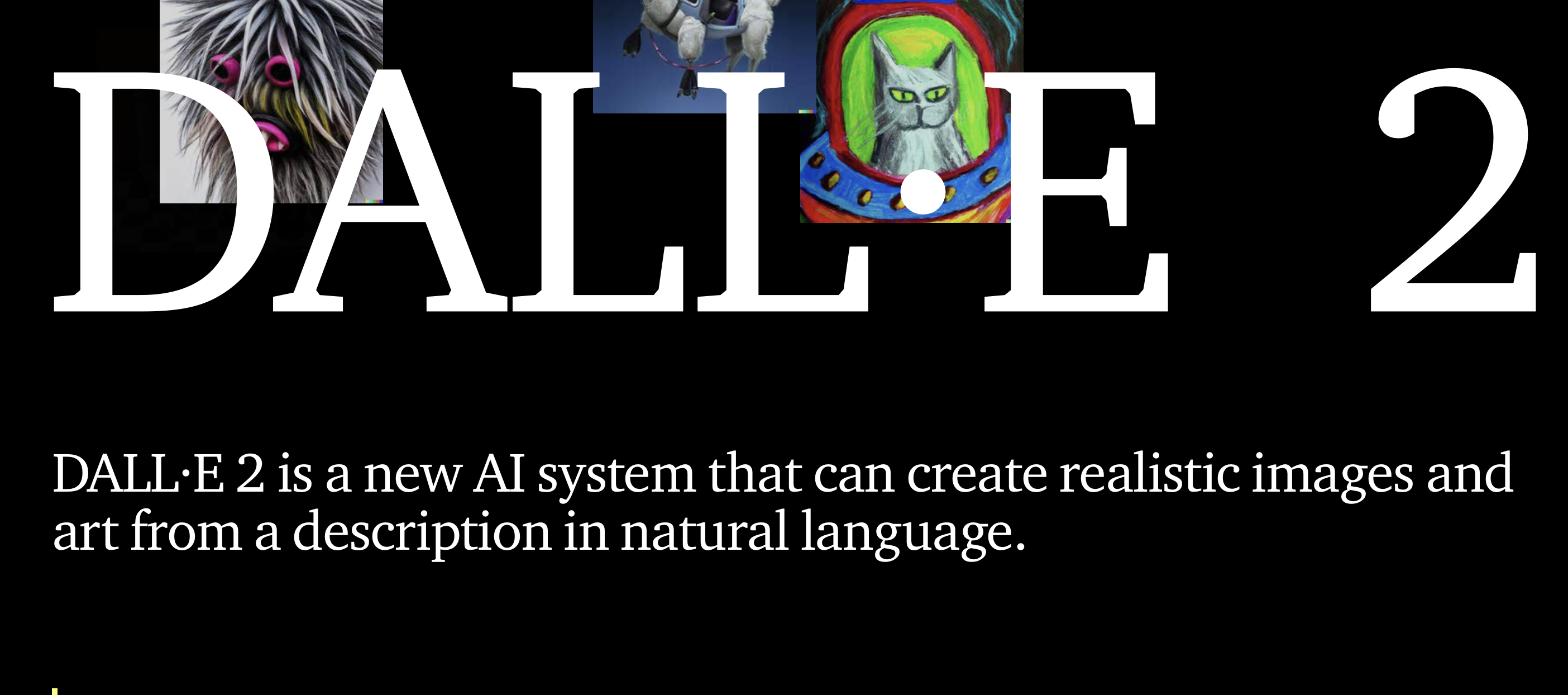
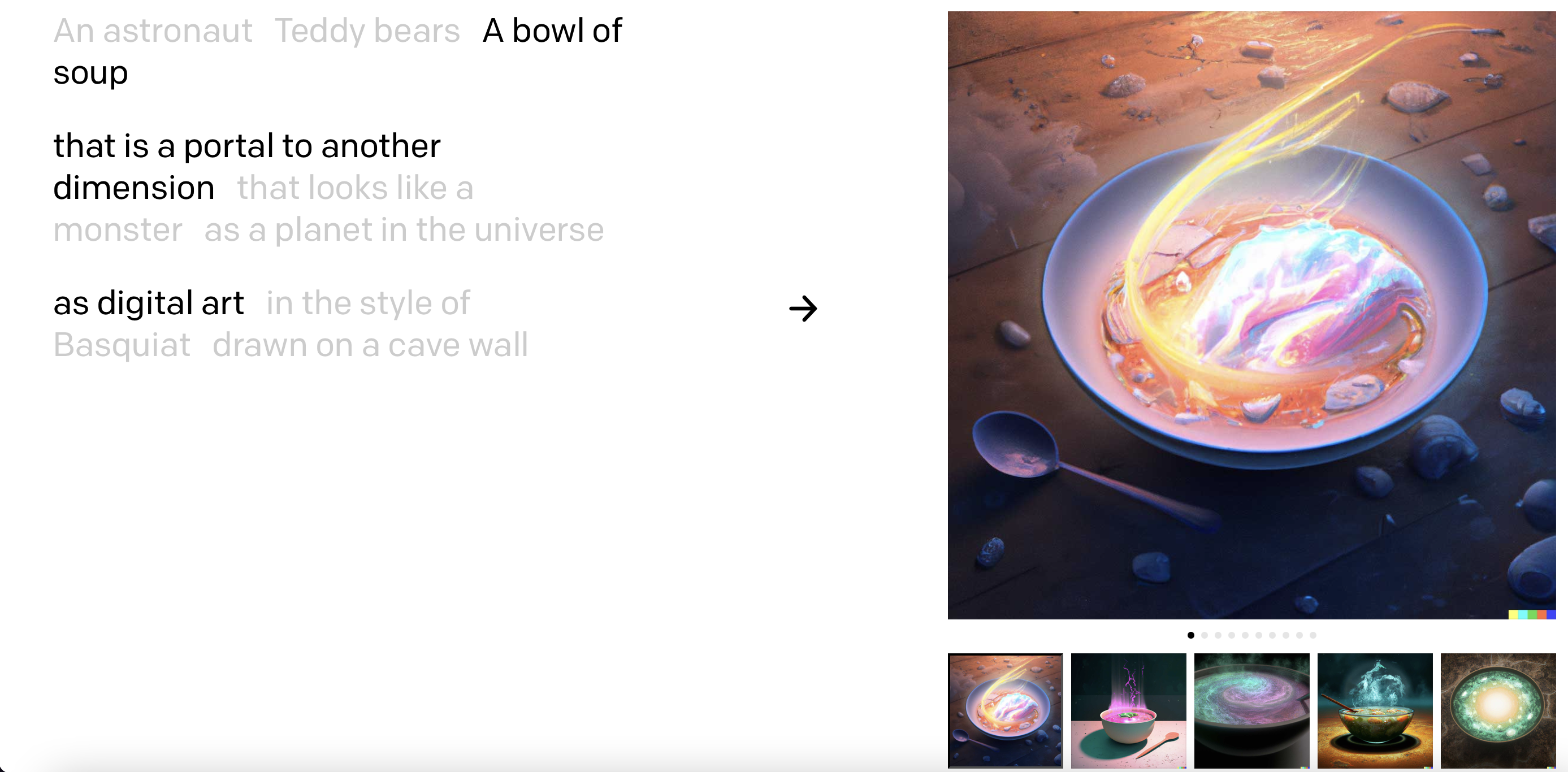
- CLIP같은 Contrastive 모델들은 이미지로부터 Robust representation을 학습할 수 있다고 알려져있음.
- 저자들은 이렇게 학습한 representation을 이용해 Image Generation을 수행하는 2-stage model을 제안함
- Stage 1 : image embedding을 생성하는 prior network
- Stage 2 : image embedding으로부터 image를 생성하는 decoder network
- prior는 주어진 텍스트를 기반으로 image embedding을 생성하는 방법을 학습하며, Diffusion network와 Autoregressive network로 이루어져있음(트랜스포머겠죠).
- decoder는 image embedding으로부터
- image embedding과 image를 생성하는데에는 stochastic하므로 샘플링해서 다양한 임베딩, 다양항 이미지를 얻어낼 수 있음.
- 특히, image represenation을 직접적으로 생성하는 방식이 이미지의 다양성을 높히는데 큰 도움을 줌(사실성, 텍스트 캡션과의 유사성 등도 훌륭하게 유지하며)
- ::본래 딥러닝 모델들은 End-to-End 방식으로 학습을 한 뒤, 그로부터 간접적으로 학습된 feature(representation)을 이용하는 반면, 저자들의 방법은 represenation(embedding)자체를 생성하는 방법을 배우기에 보다 유리하다는 것으로 해석하면 될듯::
- 특히 저자들의 decoder는 image embedding에 없는 정보(non-essential details)조차 다양하게 변화시켜 의미적으로, 스타일적으로 의미있는 이미지들을 생성해낼 수 있음
- 최근 딥러닝 트렌드는 "더 큰 모델, 더 많은 데이터셋을 갖추자"라고 봐도 무방함.
- 이런 관점에서 CLIP은 image represenation을 학습해내는데 성공적인 모델임(SoTA로 봐도 무방).
- 이와 동시에 Diffusion Network는 Image/Video 관련 Generative Model 분야에서 SoTA를 보이는 좋은 생성 모델임.
- 쓰이는 guidance technique이 sample fidelity를 높히는데 도움을 줌.
- 그래서 저자들은 Diffusion model을 decoder로 사용해, CLIP image encoder를 복원하는 식으로 학습을 함(즉, encoder의 approximate inverse가 decoder).
- 이런 CLIP-diffusion inversion 방식을 통해 여러가지 Image generation task를 수행할 수 있음.
- Non-deterministic 방식으로 decoder 모델이 학습되기 때문에 다양한 이미지를 다양한 방식으로 만들어내고 조작할 수 있다 !(심지어 학습하지 않았던 이미지까지 + 고퀄리티로).
아래는 본 연구에서 수행한 image manipulation.
Semantically similar output images
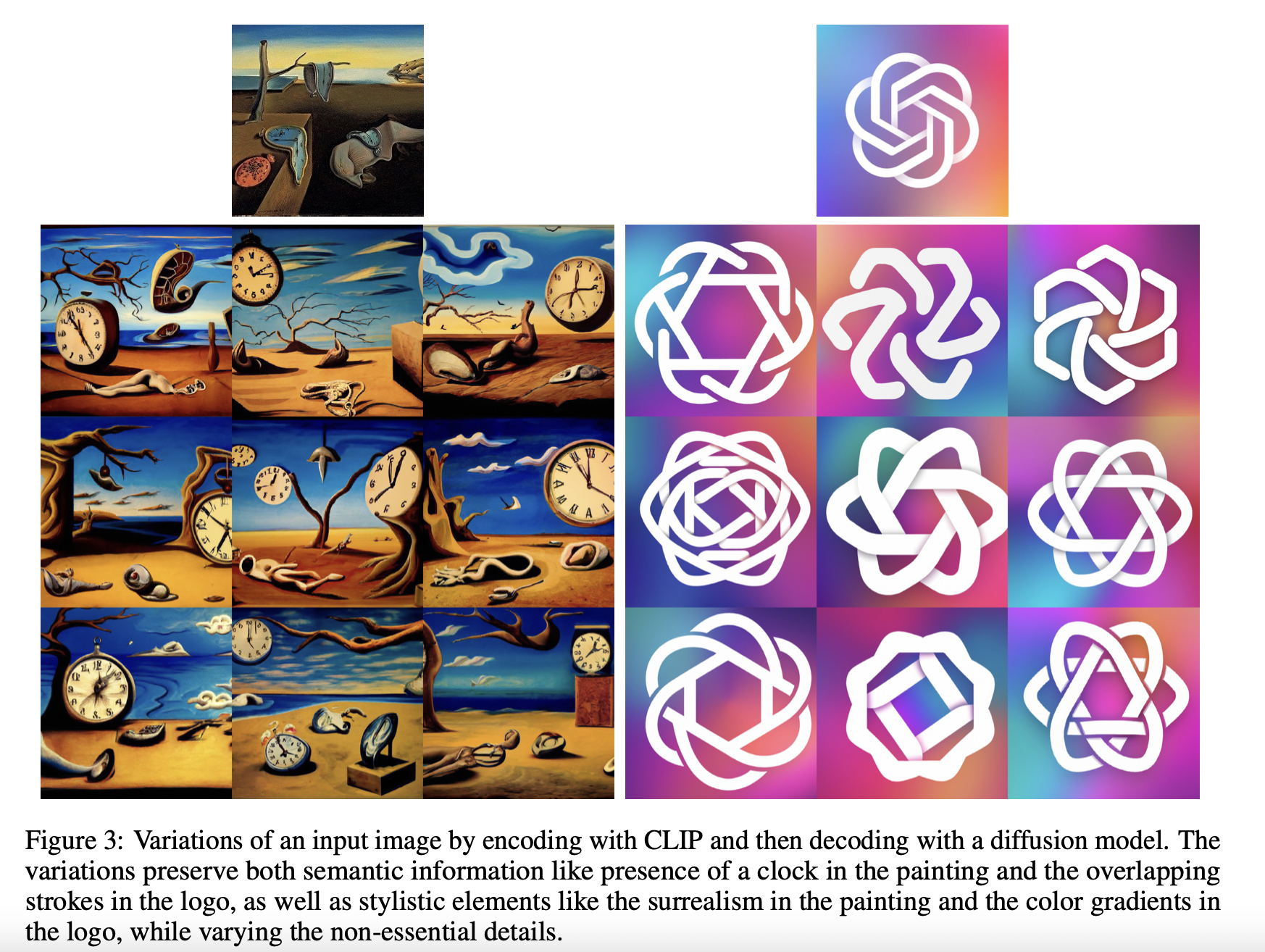
- 의미적으로 중요한 요소(시계, 흘러내림, 얽혀있음)를 놓치지 않고 이미지를 생성할 수 있다.
Interpolations of image embeddings
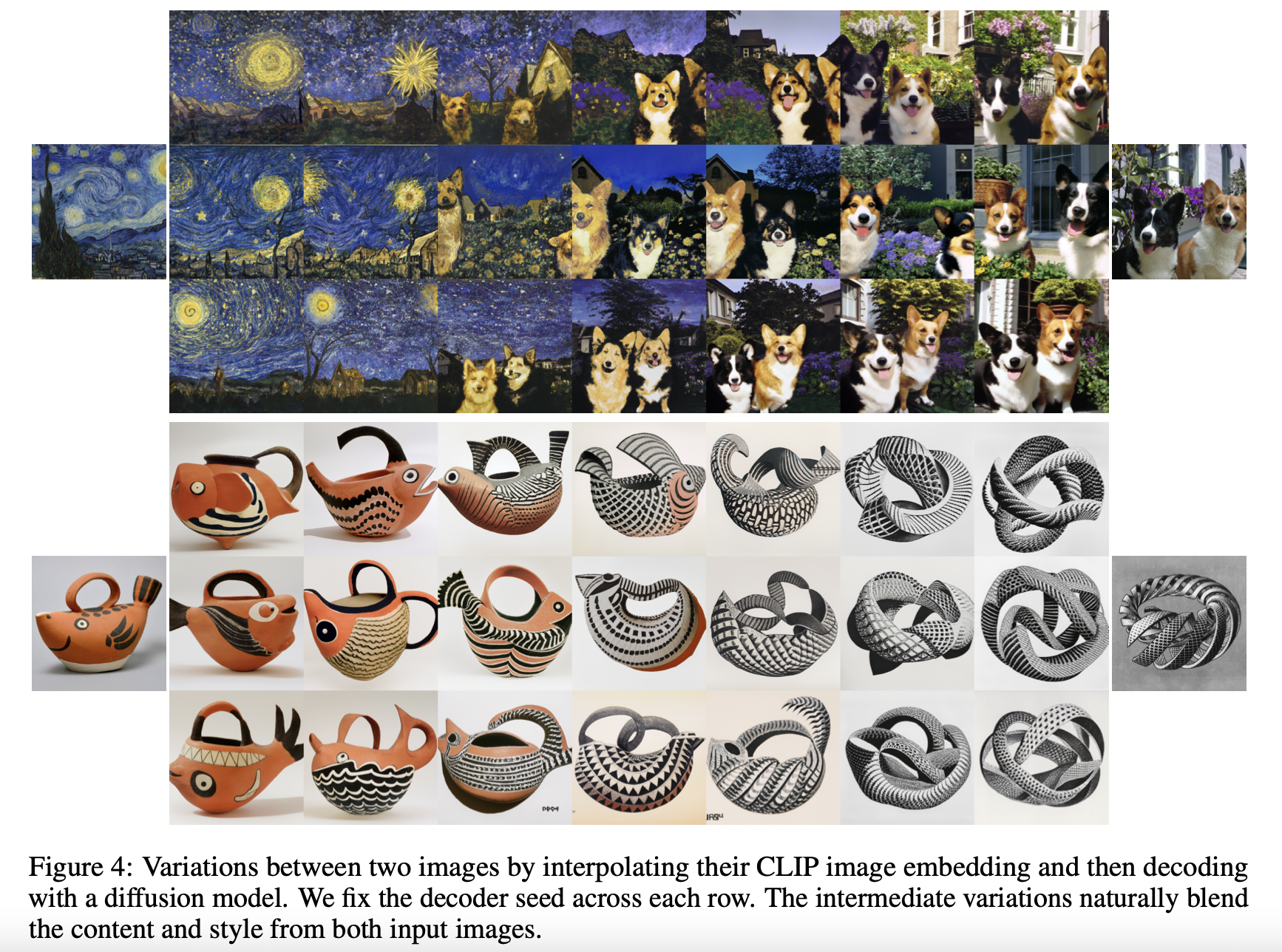
- 역시 인간의 직관성과 어울리는, (semantic content/style이 유지되는)좋은 manifold space에서 보간을 수행할 수 있다.
여기까지 GAN latent space 방식으로도 할 수 있는 태스크들이다(성능 차이는 고사하고).
하지만 GAN 방식이 CLIP latent space에 비해 부족한 점이 있는데, 바로 우리가 원하는 방향으로 text vector를 조작해서 image까지 수정하는 task를 잘 못한다는 것.
즉, CLIP latent space를 모델링한다면 무수한 체리피킹을 없이도 텍스트를 통해 인간이 원하는대로 image를 수정할 수 있음(좋음)
modify images by moving in the direction of any encoded text vector
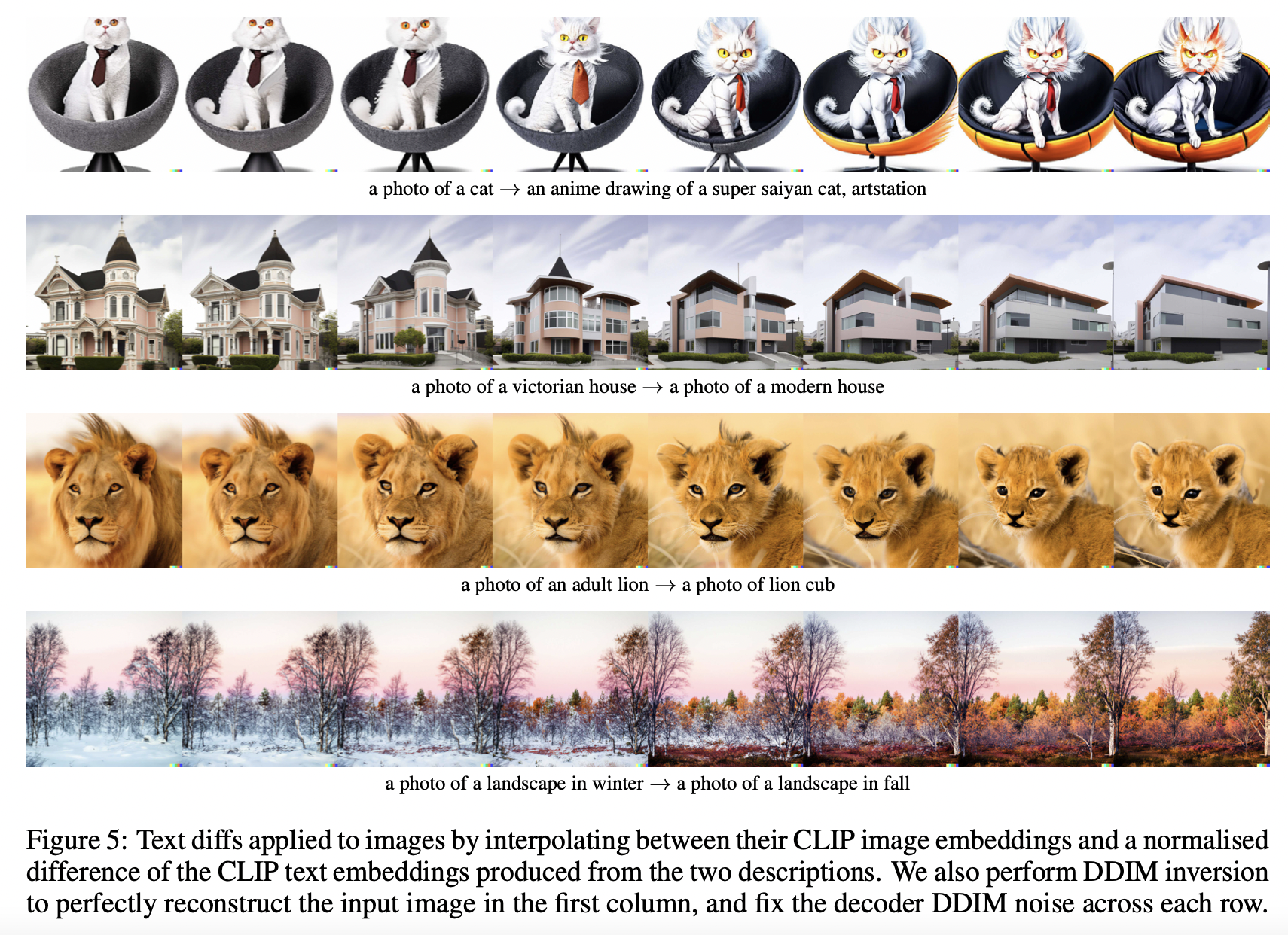
Overview of unCLIP
CLIP encoder를 다시 복원한다는 의미에서 unCLIP이라고 이름지은듯
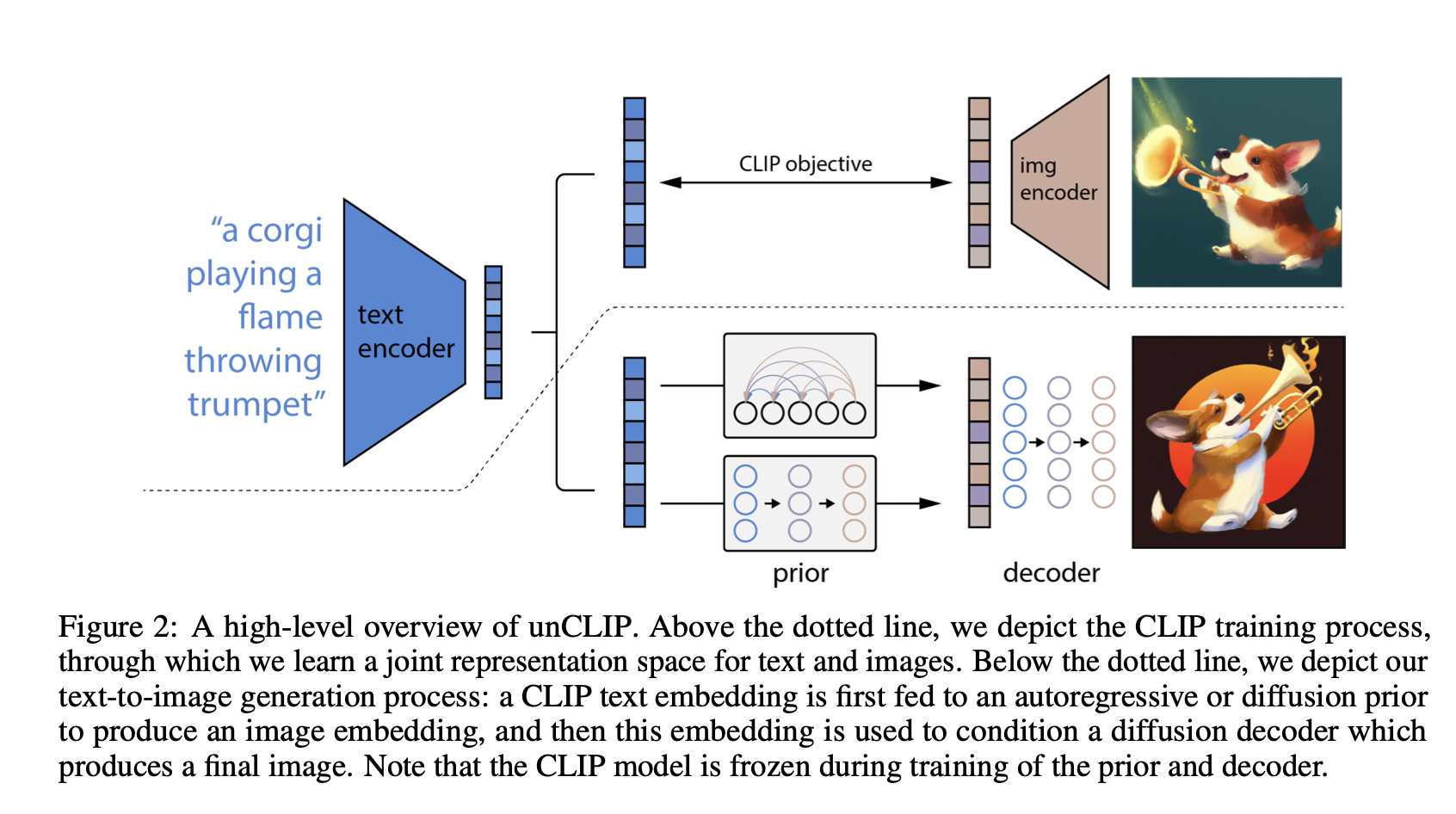
image를 x, image embedding을 zi, image captions을 y로 정의.
- Text와 Image를 받아 인코딩함으로써 학습하는 CLIP 과정이 위에 나타나있다.
- 아래에는 저자들이 제안하는 2-Stage model이 나와있다.
- stage 1: prior는 text caption y로부터 image embedding zi를 생성하는 것을 모델링함(p(zi∣y)).
- 여기서 AutoRegressvie 기반 image embedding 예측 task와 (저자들이 고안한 방법으로)Diffusion Network를 학습해 image embedding을 잘 생성해내게됨.
- stage 2: decoder는 image caption y와 prior가 건네준 image embedding zi를 기반으로 image x를 생성함(p(x∣zi,y)).
- 여기서는 Diffusion Network만을 사용함.
- 물론 CLIP은 (이미지가 없는 test 단계에서도)text caption으로부터 text embedding zt를 생성할 수 있기 때문에 이를 이미지 생성에 활용할 수도 있긴 함-자유-(p(x∣zi,y,zt).

.
- decoder는 언급된 Diffusion Network 관련 연구를 대부분 따른 것 같으나, 양질의 임베딩 생성에 도움이 되는 prior network는 생각보다 복잡하니 생략.
- 아무튼 AutoRegressive prior에서는 (compressed)image embedding token을 잘 예측하게끔 트랜스포머를 학습하며
- Diffusion prior에서는 기존의 Denoising Diffusion Probabilistic Model에 쓰인 방법(ϵ-prediction formulation) 말고, (unnosied) image embedding을 zi를 다이렉트하게, MSE loss로 예측하는 방법을 개발해 사용 !
아래는 그냥 멋지게 뽑힌 예시
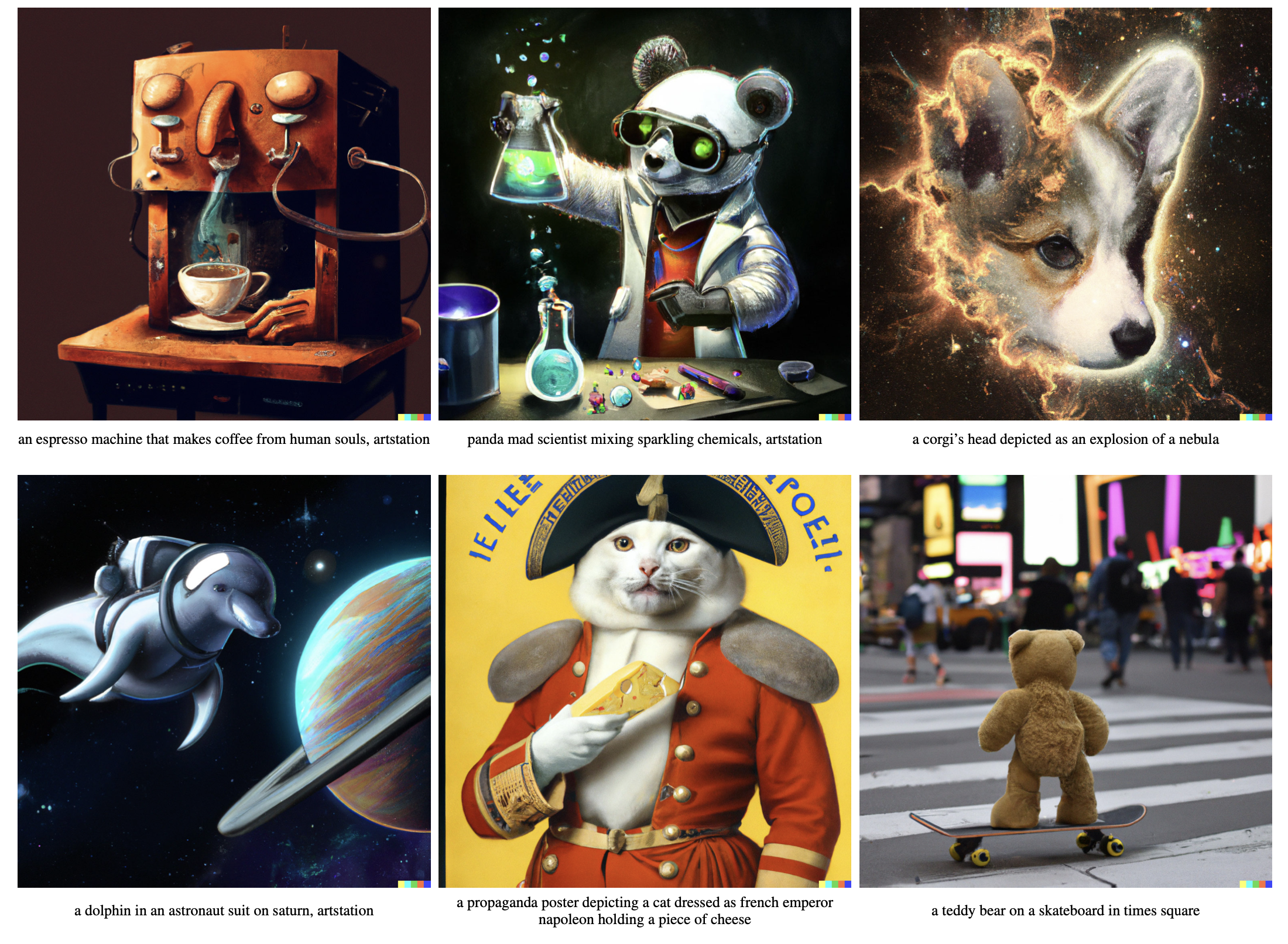
한계도 역시 존재
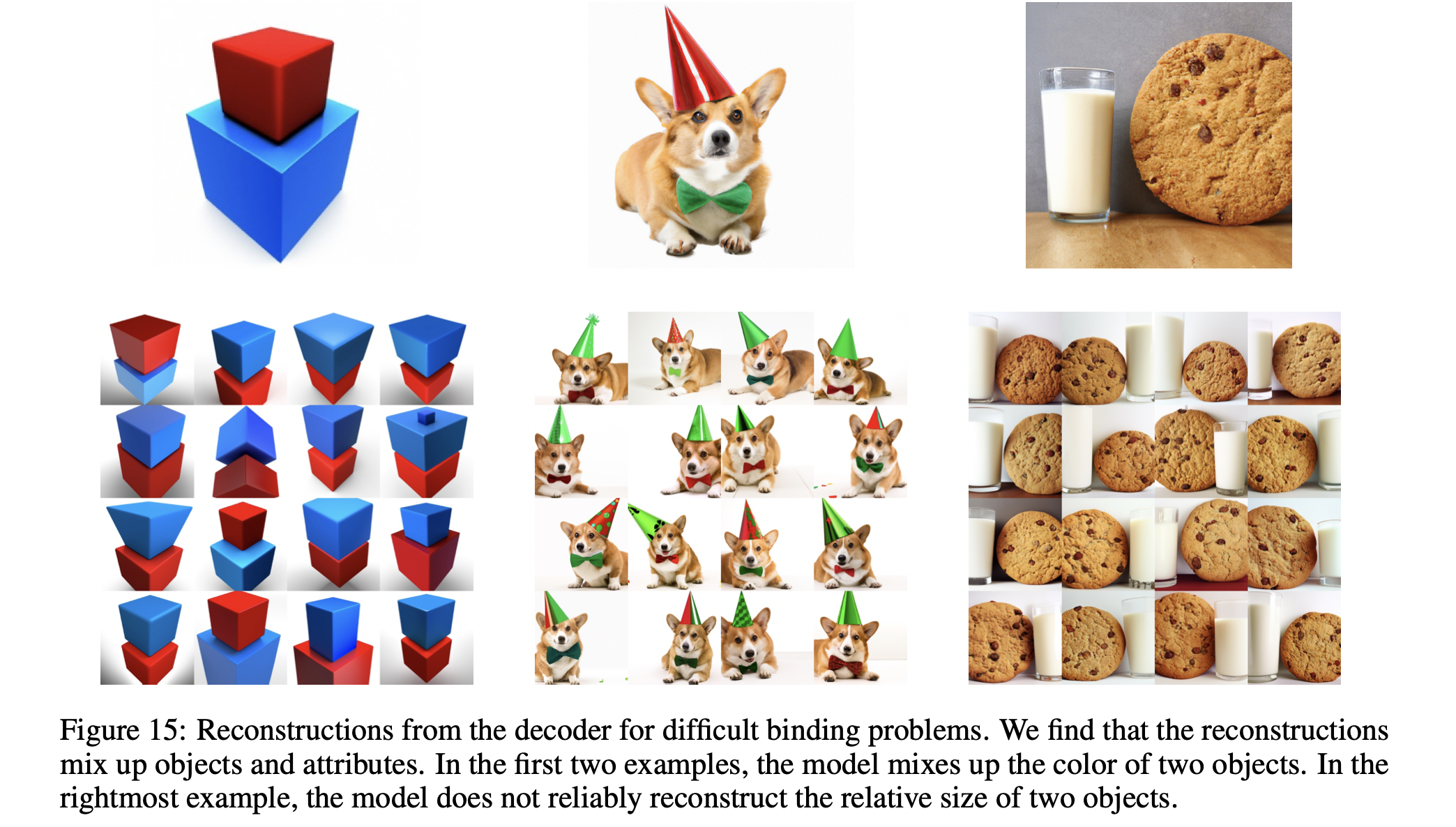
- size, color, attributes 등이 조금 섞이는 모습
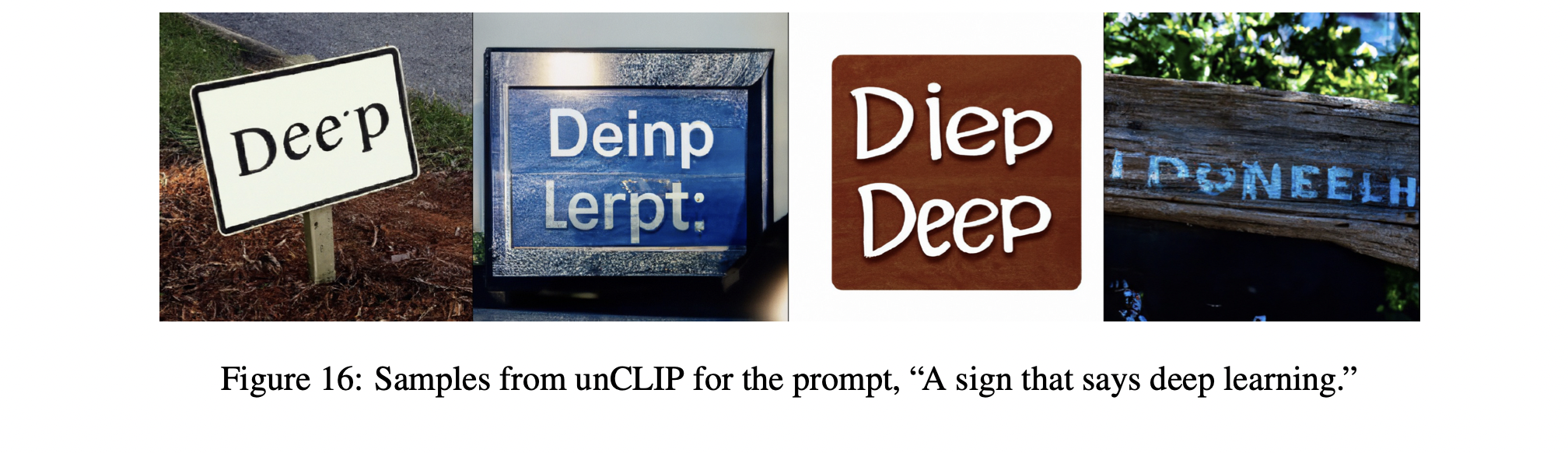
- Why don't you say "Deep Learning"...