ด seq2seq ๋ชจ๋ธ์ Google ๋ฒ์ญ, ์์ฑ ์ง์ ์ฅ์น ๋ฐ ์จ๋ผ์ธ ์ฑ๋ด๊ณผ ๊ฐ์ ์ ํ๋ฆฌ์ผ์ด์
์ ์ง์ํฉ๋๋ค.
๋ค์์ ์ผ๋ถ ์์ฉ ํ๋ก๊ทธ๋จ์
๋๋ค.
Machine translation
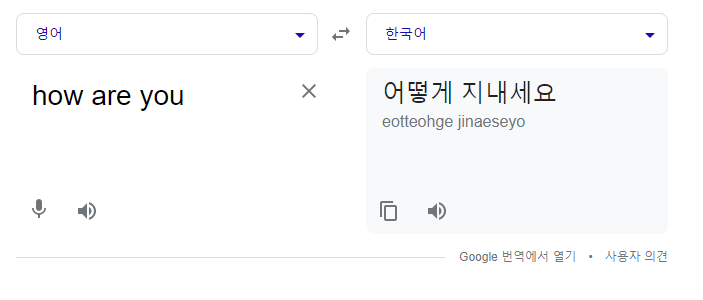
Speech recognition
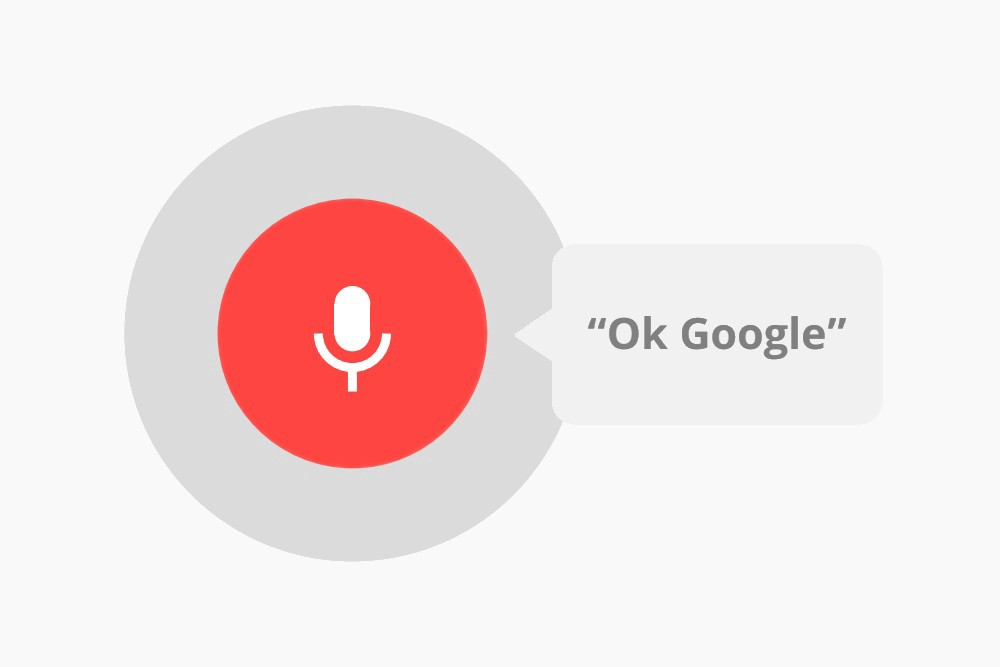
๋ฑ์ด ์์ต๋๋ค.
seq2seq(sequence to sequence)๋ ๋ชจ๋ธ์ ํนํ sequence ๊ธฐ๋ฐ์ ๋ฌธ์ ๋ฅผ ํด๊ฒฐํ ์๋ฃจ์
์ด๋ฉฐ, ํนํ input๊ณผ output์ size๋ category๊ฐ ๋ค๋ฅธ ์ ์ ๋ฌธ์ ์ ์ ํด๊ฒฐํฉ๋๋ค.
encoder-decoder architecture
seq2seq์๋ encoder์ decoder๋ผ๋ ๋ ๊ฐ์ง ๊ตฌ์ฑ ์์๊ฐ ์์ต๋๋ค.
encoder
- encoder์ decoder๋ ๋ชจ๋ LSTM ๋ชจ๋ธ์
๋๋ค.
- encoder๋ input sequence๋ฅผ ์ฝ๊ณ internal state vectors ๋ context vector(LSTM์ hidden state, cell state vectors๋ผ๊ณ ๋ ํฉ๋๋ค.)๋ก ์ ๋ณด๋ฅผ ์์ฝํฉ๋๋ค.
- encoder์ ์ถ๋ ฅ์ ๋ฒ๋ฆฌ๊ณ , internal states๋ง ์ ์งํฉ๋๋ค.
- context vector๋ decoder๊ฐ ์ ํํ ์์ธก์ ํ ์ ์๋๋ก ๋ชจ๋ input elements์ ๋ํด ์ ๋ณด๋ฅผ ์์ถํ๋ ๊ฒ์ ๋ชฉํ๋ก ํฉ๋๋ค.
- hidden state hiโ๋ ๋ค์ ๊ณต์์ ์ํด ๊ณ์ฐ๋ฉ๋๋ค.
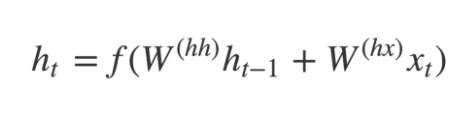
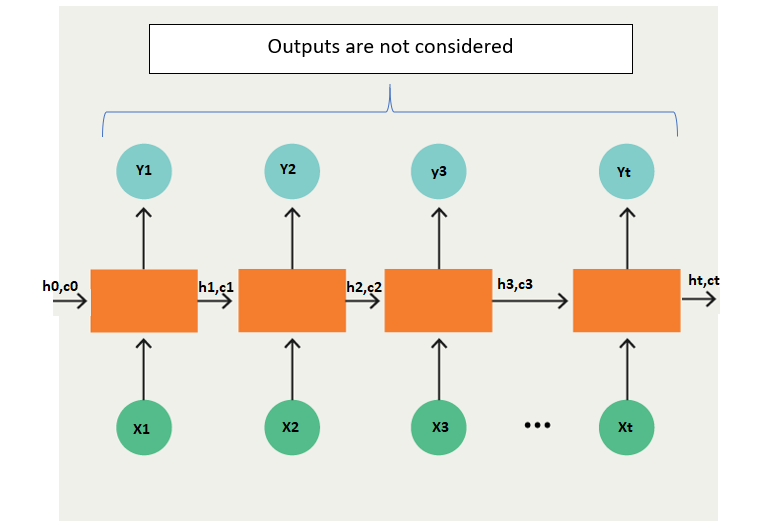
LSTM์ ๋ฐ์ดํฐ๋ฅผ ์์๋๋ก ์ฝ๋๋ฐ์.
์
๋ ฅ์ ๊ธธ์ด 't'์ sequence์ ๊ฒฝ์ฐ LSTM์ 't'์ time step์์ ์ด๋ฅผ ์ฝ์ต๋๋ค.
- xiโ: i๋ฒ์งธ time step์ input sequence
- hiโ ๋ฐ ciโ: LSTM์ ๊ฐ time step์์ 2๊ฐ์ง state(hidden state์ ๊ฒฝ์ฐ 'h', cell state์ ๊ฒฝ์ฐ 'c')๋ฅผ ์ ์งํฉ๋๋ค.
- yiโ: time step i์์์ output sequene. yiโ๋ ์ค์ ๋ก softmax activation์ ์ฌ์ฉํ์ฌ ์์ฑ๋ ์ ์ฒด vocabulary์ ๋ํ ํ๋ฅ ๋ถํฌ์
๋๋ค. ๋ฐ๋ผ์ ๊ฐ yiโ๋ ํ๋ฅ ๋ถํฌ๋ฅผ ๋ํ๋ด๋ 'vocab_size' ํฌ๊ธฐ์ vector์
๋๋ค.
decoder
- decoder๋ ์ด๊ธฐ state๊ฐ ์ธ์ฝ๋ LSTM์ ์ต์ข
state๋ก ์ด๊ธฐํ๋๋ LSTM์
๋๋ค.
- ์ฆ encoder์ ์ต์ข
cell์ context vector๊ฐ decoder network์ ์ฒซ ๋ฒ์งธ cell์ ์
๋ ฅ๋ฉ๋๋ค.
- ์ด๋ฐ initial states์ ์ฌ์ฉํด์ decoder๋ output sequence ์์ฑ์ ์์ํ๊ณ , ์ถ๋ ฅ์ ํฅํ ์ถ๋ ฅ์๋ ๊ณ์ ๊ณ ๋ ค๋ฉ๋๋ค.
- ์ฌ๋ฌ LSTM unit์ stack์ผ๋ก ๊ฐ unit์ด time step t์์ ์ถ๋ ฅ ytโ๋ฅผ ์์ธกํฉ๋๋ค.
- ๊ฐ ๋ฐ๋ณต unit์ ์ด์ unit์ hidden state๋ฅผ ๋ฐ์๋ค์ด๊ณ , ์์ฒด hidden state ๋ฟ๋ง ์๋๋ผ ์์ฑํ๊ณ ์ถ๋ ฅํฉ๋๋ค.
๋ชจ๋ hidden state hiโ๋ ๋ค์ ๊ณต์์ ์ฌ์ฉํ์ฌ ๊ณ์ฐ๋ฉ๋๋ค.
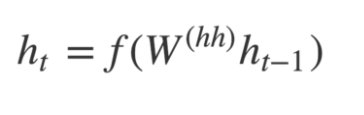
์๊ฐ ๋จ๊ณ t ์์ ์ถ๋ ฅ y_t ๋ ๋ค์ ๊ณต์์ ์ฌ์ฉํ์ฌ ๊ณ์ฐ๋ฉ๋๋ค.
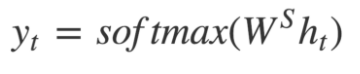
๊ฐ ๊ฐ์ค์น W(S)์ ํจ๊ป ํ์ฌ time step์์ hidden state๋ฅผ ์ฌ์ฉํ์ฌ output์ ๊ณ์ฐํฉ๋๋ค.
Softmax๋ ์ต์ข
์ถ๋ ฅ(์: question-answering problem์ word)๋ฅผ ๊ฒฐ์ ํ๋ ๋ฐ ๋์์ด ๋๋ ํ๋ฅ ๋ฒกํฐ๋ฅผ ๋ง๋๋ ๋ฐ ์ฌ์ฉ๋ฉ๋๋ค.
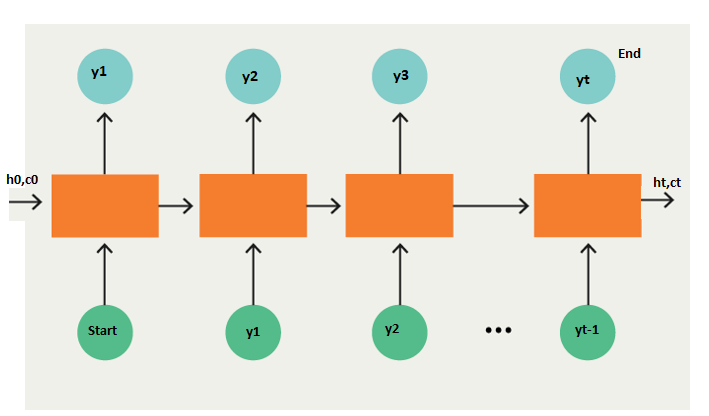
์์ ์ด๋ฏธ์ง์ ๊ฐ์ด output sequence์ ๋ ๊ฐ์ token์ ์ถ๊ฐํฉ๋๋ค.
Example
์์ reference๋ก ๋จ๊ธด posting์์ ์์ ๋ฌธ์ฅ์ ์ ์ํฉ๋๋ค.
โSTART_ John is hard working _ENDโ.
๊ฐ์ฅ ์ค์ํ ์ ์ decoder์ ์ด๊ธฐ state(h0โ,c0โ)๊ฐ encoder์ ์ต์ข
state๋ก ๊ฒฐ์ ๋๋ค๋ ์ ์ธ๋ฐ์.
์ด ์ฌ์ค์ decoder๊ฐ encoder์ ์ํด encoding๋ ์ ๋ณด์ ๋ฐ๋ผ output sequence๋ฅผ ์์ํ๋๋ก ํ๋ จ๋์์์ ์๋ฏธํฉ๋๋ค.
๋ง์ง๋ง์ผ๋ก ๊ฐ time step์์ ์์ธก๋ output์ ๋ํด loss๋ฅผ ๊ณ์ฐํ๊ณ , network์ parameter๋ฅผ updateํ๊ธฐ ์ํด error๋ฅผ time์ ๋ฐ๋ผ backpropagationํฉ๋๋ค.
์ถฉ๋ถํ ๋ง์ ์์ data๋ก ๋ ์ค๋ ๊ธฐ๊ฐ ๋์ network๋ฅผ ํ๋ จํ๋ฉด ๋ ์ข์ ์์ธก ๊ฒฐ๊ณผ๋ฅผ ์ป์ ์ ์์ต๋๋ค.
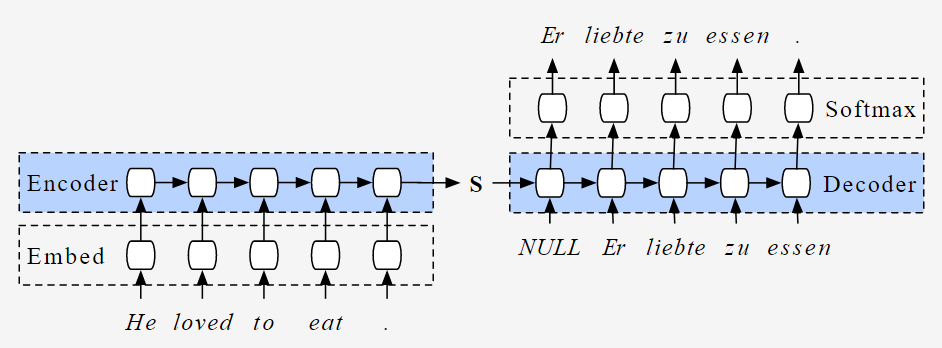
Overall Encoder-Decoder Architecture
- inferenceํ๋ ๋์ ํ ๋ฒ์ ํ๋์ ๋จ์ด๋ฅผ ์์ฑํฉ๋๋ค.
- decoder์ ์ด๊ธฐ state๋ encode์ ์ต์ข
state์ ์ํด ์ค์ ๋ฉ๋๋ค.
- ์ด๊ธฐ input์ ํญ์ START token์
๋๋ค.
- ๊ฐ time step์์ decoder์ state๋ฅผ ์ ์งํ๊ณ , ๋ค์ time step์ initial state๋ก ์ค์ ํฉ๋๋ค.
- ๊ฐ time step์์ ์์ธก๋ ์ถ๋ ฅ๊ฐ์ ๋ค์ time step์ input์ผ๋ก ์ ๊ณต๋ฉ๋๋ค.
- decoder๊ฐ END token์ ์์ธกํ ๋ loop๋ฅผ ๋์ต๋๋ค.
Encoder-Decoder Models์ ์ฅ๋จ์
- memory๊ฐ ์์ฃผ ์ ํ์ ์
๋๋ค.
์ฌ๊ธฐ์ S ๋๋ W๋ผ๊ณ ๋ถ๋ฅด๋ LSTM์ ๋ง์ง๋ง hidden state๋ ๋ฒ์ญํด์ผ ํ๋ ์ ์ฒด ๋ฌธ์ฅ์ ๋ฒผ๋ฝ์น๊ธฐํ๊ธฐ ์ํด ๋
ธ๋ ฅํ๋ ํํธ์
๋๋ค.
S ๋๋ W๋ ์ผ๋ฐ์ ์ผ๋ก ๊ธธ์ด๊ฐ ๋ช ๋ฐฑ ๋จ์์ ๋ถ๊ณผํ๋ฐ, ์ด ๊ณ ์ ์ฐจ์ vector์์ ๊ฐ์ ๋ก ์ ์ฉํ๋ ค๊ณ ํ๋ฉด neural network๊ฐ ๋ ๋ง์ด ์์ค๋ฉ๋๋ค.
lossy compression์ ์ํํด์ผ ํ๋ค๋ ์ธก๋ฉด์์ neural network๋ฅผ ๊ณ ๋ คํ๋ ๊ฒ์ด ๋ ์ ์ฉํ๋ค๊ณ ํฉ๋๋ค.
- ์ผ๋ฐ์ ์ผ๋ก ์ ๊ฒฝ๋ง์ด ๊น์์๋ก ํ๋ จํ๊ธฐ๊ฐ ๋ ์ด๋ ต์ต๋๋ค. recurrent neural network์ ๊ฒฝ์ฐ sequence๊ฐ ๋ ๊ธธ์๋ก neural network๋ time step์ ๋ฐ๋ผ ๋ ๊น์ด์ง๋๋ค.
์ด๋ก ์ธํด RNN์ด ํ์ตํ๋ ๋ชฉํ์ gradient๊ฐ backward ๊ณผ์ ์์ vanishing๋ฉ๋๋ค.
LSTM๊ณผ ๊ฐ์ด gradient vanishing์ ๋ฐฉ์งํ๊ธฐ ์ํด ํน๋ณํ ์ ์๋ RNN์ ์ฌ์ฉํ๋๋ผ๋ ์ด๋ ์ฌ์ ํ ๊ทผ๋ณธ์ ์ธ ๋ฌธ์ ์ ์ผ๋ก ๋จ์ ์์ต๋๋ค.
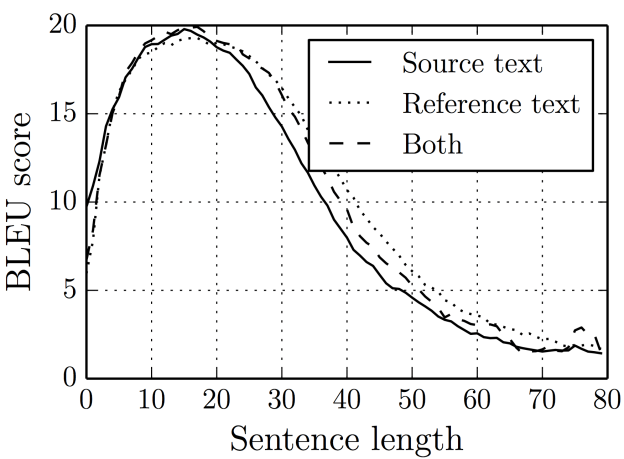
๋ํ ๋ณด๋ค ๊ฐ๋ ฅํ๊ณ ๊ธด ๋ฌธ์ฅ์ ์ํด Attention Models ๋ฐ Transformer์ ๊ฐ์ ๋ชจ๋ธ์ด ์์ต๋๋ค.
์ฌ๊ธฐ๊น์ง seq2sqe์ encoder, decoder๋ฅผ ์ดํด๋ณด์์ต๋๋ค.