Summary
- 깊이 증가시키며(16-19 Layers) 성능 향상
- 모든 Conv LAYER에서 3x3 filter 사용 → 파라미터수 줄여 연산량 감소
Abstract
- Depth로 Accuracy를 향상시키는 방법
- 최소 크기의 Filter(3x3)을 사용하면서 Depth를 증가시켜 성능 향상
Introduction
- Conv Net 효과 발전, 성능 향상 위한 다양한 시도 있었다.
- 본 논문은 구조 설계 시 Depth에 집중
- 더 많은 Conv Layer를 추가했다
- 이는 모든 Layer에 3x3 Filter를 사용했기에 가능
- 다양한 부분에서 뛰어난 결과 얻었다
ConvNet Configurations
Architecture
- Input = 224x224 RGB Image, 전처리 시 각 픽셀마다 RGV Value 평균 뺌.
- Very small Receptive Field가지는 Filter인 3x3 사용
- 이는 상하좌우, 가운데 개념을 가진 Smallest Size이다
- 비선형성 위해 1x1 Filter도 사용
- Stride =1 고정
- Spatial Resoluition 유지하기 위해 Padding =1
- Max Pooling = 2x2, stride 2
- FC Layers는 3개
- 처음과 두번째는 4096 Channels, 세번째는 1000 Channels 가진 Softmax Layer
- Activation Function은 ReLU 사용
- AlexNet에 적용한 LRN은 사용안함
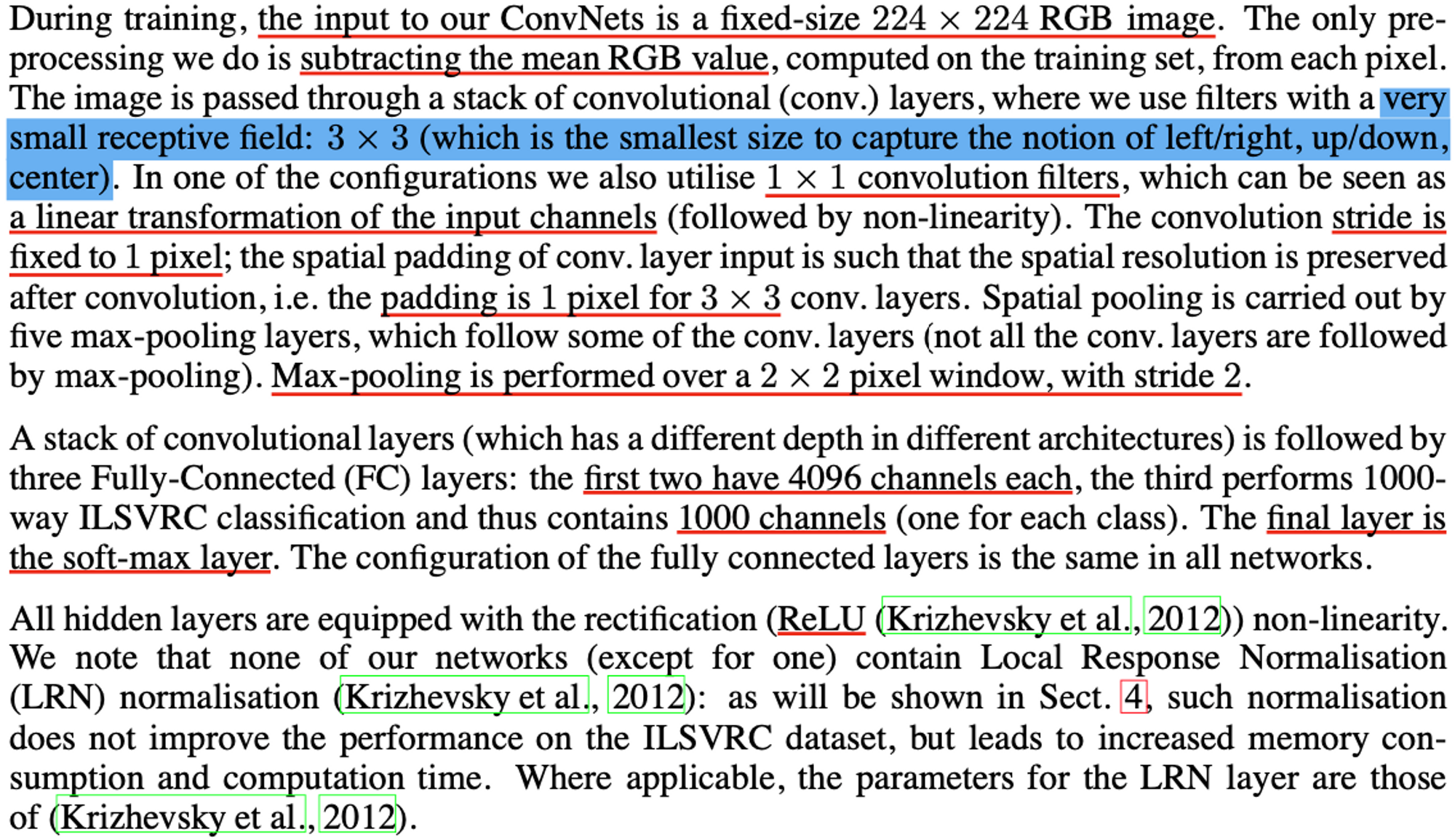
Configurations
- 11 Layers(8 conv, 3 FC) 부터 19 Layers (16 conv, 3 FC)
- Width(Channels)는 64에서 시작해서 512까지 증가
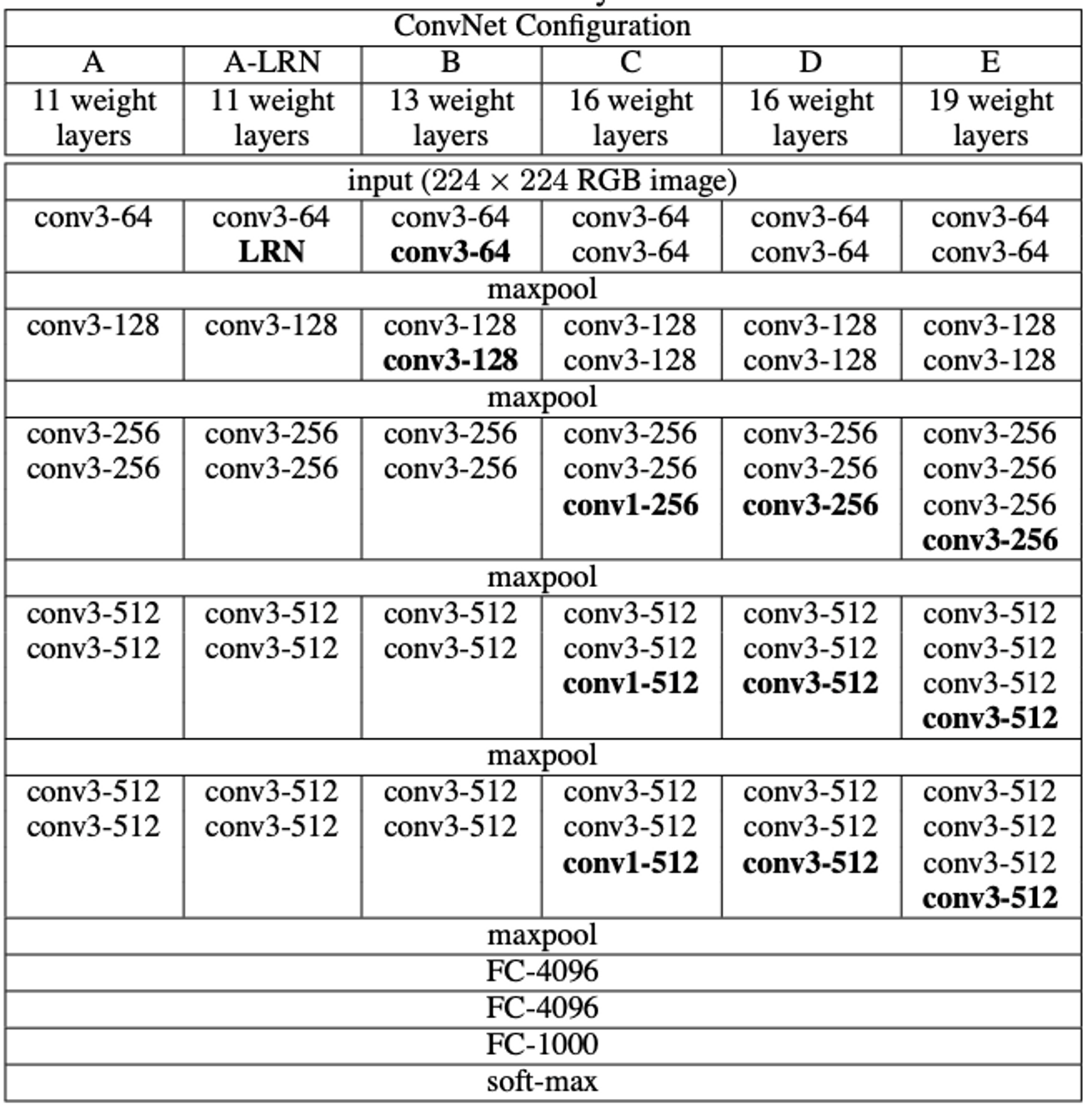

Discussion
- 전체에 Stride 1 ,3x3 Filter
- 3x3 2개 → 5x5, 3x3 3개 → 7x7 ⇒ Parameters수 줄여
- ex) 3(32C2)=27C2, 72C2=49C2
- 1x1 Conv Layer는 non-linearity를 증가
- 1x1은 Linear Projection이지만 ReLU로 인해 비선형성 부여
- GoogleNet과 비교하면, 깊은 구조에 작은 filter를 사용한 것 유사하지만
VGG보다 복잡하고 첫번째 Layer 이후 Feature Map의 Spatial Resolution이 급격하게 줄어든다.
Classfication Framework
Training
- mini-batch gradient descent
- batch size = 256, momentum = 0.9
- weight decay (L2 penalty) = 0.0005
- dropout (FC layer 1,2)
- learning rate = 0.01로 초기화, Valid 향상 없으면 101감소, 총 3번 감소
- epochs = 74 (370K iter)
- pre-initialised
- 나쁜 초기화는 성능 저하 야기 → 구조 A를 shallow enough하게 학습시켜서 이를 첫 4개 Conv Layer와 마지막 3개 FC Layer의 초기화에 사용
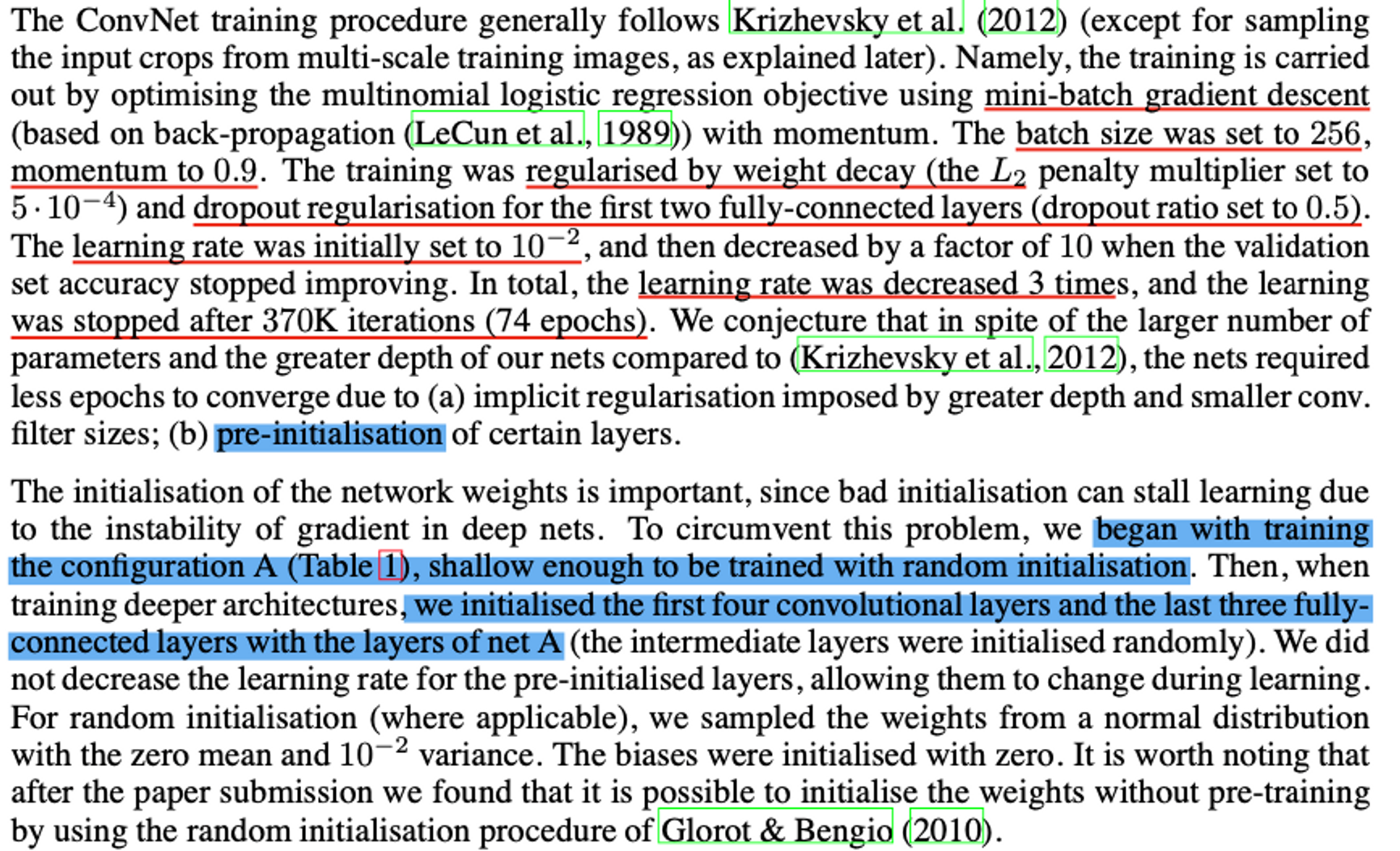
Training image size
- crop된 이미지를 무작위로 수평 뒤집기
- 무작위로 RGB 값 변경하기
- image rescaling
- single-scale training
- Multi-scale Training
- 256 to 512
- Scale Jittering
- wide range of scales에 있는 Object 판별 가능
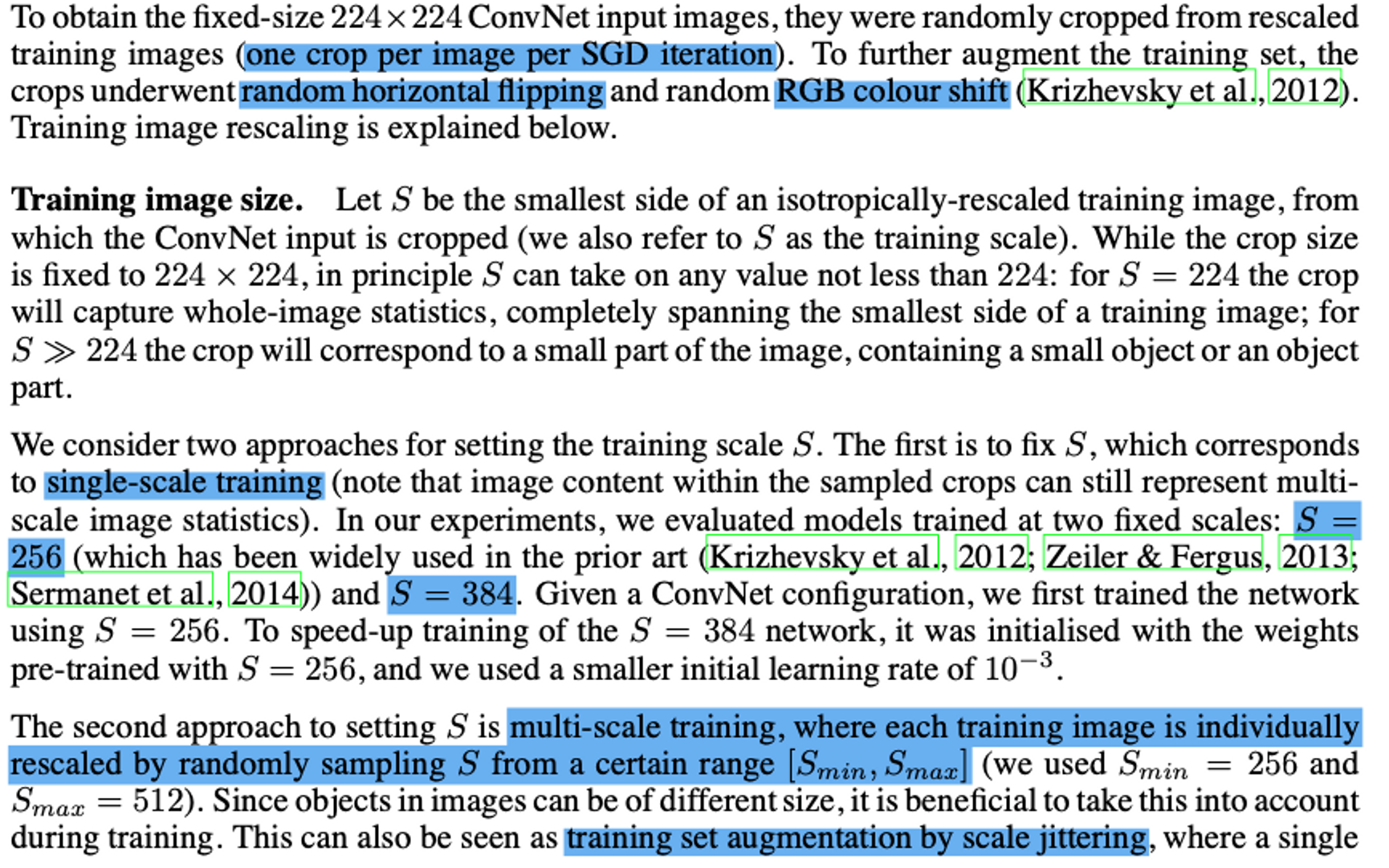
Testing
- Q로 Rescale
- Fully Connected Layer을 conv layer로 변환
- 첫 FC layer는 7x7 conv layer, 마지막 두 FC layer는 1x1 conv layer로 변환
CLASSIFICATION EXPERIMENTS
4.1 Single Scale
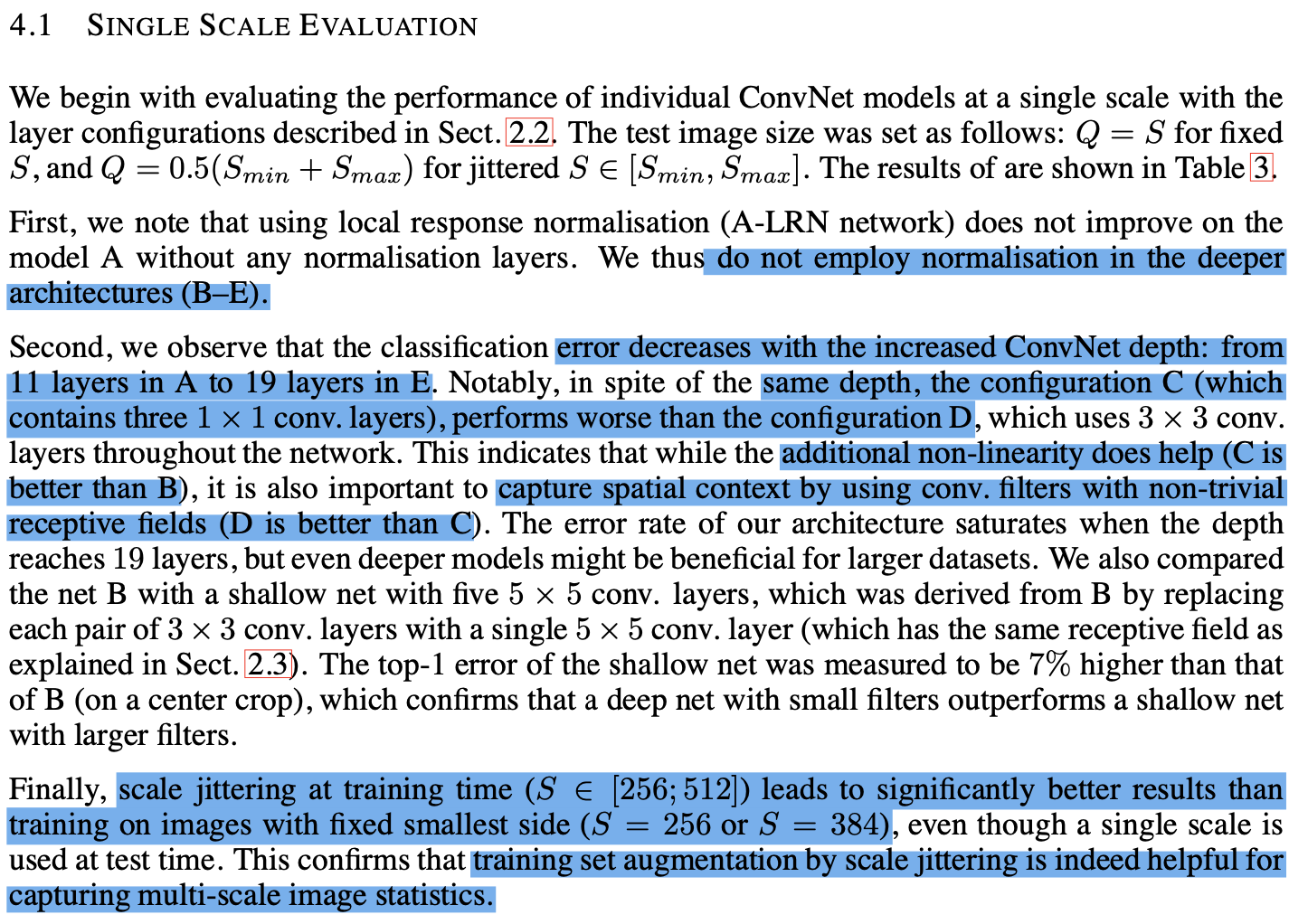
4.2 Multi-Scale
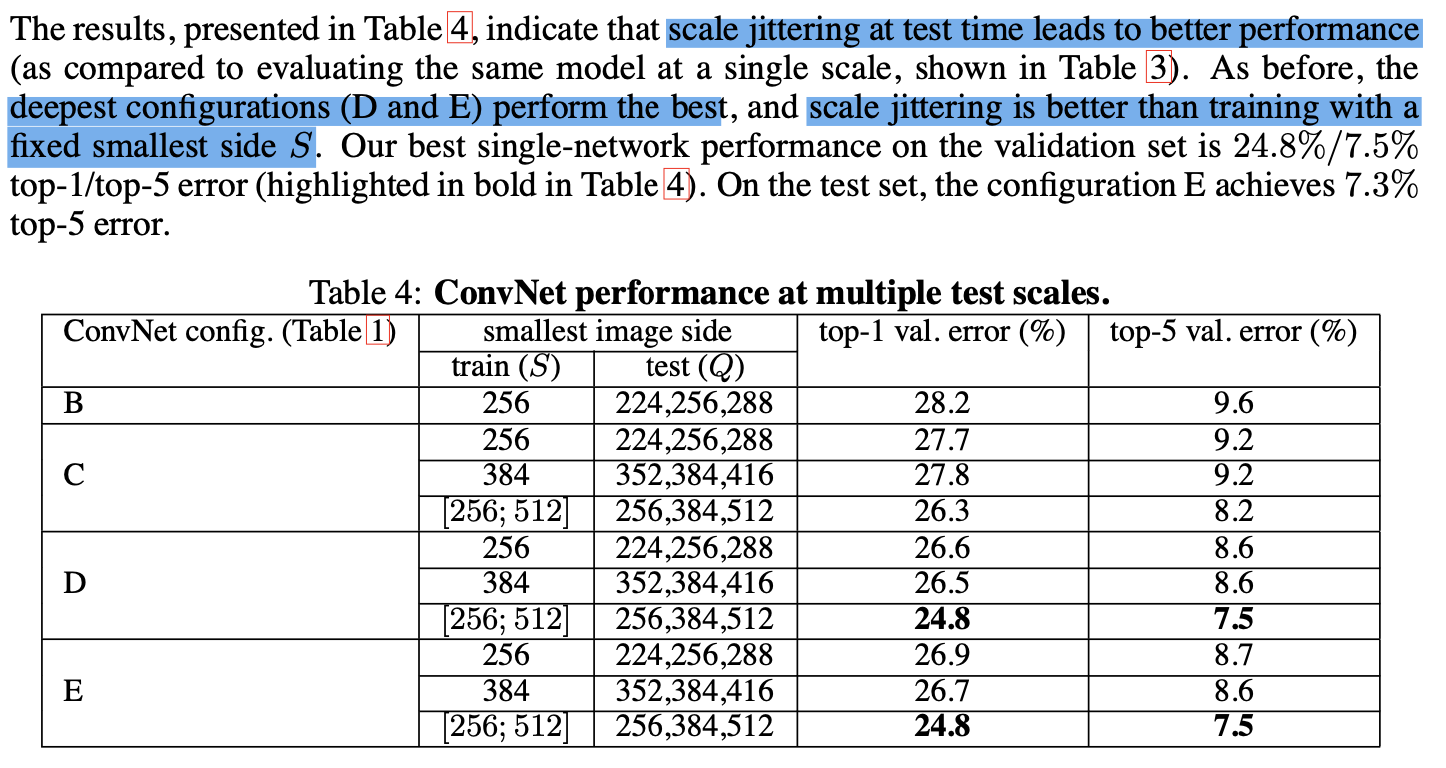
Conclusion
depth is beneficial for the classification accuracy
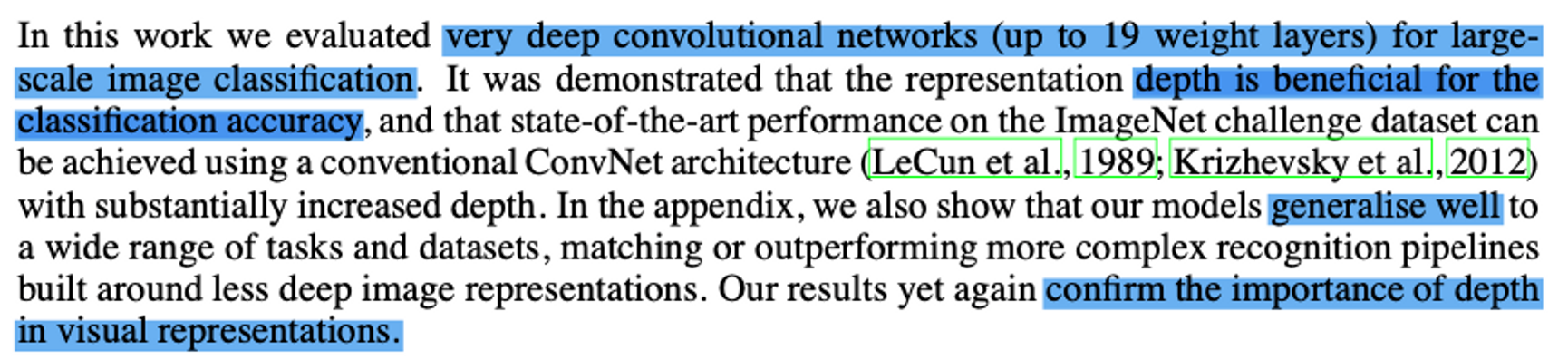