Large Language Model Can Interpret Latent Space of Sequential Recommender
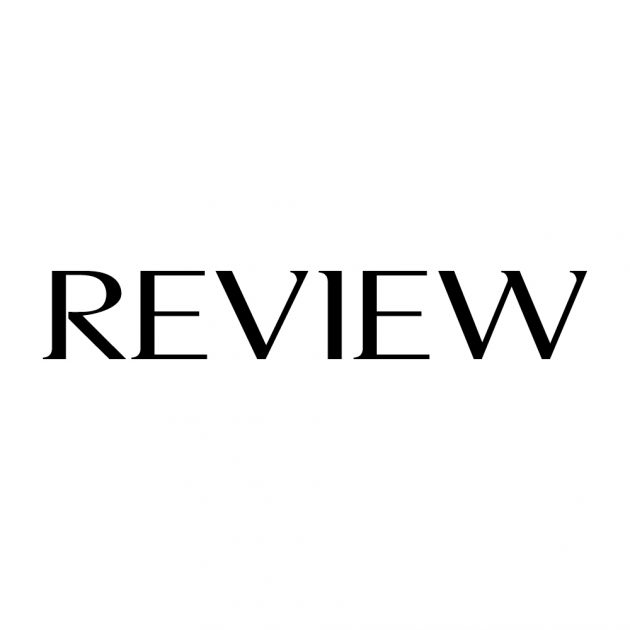
INTRODUCTION
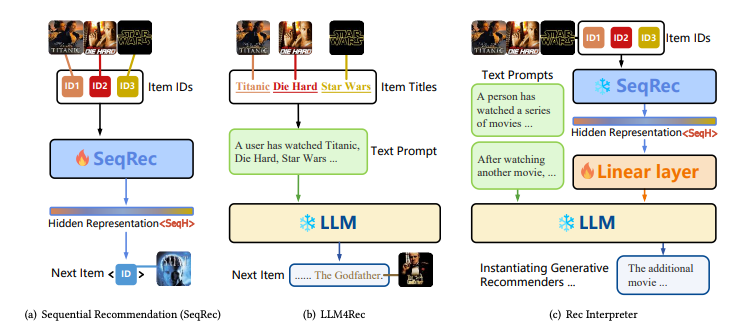
- 추천 이력을 바탕으로 next item을 prediction하는 sequential recommendation은 fundamental task
- 일반적으로, 식별 가능한 ID를 부여 후 hidden embedding sequence를 바탕으로 representation을 학습해 추천하는 method
- LLM은 여전히 여러 task를 dominate하며, 최근에는 여러 modalities를 잘 반영하는 걸 보여줌
- ViT, HiFiGAN 등을 통해 얻은 hidden state를 token embedding으로 사용하는 방식
- 현재 LLM4Rec은 text prompt를 사용해 한번에 LLM을 통과시키는 것으로, 그 한계에 직면해 정체되어 있음
- LLM이 recommender의 representation을 이해할 수 있을까? 라는 물음에서 우리는 RecInterpreter를 제안
- LLaMa와 GRU4Rec, SASRec등을 통해 두 모달리티를 통합하려 함
- Lightweight adapter를 통해 representation을 LLM으로 align
* 이 token을 text token사이에 삽입하여 prompting
- adapter를 training 할 때에는, 다른 두 모달의 weight는 frozen
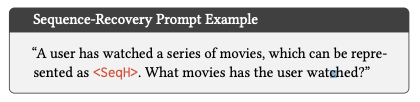
- recommendation specific token을 갖기 위해, 우리는 sequence recovery token을 제안
- empirical하게 LLaMA는 sequence hidden state를 이해할 수 있지만, 너무 compressive하기에 limitation이 존재
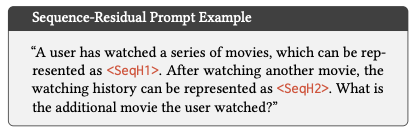
- 우리는 기존 시청 기록과, 추가적으로 상호작용한 뒤 각각의 representation을 다음과 같은 prompt를 통해 LLM의 이해를 도움
- 실험적으로, 이 method는 매우 효과적이며, 대상 아이템의 text description까지 이해함
- 또한 linear projection layer만이 tunable하기에 여러 platform에서 adaptation하기에도 용이
- 또한 generative recommender의 hidden state는 이산적으로 설명이 힘들지만, 우리는 RecInterpreter는 surrogate하게 설명할 수 있음
INSPIRE LLMS TO UNDERSTAND SEQUENTIAL RECOMMENDERS
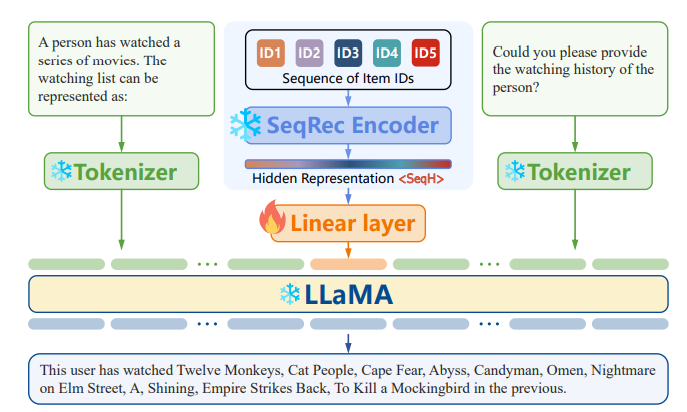
- Framework 소개
- Sequence Recovery Prompt와 Sequence Residual prompt
Sequence-Recovery Prompting
- 이전 multi-modal LLM 연구에서 motivated
- LLM이 추천 hidden state를 바탕으로 item을 text로 reconstruct하도록 objective 구성
Sequence Encoding via Sequential Recommenders
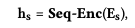
- Es : 상호작용한 item 목록의 embedding을 concat
- SASRec 등 을 사용해 hidden state로 인코딩
Representation Adaptation vis Lightweight Adapter

- LLM의 token size (ex: LLaMA는 4096)으로 dimension mapping layer
- 이 hidden state token은 textual content와 user interaction을 둘 다 보유
Prompt Design for the Adapter Training
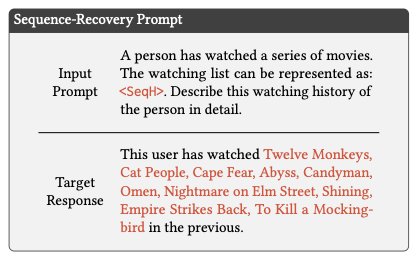
- Input과 Output을 다음과 같이 설계
- 중요한 것은 프롬프트가 두 가지 component를 보함한다는 것
- Input prompt는 projected된 hidden state를 포함
- Output prompt는 Auto Regressive하게 hidden state의 text description을 reconstruct
Sequence-Residual Prompting
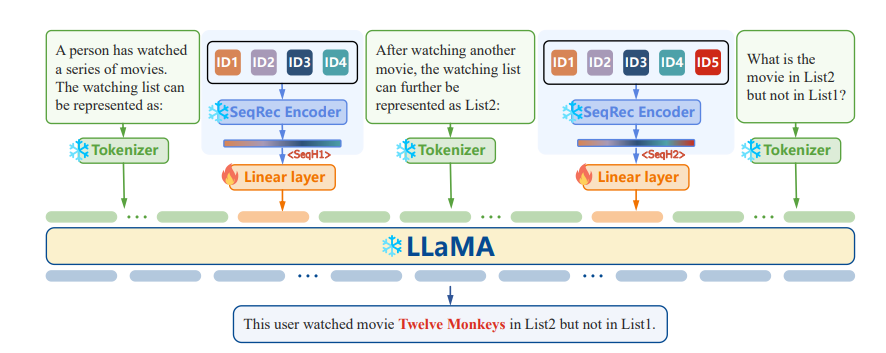
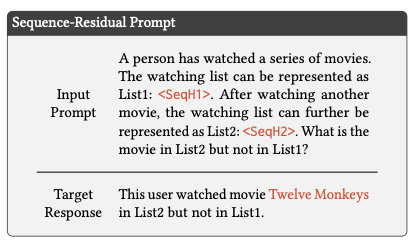
- Recommendation은 일반적으로 매우 sparse, 따라서 representation을 통해 모든 것을 이해하는 건 limitation이 존재
- Flamingo는 두 비슷한 이미지를 제시하고, 차이를 동시에 제공하여 LLM의 visual modalitie를 강화한 논문
- 비슷하게, 우리는 LLaMA가 sequence의 residual(잔여) item을 더 잘 식별하도록 하는 Prompt를 제안
- 두 hidden state를 project한 뒤, figure와 같이 prompt를 구성
- LLaMa가 이전 hidden state와 다음 hidden state를 비교하여 추가적으로 어떤 Item을 interaction 했는지 이해하도록 함
Instatntiate Oracle Items
- 위의 training step으로 generative recommender에서 어떻게 작용하는지 확인
DreamRec
- DreamRec으로 generation하는 item은 hidden vector이기에, nearest item을 추출해야 함, 따라서 후보군으로 제한될 수 밖에 없음
- 우리는 DreamRec으로 Oracle item의 description을 생성할 수 있기에, explicit한 추천이 가능
Construct Sequence-Residual Task with Oracle Item
- Sequence residual prompting의 input으로 기존 sequence와 DreamRec으로 생성한 아이템이 포함된 sequence를 동시에 입력
- LLaMA는 oracle item의 textual description을 두 hidden state를 비교해 제시할 것
Training and Inference
- Training시에는, 기존과 동일하게 GT sequence를 유지하며 prompting
- Inference 때, 앞서 설명한 방법으로 oracle item의 text description을 획득 (이는 DreamRec에서 불가능 했던 것)
EXPERIMENT
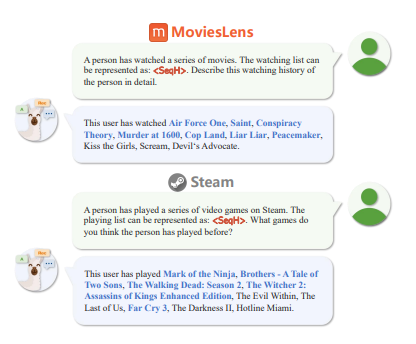
Experimental Settings
- LLM의 Tuning은 매우 time-consuming, 따라서 ML100K와 STEAM dataset사용
- Recommendation Backbone으로 GRU4Rec, Caser, SASRec, DreamRec 사용
Sequence-Recovery Result
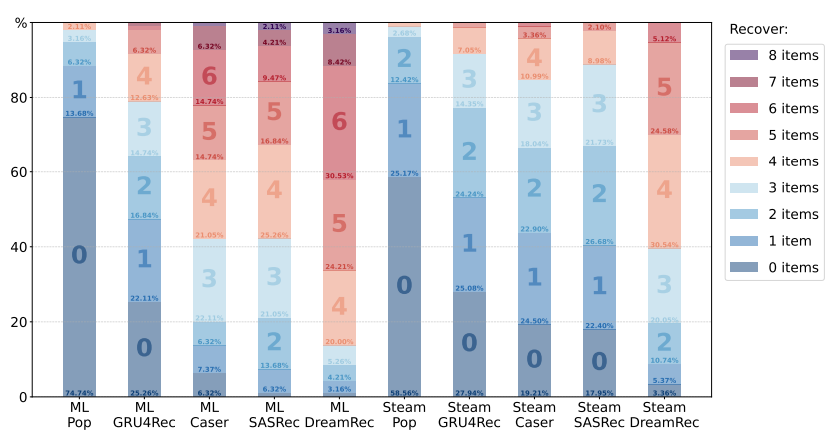
- 성능을 확인하기 위한 가장 간단한 방법은, Encoding된 항목을 text description과 함께 복구하는 것
- 이는 매우 어려운 작업임을 명심해야 함, 각 데이터 셋에는 수 천개의 항목이 포함되어 있으며 hidden state는 매우 compressive하기에
- Popular와 비교하여, 우리의 LLaMA는 sequential recommender의 representation을 이해하는 능력을 보여줌
- 특히 LLaMA는 ML 데이터 셋에서 더 나은 복원력을 보이는데, 이는 Steam dataset의 게임 description이 더 복잡하고 noisy하기 때문인 것으로 보임
- 대체적으로, DreamRec이 가장 높은 성능
Sequence-Residual Result
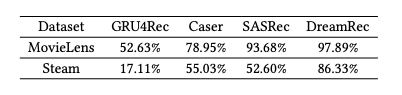
- table은 sequence-residual prompting의 결과
- LLaMA는 DreamRec과 가장 좋은 Interaction을 보임
- 여전히 Steam에 비해 ML-100k에서 더 좋은 성능을 보이지만, DreamRec을 사용하면 Steam에서도 준수한 성능
- 이는 DreamRec이 학습한 representation이 복잡한 text description을 더 잘 파악하는 것으로 볼 수 있음
Instantiate Oracle items
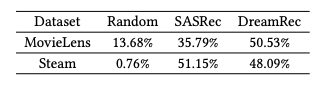
- 앞서 말한 방법을 토대로 DreamRec을 통한 Inference를 통해 추천 결과로 instatantiate할 수 있음
- 다만 DreamRec의 예시와 비슷하게, candidate를 벗어나는 instance가 실험적으로 다수 발견 됨
- 따라서 Hit ratio, NDCG와 같은 metric이 아닌 ChatGPT를 통해 evaluation을 진행
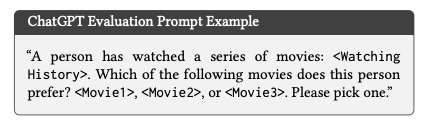
- 다음 프롬프트를 통해 SASRec, DreamRec, random sample 중 어떤 것을 GPT가 고를지 평가
- SASRec, DreamRec이 naive recommendation을 dominate