Multi-modal Learning
한가지 타입의 data가 아니라 여러가지 타입의 data를 함께 사용하는 방법
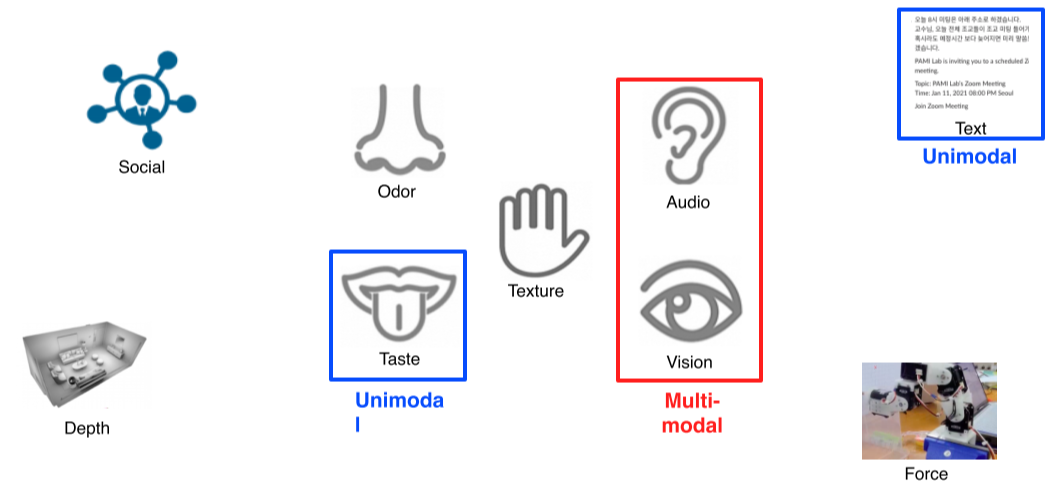
- CV라 vision centric하게 다룰 예정
Challenges
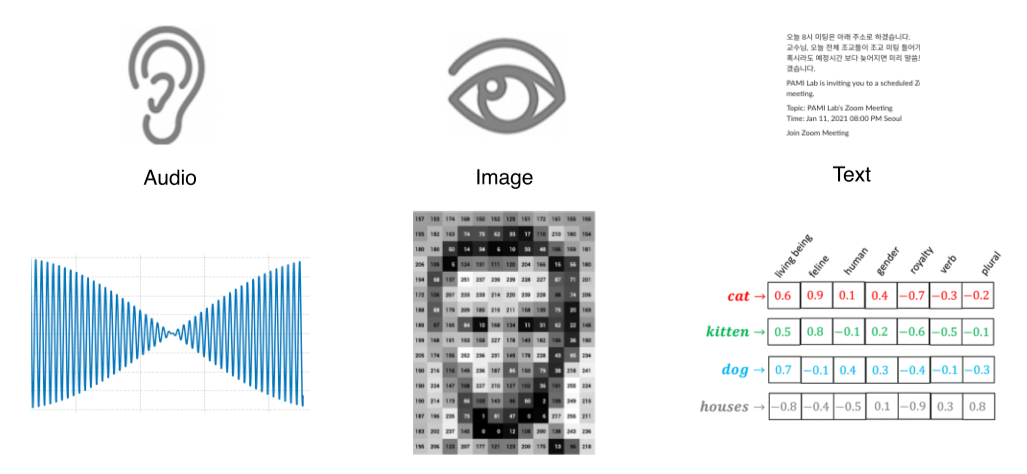
- Data 표현 형태가 제각각이라 다루기가 어려움
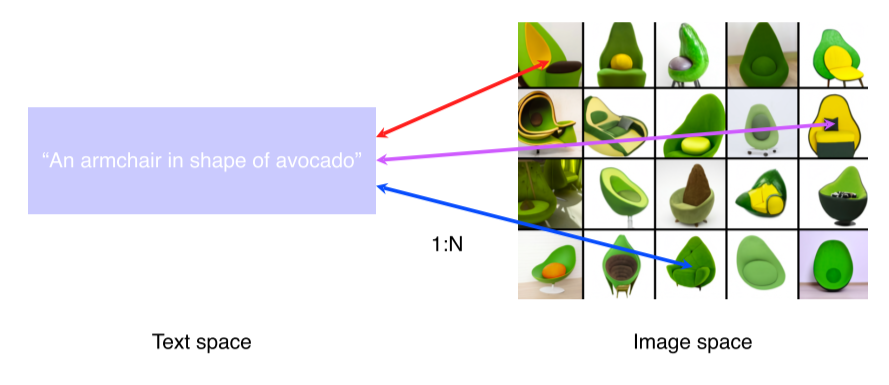
- 정보의 양과 feature space이 unbalance --> 1:N matching
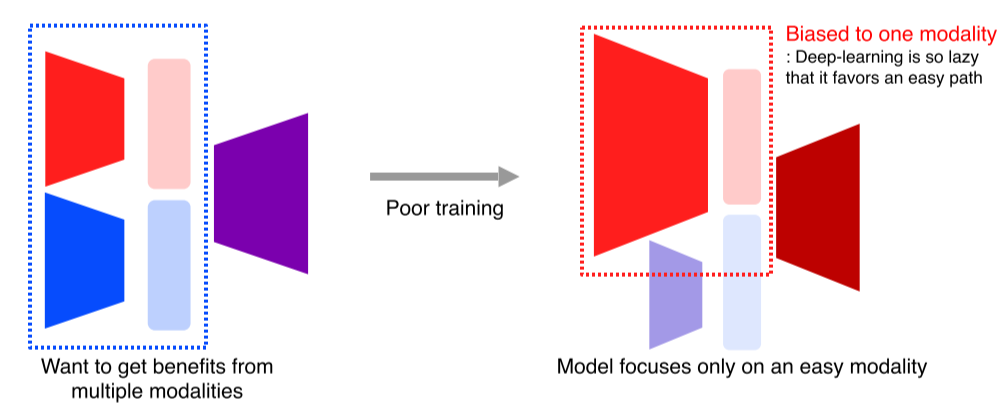
- 학습이 잘못되면 한가지 modal에만 편향돼서 성능이 더 떨어지는 경우가 있음
Methods
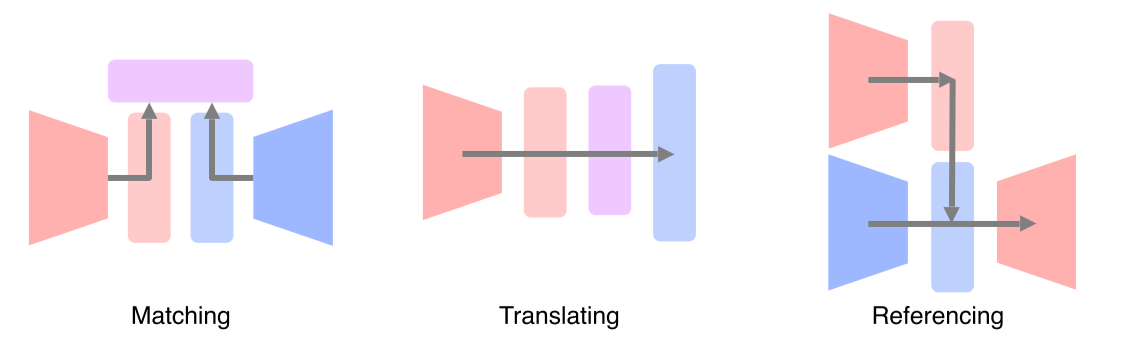
Matching
: 서로 다른 modality를 공통된 space로 보내서 match
Translating
: 하나의 modality를 다른 modality로 translate
Referencing
: 하나의 modality로 output을 내는데, 그때 다른 modality를 참조
Visual data & Text
Text embedding
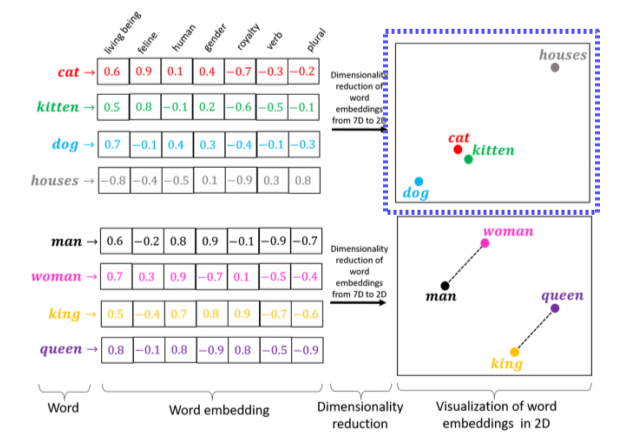
- Character 단위로 구분을 하면 사용하기 어려워서 기본 unit은 word 단위로 설정
- Word는 embedding vector로 표현
Multi-dimensional한 실수값을 갖게 해서 각 dimension에 대한 경향성을 나타냄
- Embedding vector를 low dimension(2D)에서 projection해보면
1) 연관이 있는 단어끼리의 거리는 가깝고
2) 단어끼리의 상관관계가 같으면 word 사이의 vector도 같게 나타남
--> Generalization!
Skip-gram model
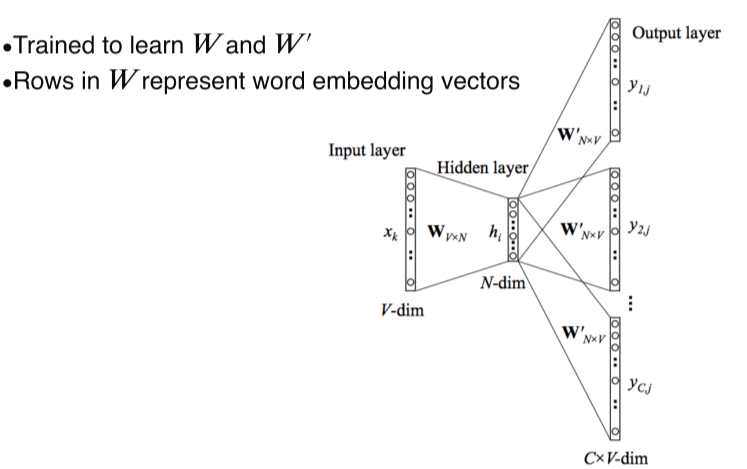
- W: word embedding vector
- xk: Input. Dimensional vector들은 one-hot vector라서 W랑 곱하면 그 word에 해당되는 embedding vector만 활성화됨
- hi: word embedding vector가 추출된 hidden layer
- Output layer: hi와 W′를 곱해서 나온 결과로 xk 전후에 어떤 단어가 나오는지 패턴을 학습
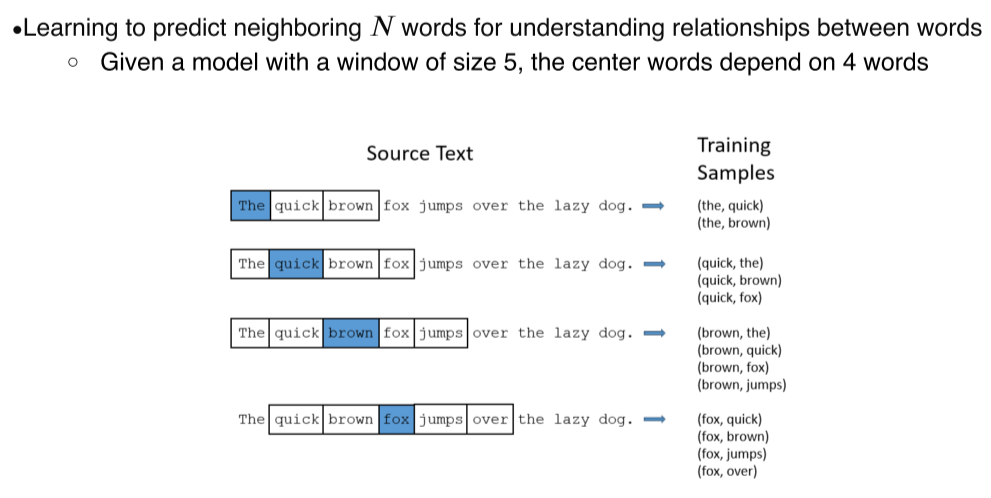
- 주변의 N개의 word를 predict하게 학습해서 주변과의 관계를 학습
Joint embedding
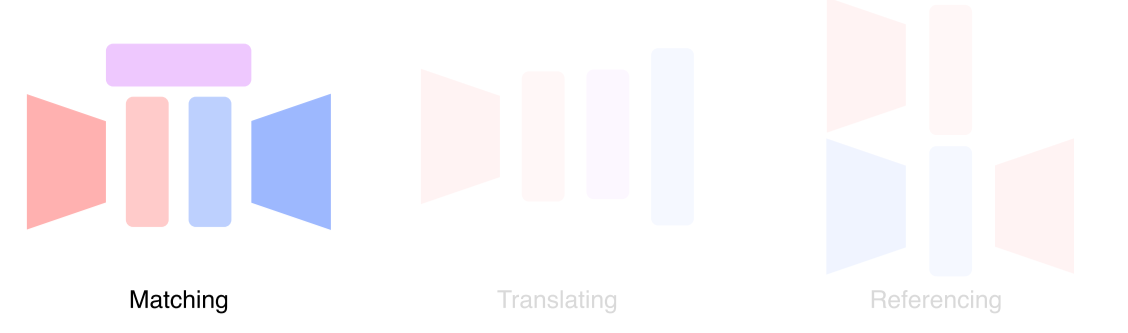
- Matching을 하기 위한 embedding vector를 학습하는 방법
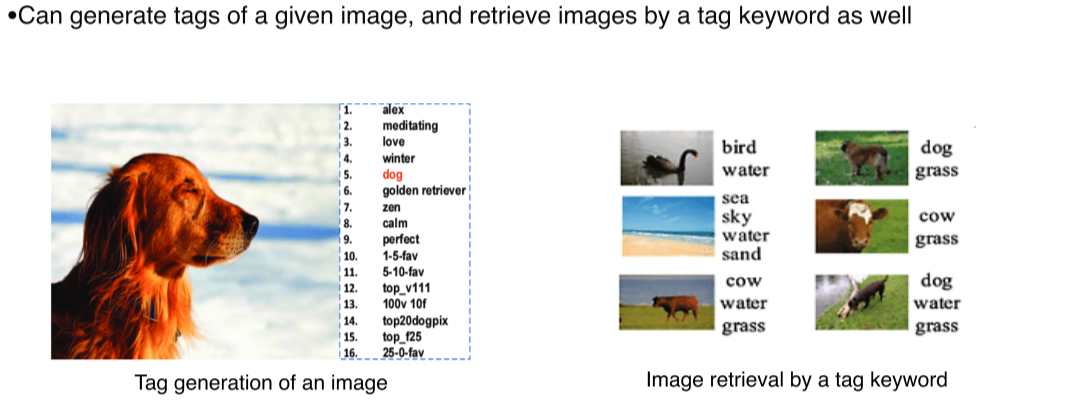
- Tag -> image, image -> tag
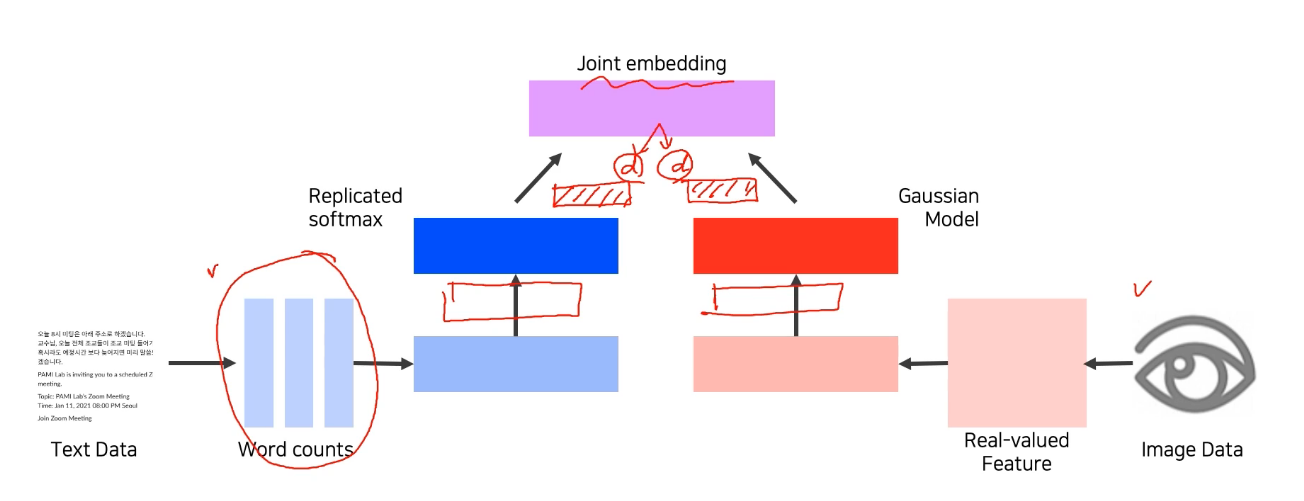
- Text, image 모두 d-dimensional feature vector로 변환 후, 두 vector가 호환성이 있도록 joint embedding space를 학습
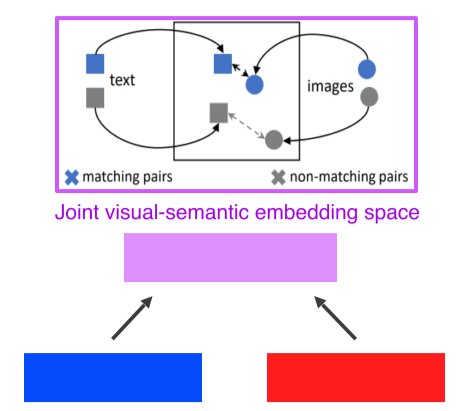
- Text, image를 같은 embedding space에 mapping 후, matching되는 embedding 간의 distance는 줄이고(push) non-matching일 경우엔 distance를 키움(pull)
--> Distance를 기반으로 학습하기 때문에 metric learning
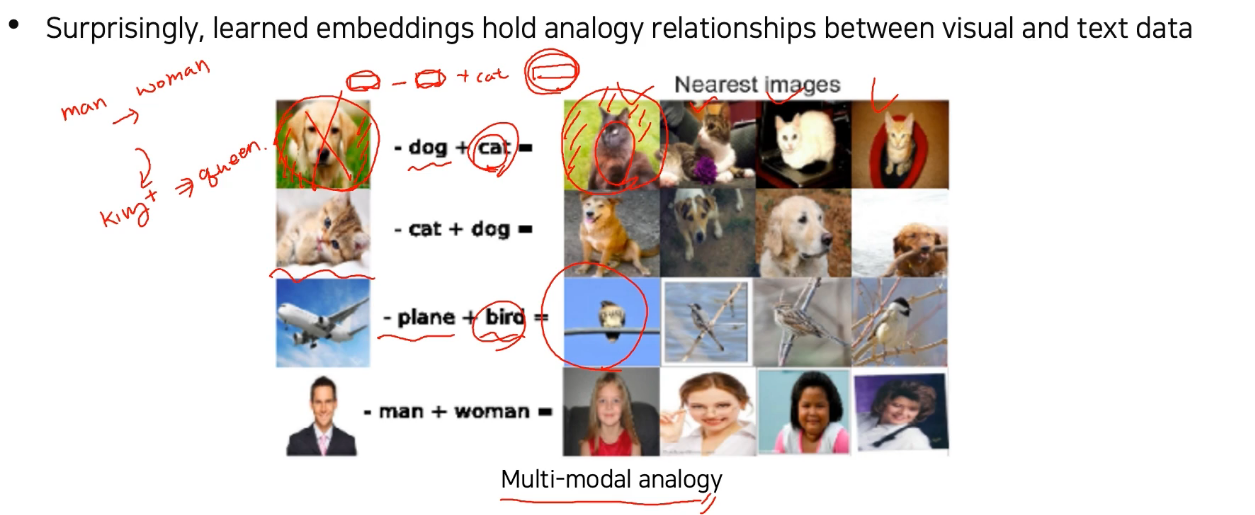
- 풀밭에 있는 개 사진에서 dog embedding을 빼고 cat embedding을 더했더니 풀밭에 있는 고양이 사진이 나옴
--> Dog을 제외한 나머지 embedding vector는 유지됐기 때문!
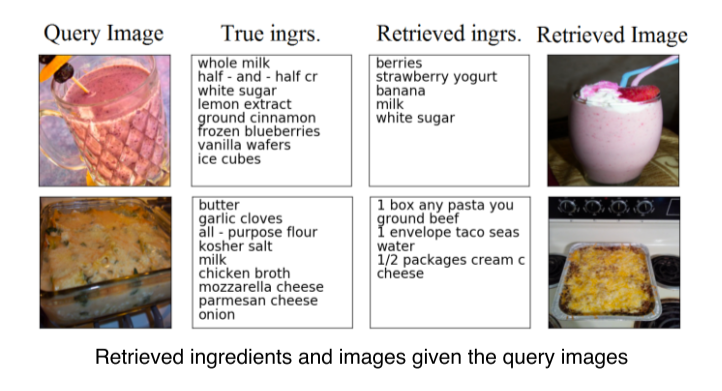
- Food image에서 recipe를 추출하거나, recipe에서 food image를 얻어낼 수도 있음
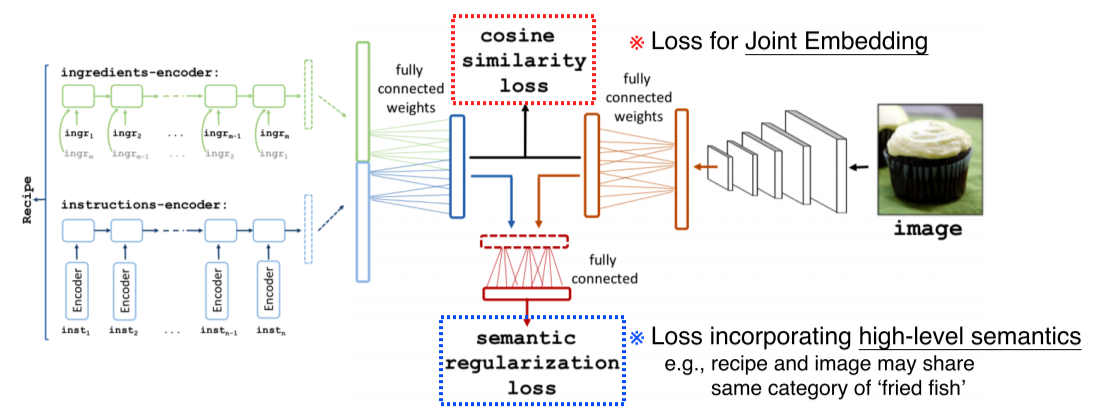
-
Recipe에는 ingredient, instruction의 순서가 정해져있기 때문에 RNN 계열의 model로 ingredient, instruction를 fixed vector로 만듬
-
Joint embedding의 loss는 cosine similarity loss를 사용해 food image와 recipe가 연관이 있다면 cosine similarity를 높게 만든다
-
2번째 loss로 semantic regularization loss를 사용해 cosine similarity loss로 해결되지 않는 부분의 guide를 줘서 high-level semantics를 해결
Cross modal translation
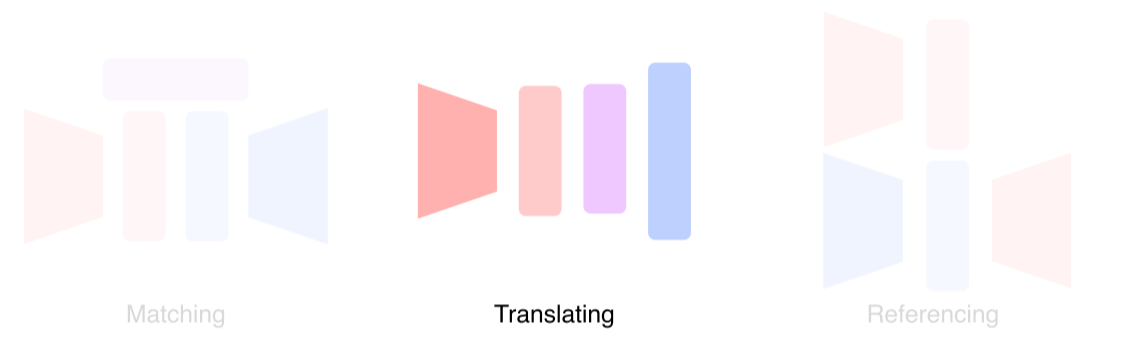
- Multi-modal을 translation으로 활용하는 방법
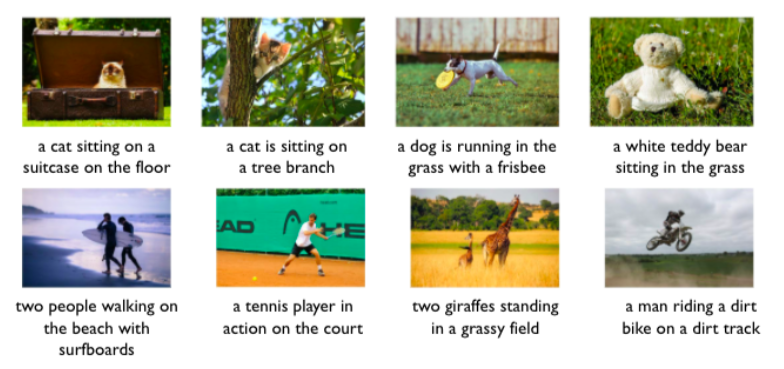
Image captioning
: image가 주어졌을때, image를 가장 잘 설명하는 text description을 생성해내는 방법
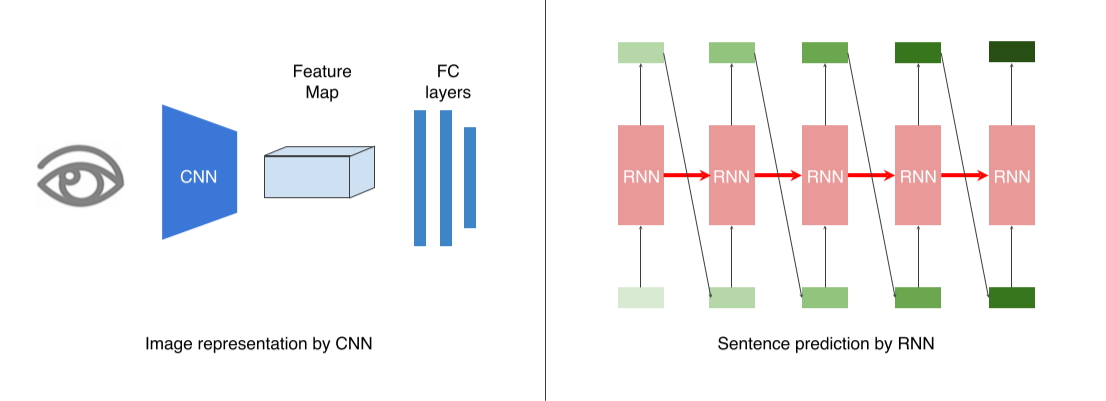
- Image를 input으로 다루기 위해서 CNN을 사용하고,
Sentence를 출력하기 위해 RNN을 사용
Show and tell
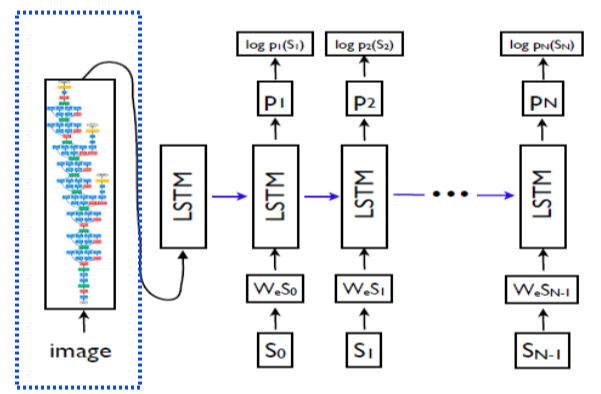
- Image는 pre-trained ImageNet을 사용해 fixed dimensional vector로 변환
- Fixed dimensional vector를 condition으로 제공, token을 input으로 넣어 LSTM은 단어를 출력함
Show, attend and tell
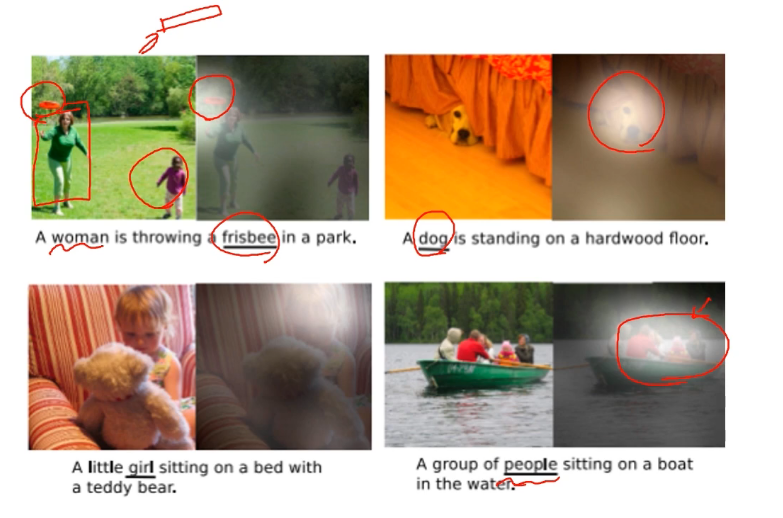
- 특정 word를 출력할 때 그 word를 image의 어떤 부분을 보고 출력했는지를 보여줌
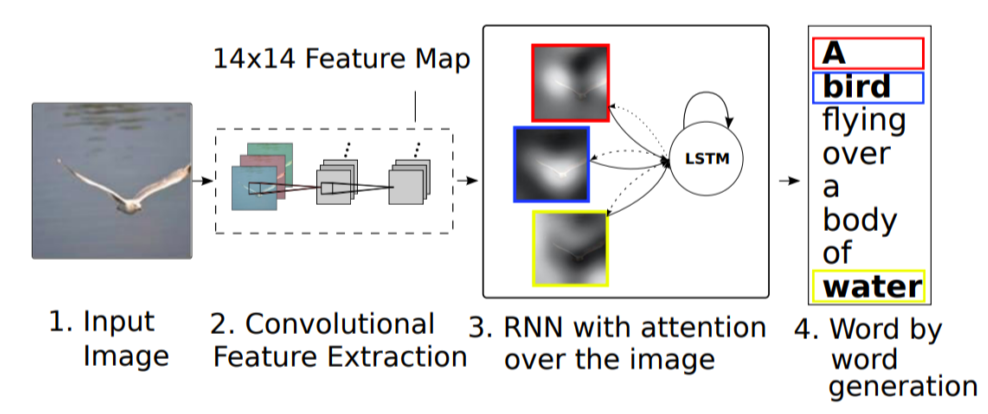
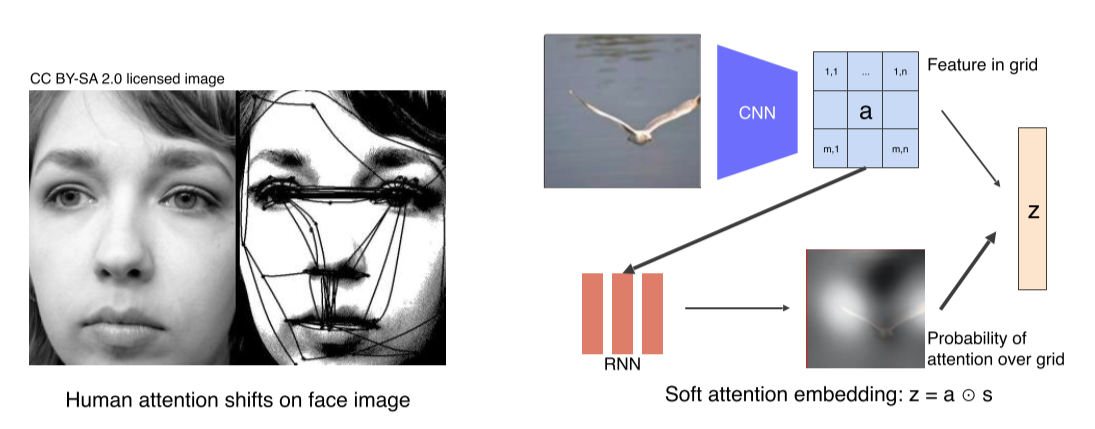
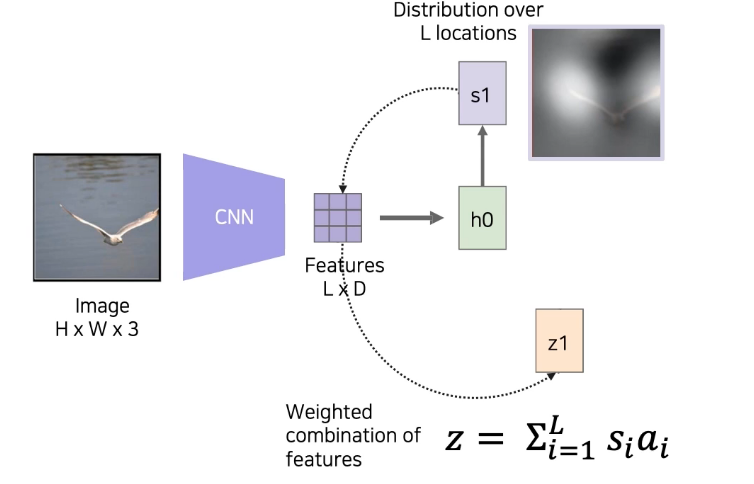
- Input image를 CNN을 거쳐 feature map으로 만듬
- Feature map을 LSTM의 condition으로 넣음
- 어떤 부분을 reference할지 결정하는 spatial attention s1 을 만듬
- s1을 weight로 사용해, feature map과 inner product해서 pixel dimensional vector z1 을 만듬
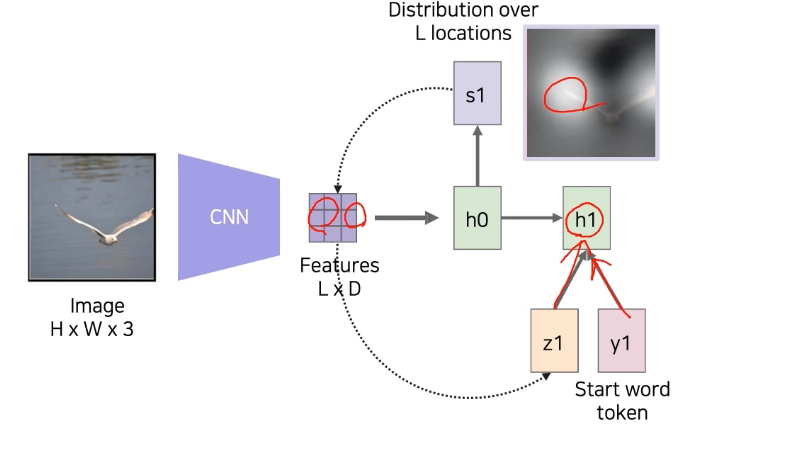
- z1을 condition으로, start token y1 과 함께 RNN에 넣어줌
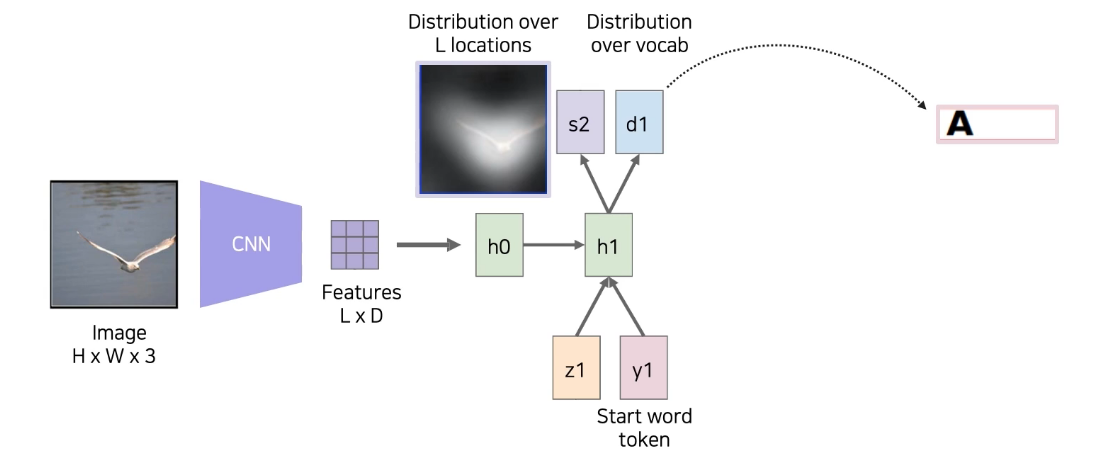
- RNN에서는 word 'A'를 출력하고 다음은 어떤 부분을 reference할지 예측하는 s2를 만듬
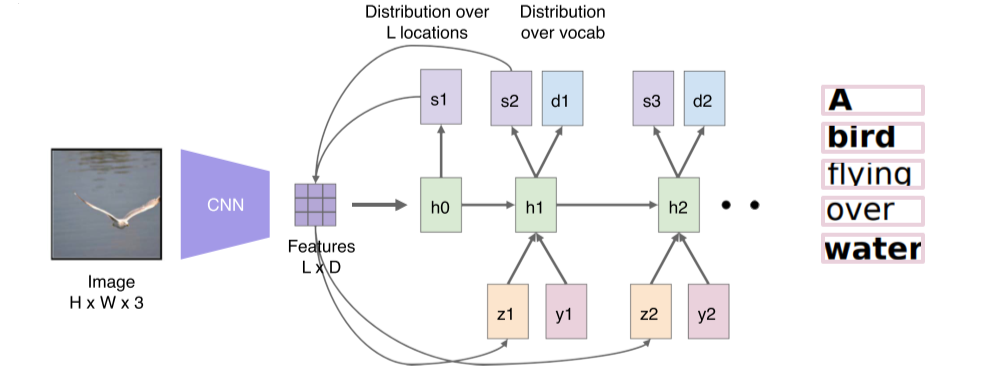
- s2 를 다시 feature map와 inner product해서 z2 라는 condition 생성
- y2 는 이전에 출력했던 단어인 'A'를 사용해 RNN에 넣어줌
- 다음 단계의 expected distribution인 s3 와 output word d2 를 생성
Text-to-image by generative model
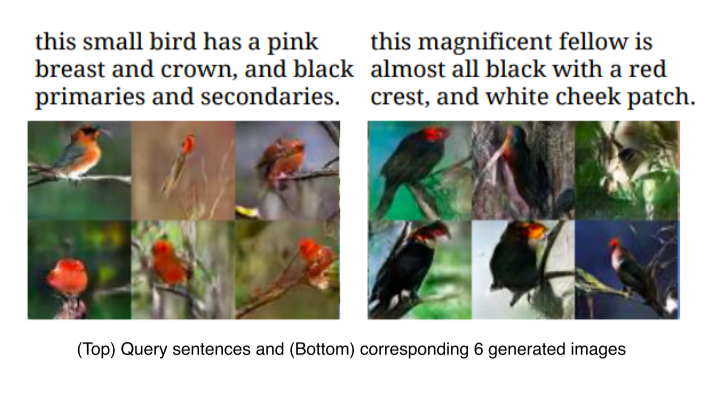
- 반대로 text -> image는 1:N mapping이기 때문에 generative model이 필요
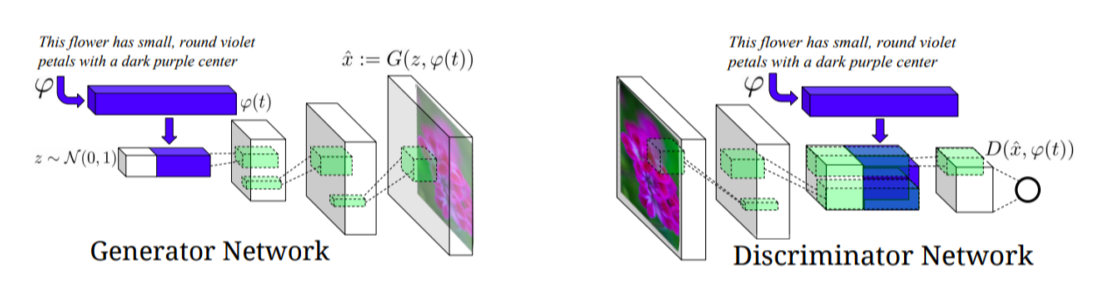
- Generator
- Text 전체를 fixed dimensional vector로 만들고, gaussian random code를 붙여서 같은 input에 대해 항상 똑같은 output이 나오는 것을 방지
- 이후 decoder를 거쳐 image를 generate
- Disriminator
- Input image를 encoder에서 low dimensional spatial feature로 만들고, generator에서 썼던 sentence 정보를 붙임
- Sentence condition 하에, input image가 True/False인지를 판별하도록 discriminator를 학습시킴
Cross modal reasoning
Cross modal 중 referencing을 이용하는 task
Vision question answering
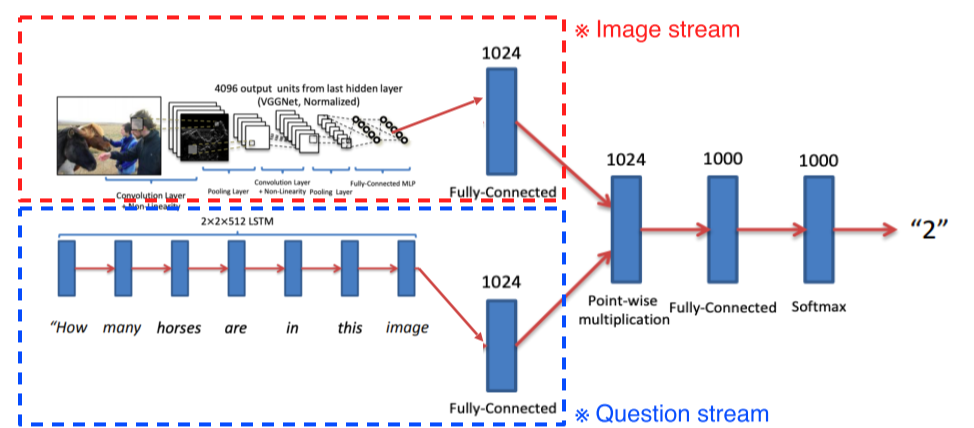
- 영상과 질문이 주어지면 답을 도출
- 질문: text 형태로 RNN을 거침
- 2개의 embedding vector가 interact할 수 있도록 해줌
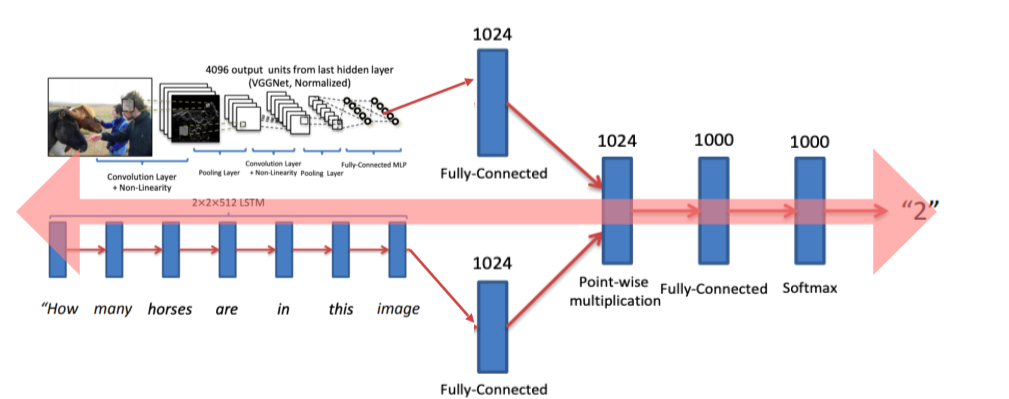
Visual data & Audio
Sound representation
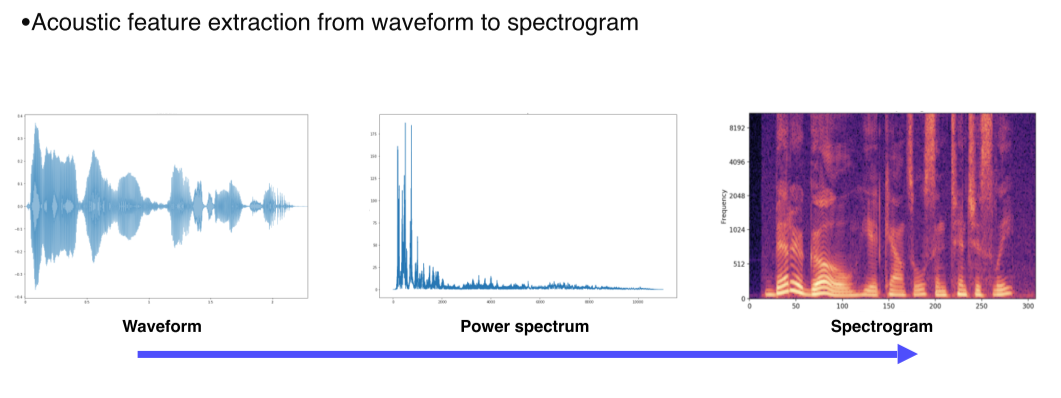
- Sound data는 1-D signal로 존재
--> Spectogram, MFCC 등의 형태로 변환해서 사용
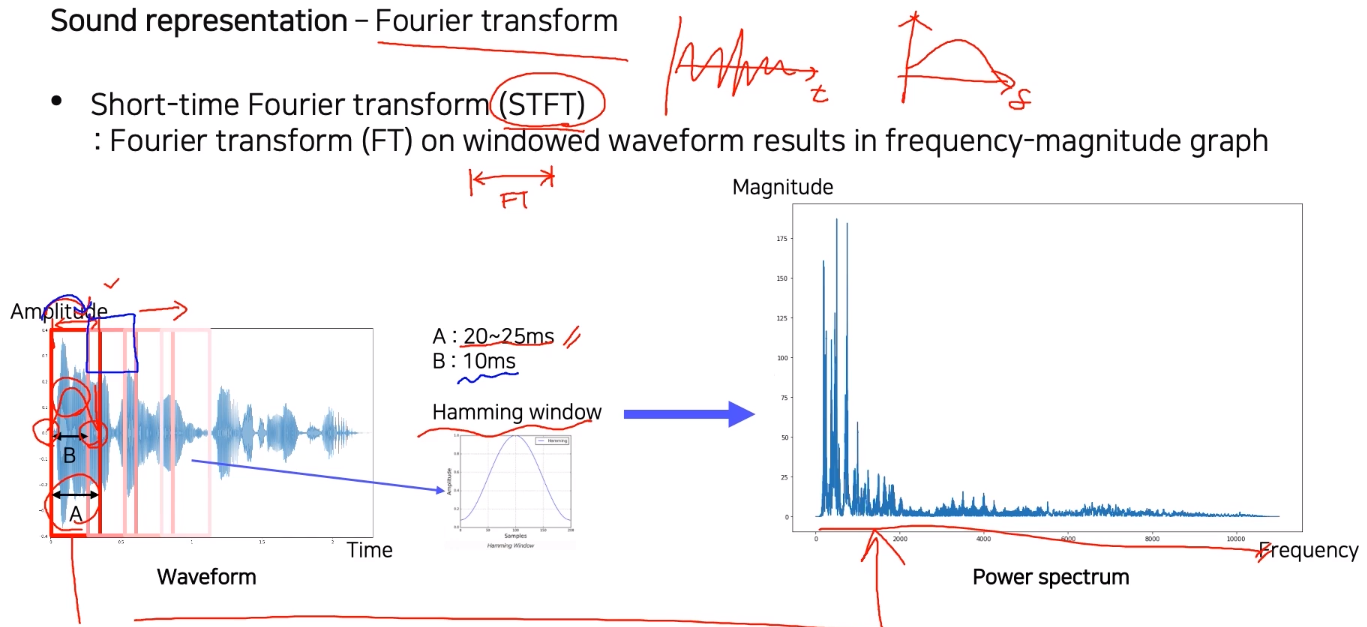
-
waveform 전체에 대해 fourier transform을 적용하면 시간축 t 에 대한 waveform을 주파수축 f 로 옮길 수 있음
-
하지만 그냥 옮기면 시간에 따른 변화를 파악할 수 없기 때문에 짧은 window 구간에 대해서만 FT를 적용
+ Hamming window로 중앙에 가까울수록 weight를 줌
-
Window는 discrete하게 일정 간격을 띄어서, 적당히 overlap시켜서 결정
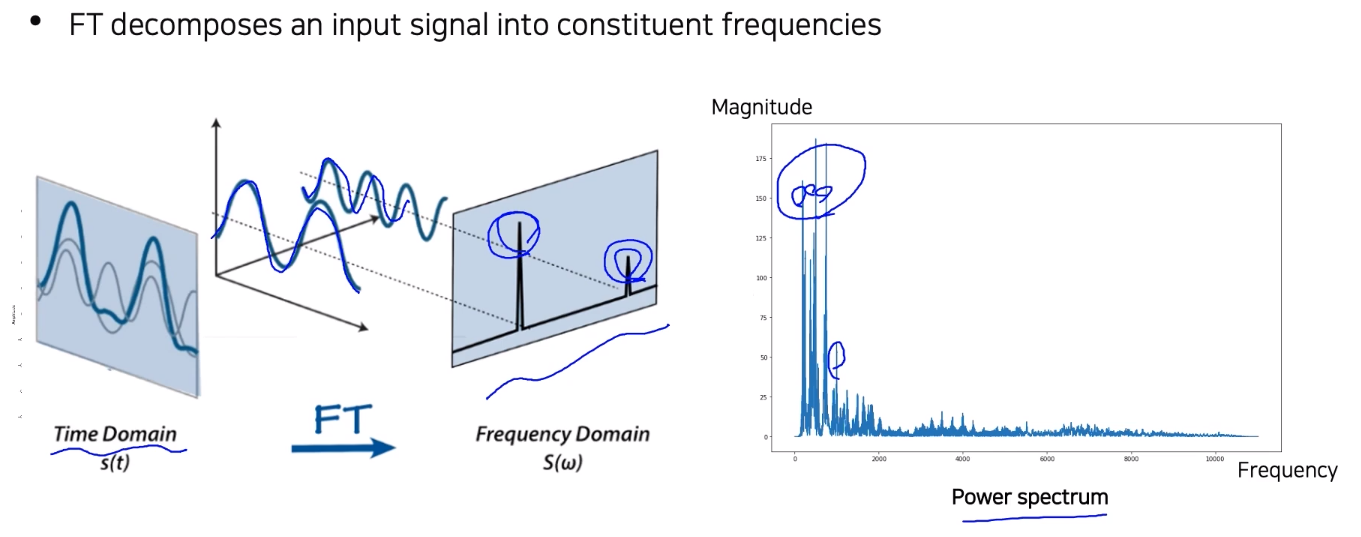
- Fourier transform으로 time 축의 input signal의 삼각함수를 분해해서 각 삼각함수가 어느정도 성분으로 들어있는지 비교해서 frequency 축에 출력
--> Power spectrum에서 각 frequency가 어느정도 들어있는지 확인 가능
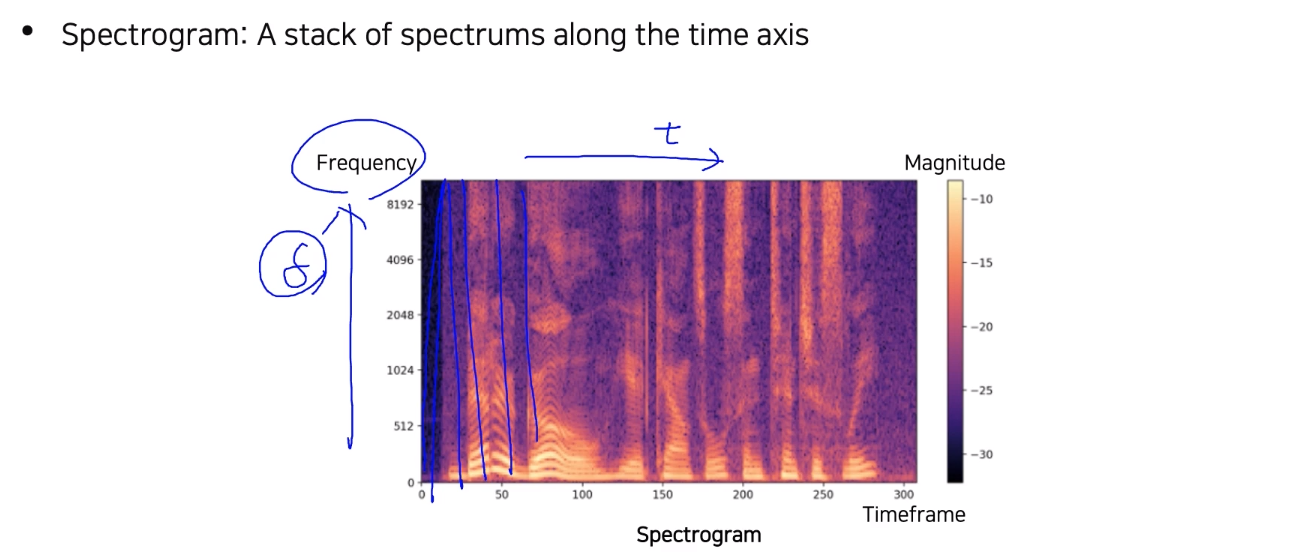
-
Spectogram: 각 spectrum을 세로로(시간축을 따라) stack
--> 시간에 따라 주파수 성분이 어떻게 변해가는지 눈으로 확인 가능
i.e. 색이 연할수록 해당 주파수 성분이 적고, 진할수록 많은 등
-
Keyword: Melspectogram
, MFCC
Joint embedding
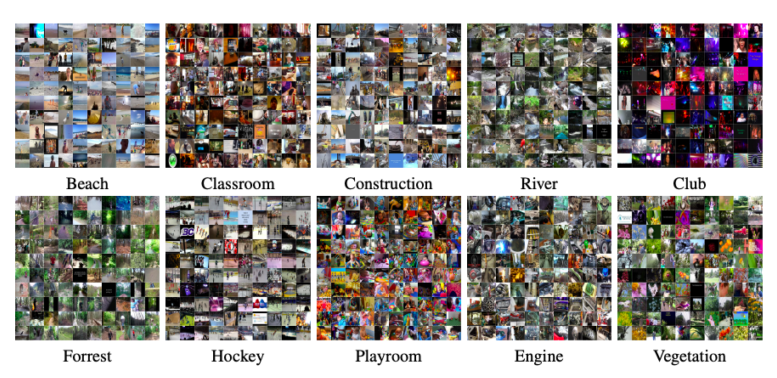
- Sound tagging: 소리로 해당 장면을 인식
SoundNet
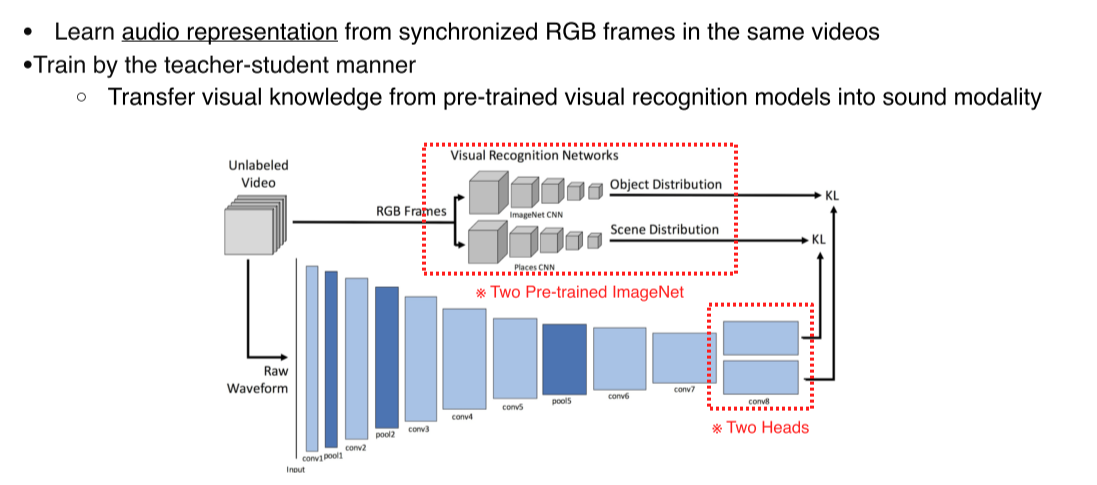
-
Video dataset의 image는 pre-trained visual recognition network를 사용, object와 scene을 판별
-
Video dataset의 audio는 1-D CNN에 입력, 2개의 head에서 object, scene을 판별 후 KL-div로 image부분의 출력과의 오차를 줄임
-
Image network는 fixed, audio network는 학습하기 때문에 teacher-student 방식
--> Visual knowledge를 sound에게 transfer
-
Raw waveform을 사용한 것이 특징(아직 MFCC 등이 발달하기 이전)
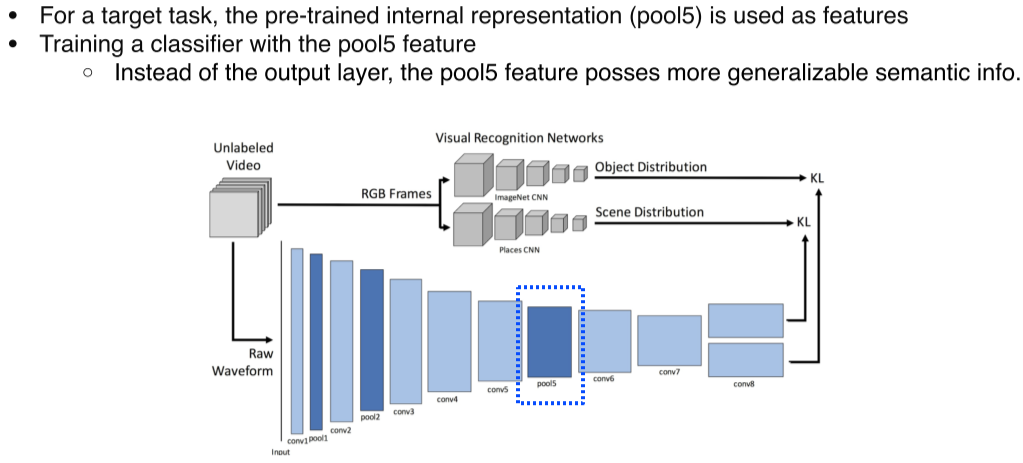
- Pool5가 학습된 대표적 feature라고 생각했기 때문에 target task에서는 pool5에 classifier를 추가해서 target task를 수행
--> 그보다 뒤쪽 layer는 KL-div로 visual output과 너무 닮아있어서(specifically optimized) pool5 정도가 더 generalized semantic info를 갖고 있을거라 판단
Cross modal translation
Speech2Face
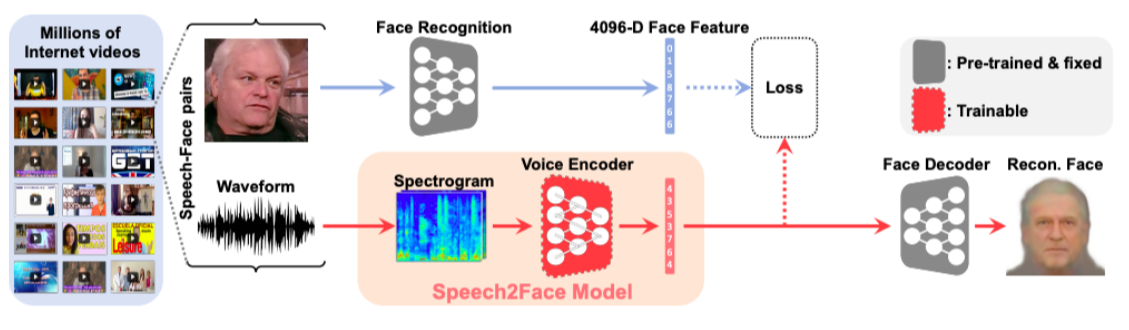
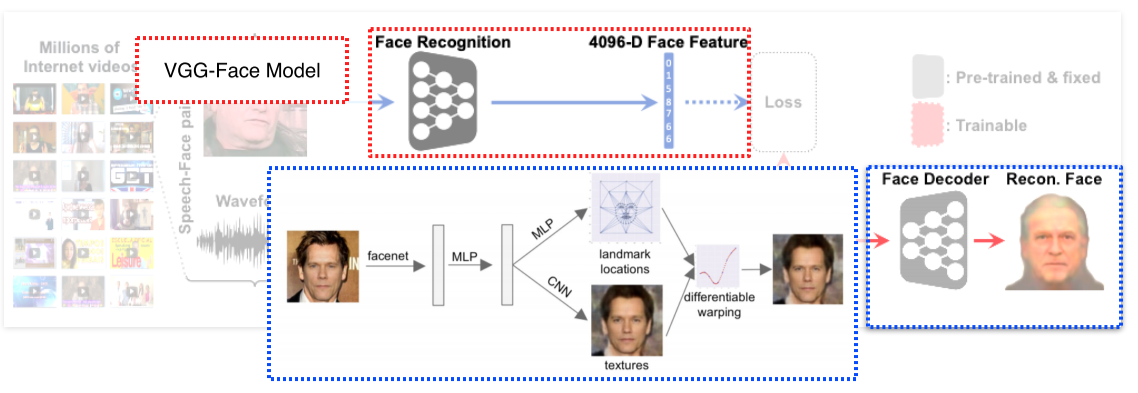
-
이를 위해 module network를 적극 활용
- 각자 task에 맞는 pretrained-network를 조합해 활용
-
VGG-Face Model: 얼굴 image가 들어오면 fixed dimensional vector로 표현
-
Face Decoder: face feature가 들어오면 정규화된 무표정의 정면 얼굴을 reconstruct
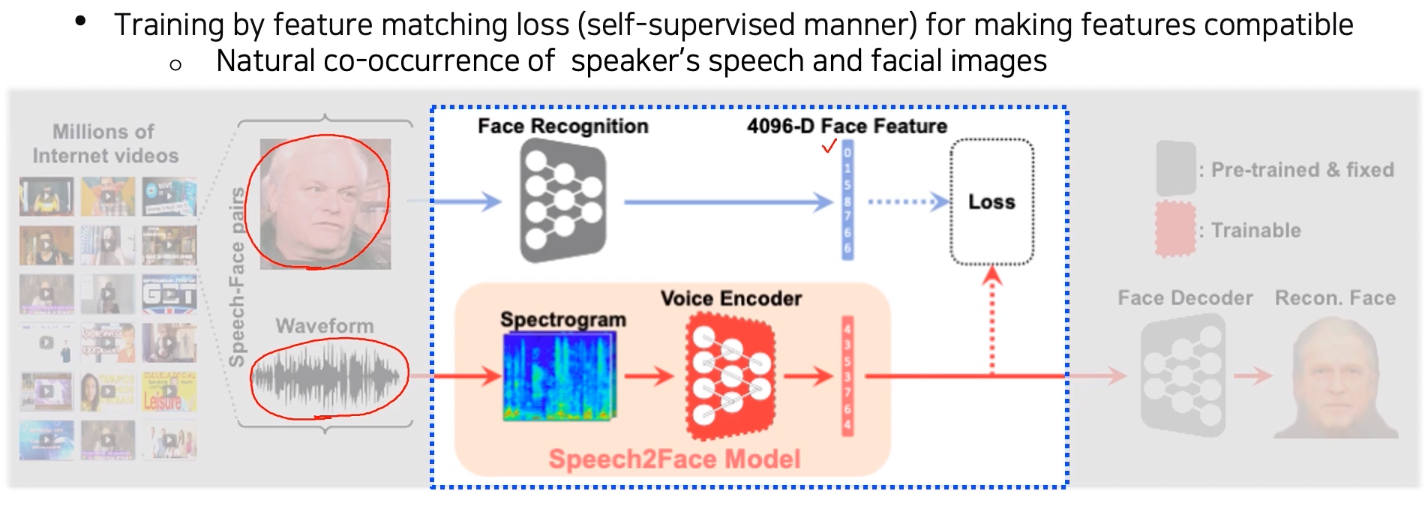
-
Video의 image에서는 face recognition network의 input으로 넣어 face feature를 추출
-
Video의 audio는 spectogram으로 만든 후 Speech2Face model을 통과시켜 feature vector를 추출 후 face feature를 따라하도록 teacher-student식 학습(joint embedding learning을 응용)
-
Annotation이 필요없는 self-supervised learning
--> Video에서 image-audio가 미리 pair라 annotate 됐다고 볼 수 있음
Image-to-speech synthesis
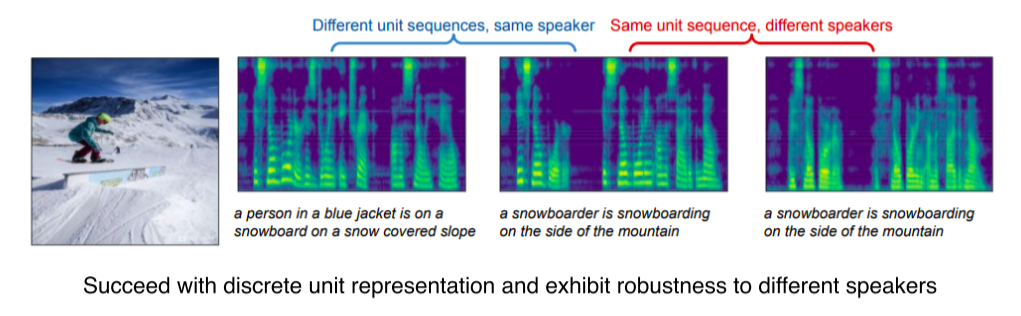
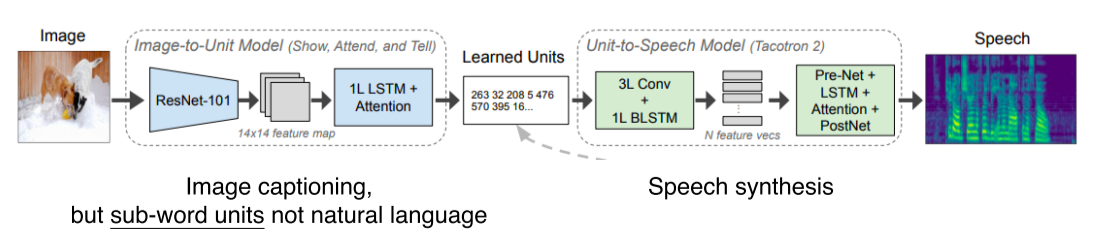
-
Image는 CNN으로 14x14 feature map, 이후 Image-to-Unit model로 sub-word unit을 추출(완전한 word가 아니라 token 형식에 더 가까움)
-
Unit-to-Speech model(TTS, text to speech)에서 sub-word unit을 speech로 출력
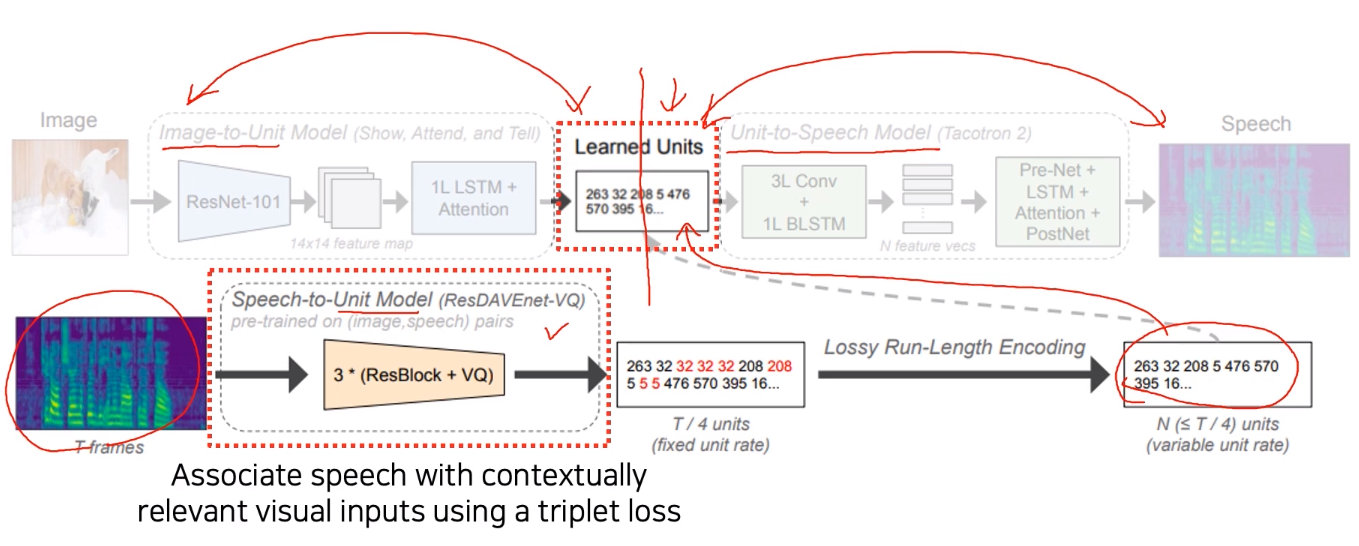
-
Speech-to-Unit에서 나온 unit을 model 중간의 learned unit에 넣어줌
-
Image-to-Unit, Unit-to-Speech model은 각각 image-unit, unit-speech를 기반으로 학습 --> end-to-end, 하지만 한번에 모두 train되는 것은 아님
Cross modal reasoning
Sound source localization
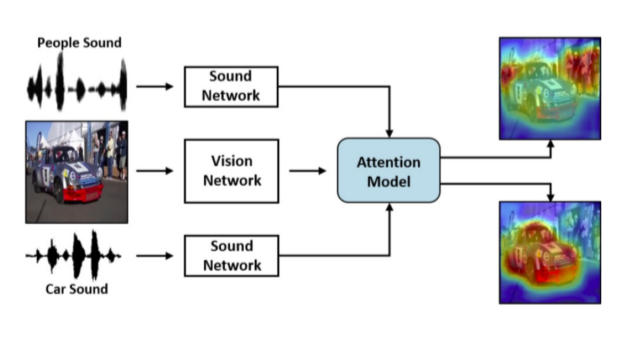
- Sound가 image의 어느 부분에서 발생하는 건지 알려주는 task
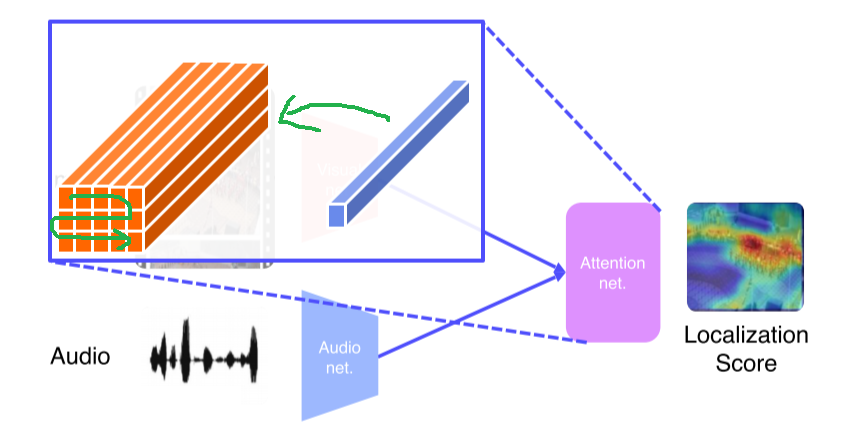
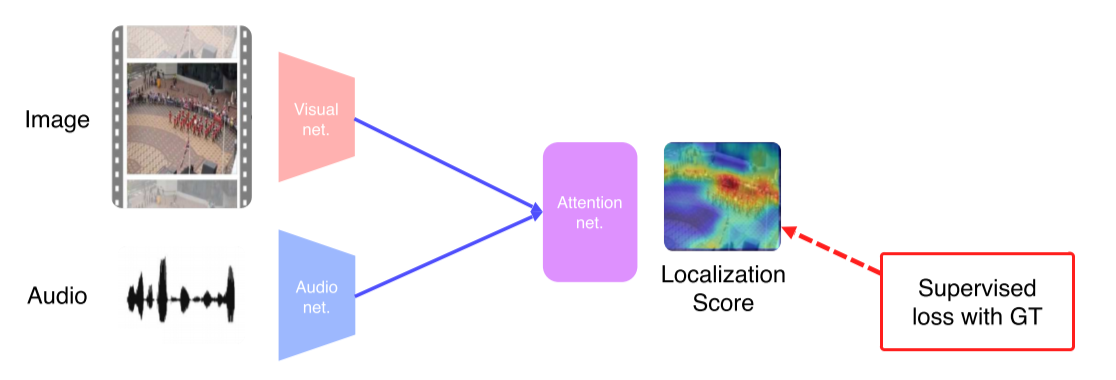
- Fully-Supervised: Ground truth가 있다면 KL-div, C-E, L-2 norm 등으로 학습
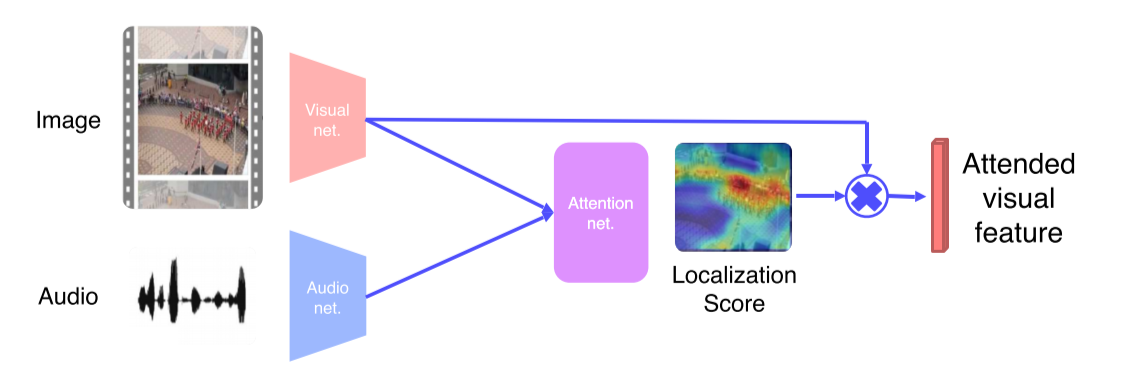
- Unsupervised: Image feature map과 localization score를 weighted sum pooling해서 image에 weight를 줌 --> 어느 부분이 중요한지 알 수 있음!
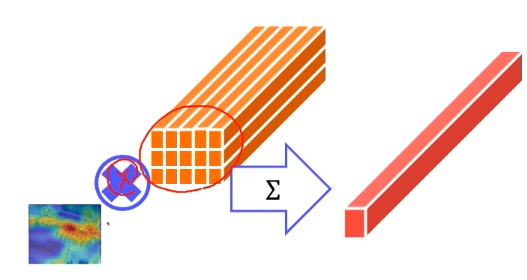
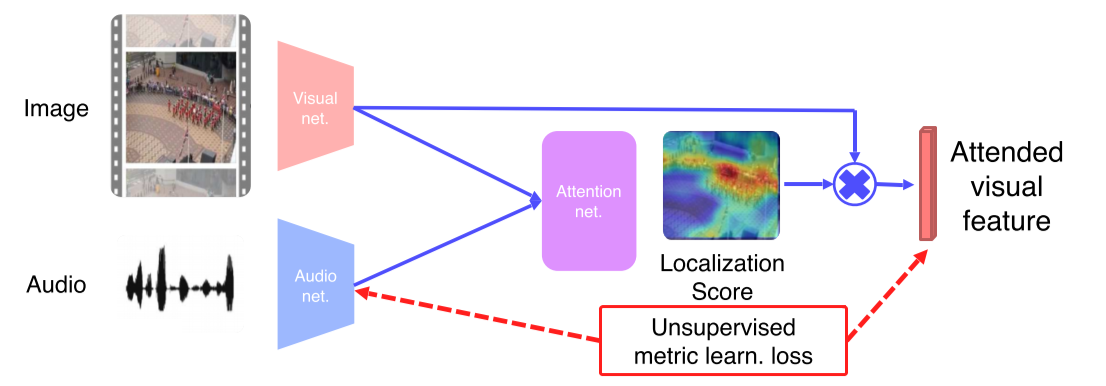
- 이후 sound feature와 attended visual feature를 metric learn해서 같은 video에서 나왔으면 같게, 다른 video에서 나왔으면 다르게 만듬
- Attended visual feature는 localization score에서 가장 weight가 높은 부분을 닮고있음, 이를 sound feature와 train해서 한 video에서 나왔다고 model에게 알려줌
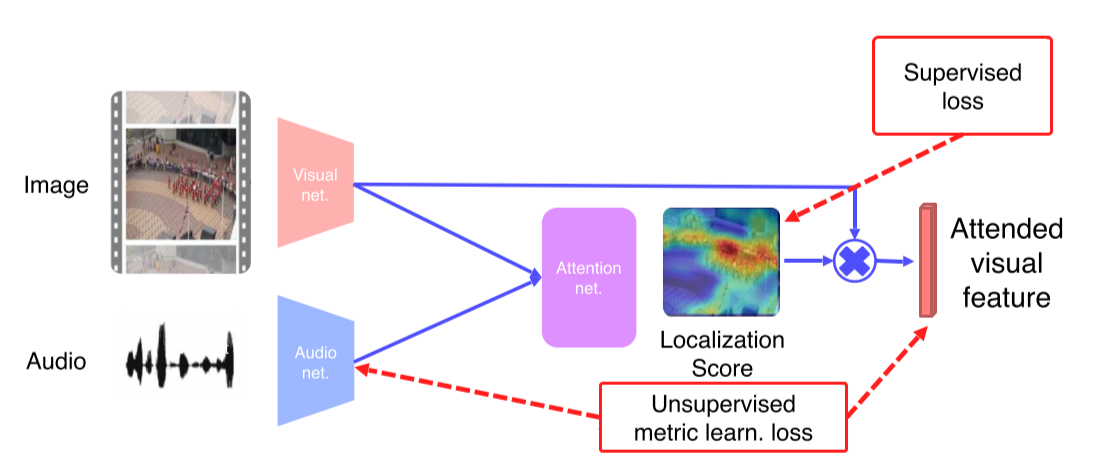
- 2개 다 쓰면 semi-supervised learning
Looking to listen at the cocktail party
칵테일 파티 효과(cocktail party effect): 파티의 참석자들이 시끄러운 주변 소음이 있는 방에 있음에도 불구하고 대화자와의 이야기를 선택적으로 집중하여 잘 받아들이는 현상에서 유래한 말
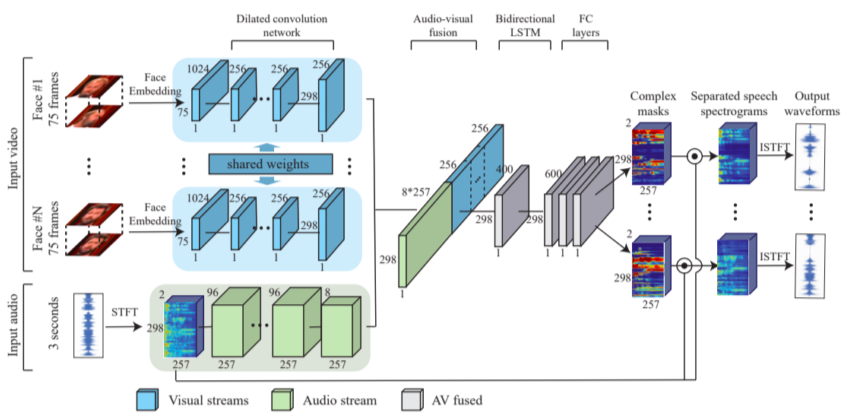
- 크게 visual stream, audio stream, fusion, output seperation의 4가지 부분으로 나뉨
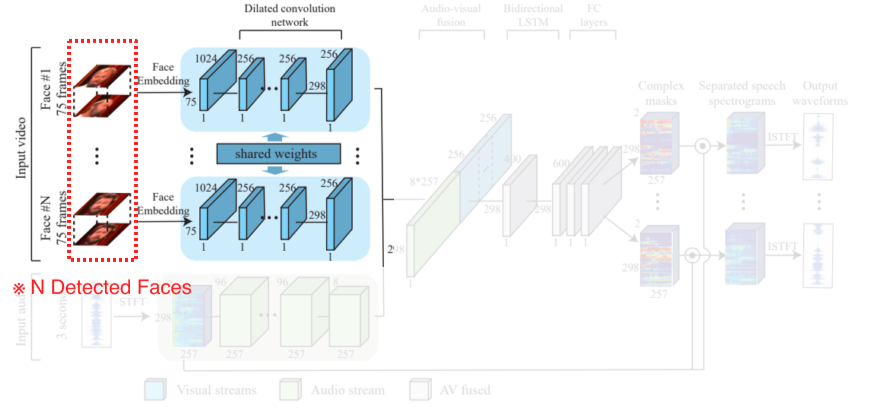
- 각각의 얼굴을 face embedding을 해서 feature vector로 출력
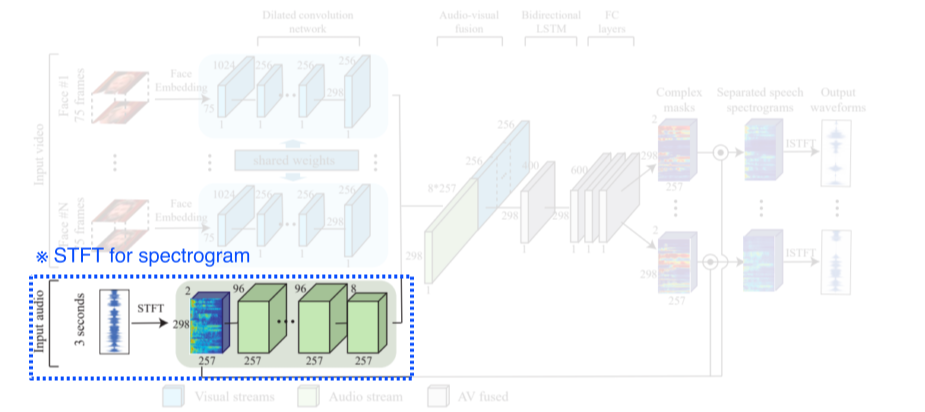
- Spectogram으로 speech에 대한 정보를 추출
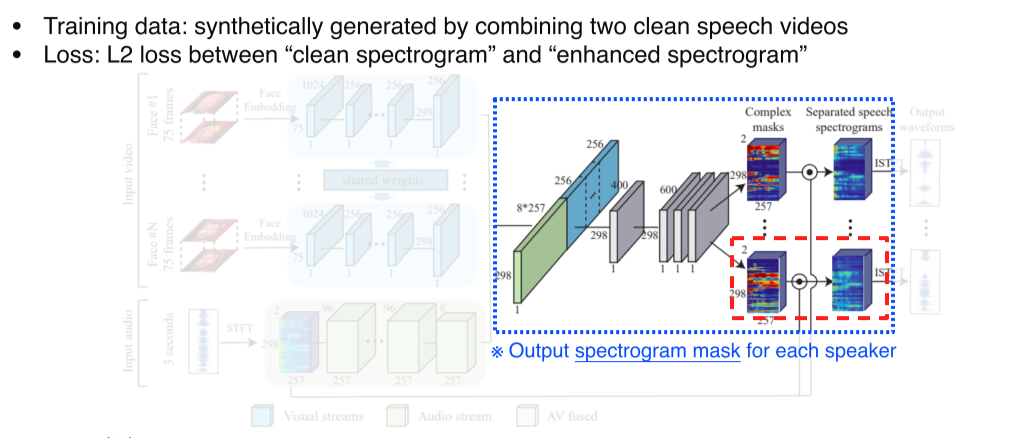
-
Face feature, speech feature를 concat 후 complex mask 형태로 출력
-
Complex mask는 각각 spectogram을 어떻게 분리할지 결정하기 때문에, complex mask와 spectogram을 곱해서 seperated speech spectogram을 출력 후 다시 waveform으로 복원
-
Metric은 L2 loss를 사용
--> Clean spectogram(ground truth)가 있어야한다는 의미
--> Train data는 2개의 clean speech data를 합성(synthetically generate)해서 사용
Reference
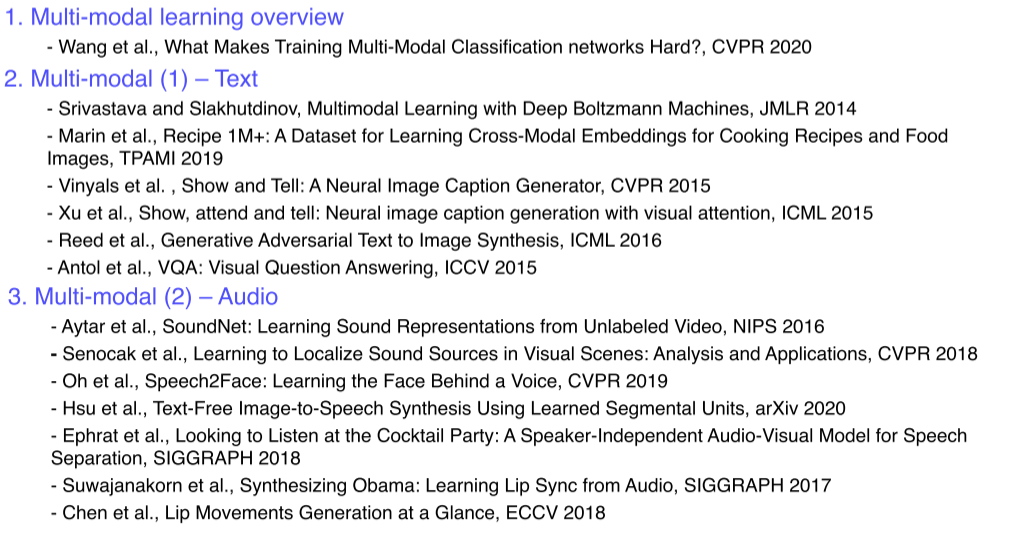
Further study
(1) Multi-modal learning에서 feature 사이의 semantic을 유지하기 위해 어떤 학습 방법을 사용했나요?
(2) Captioning task를 풀 때, attention이 어떻게 사용될 수 있었나요?
(3) Sound source localization task를 풀 때, audio 정보는 어떻게 활용되었나요?